Privacy-Preserving Deep Sequential Model with Matrix Homomorphic Encryption.
ACM Asia Conference on Computer and Communications Security (AsiaCCS)(2022)
摘要
Making deep neural networks available as a service introduces privacy problems, for which homomorphic encryption of both model and user data potentially offers the solution at the highest privacy level. However, the difficulty of operating on homomorphically encrypted data has hitherto limited the range of operations available and the depth of networks. We introduce an extended CKKS scheme MatHEAAN to provide efficient matrix representations and operations together with improved noise control. Using the MatHEAAN we developed a deep sequential model with a gated recurrent unit called MatHEGRU. We evaluated the proposed model using sequence modeling, regression, and classification of images and genome sequences. We show that the hidden states of the encrypted model, as well as the results, are consistent with a plaintext model.
更多查看译文
关键词
Homomorphic encryption, Artificial Intelligence, Recurrent Neural Network, Deep Learning
AI 理解论文
溯源树
样例
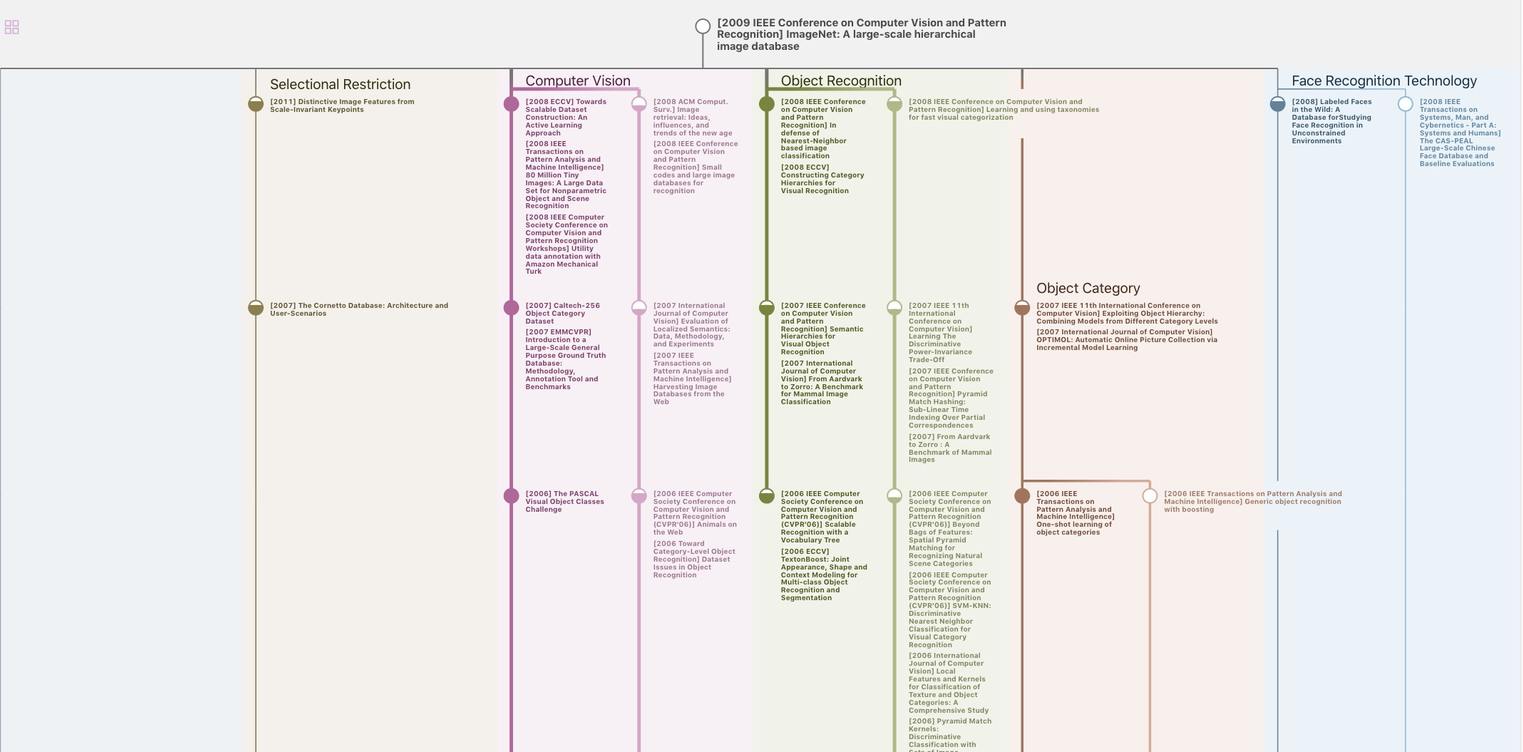
生成溯源树,研究论文发展脉络
Chat Paper
正在生成论文摘要