SemOI2 - Building Adaptive and Cost-Effective Recognition Applications With Semantic Augmentation.
AAAI Spring Symposia(2022)
Abstract
Neural nets are the backbone for many innovative applications when it comes to making sense out of unstructured data. This technology, however, also has some significant downsides. Training these neural nets requires vast amounts of annotated training data and computational power and is, thus, expensive. Adding, altering, or removing concepts all trigger costly retraining of the neural net. Semantic technologies can curb these systemic disadvantages. They can be connected to the neural net and augment the detection. The ontology can be easily adapted to new situations and use cases without requiring much computational power. This paper presents a prototype application for connecting the general-purpose OpenImages detection with a semantic. Freeto-use training datasets provide a cost-efficient way to use neural nets – often, there exist even pre-trained models for the large machine learning frameworks. The semantic contextualizes the low-level knowledge and enables the realization of specific use cases. We further propose a methodology to transfer the probability of the image detection and the prominence of items in the picture to the ground truth of the ontology by introducing a new measurement Semantic Confidence (SC).
MoreTranslated text
AI Read Science
Must-Reading Tree
Example
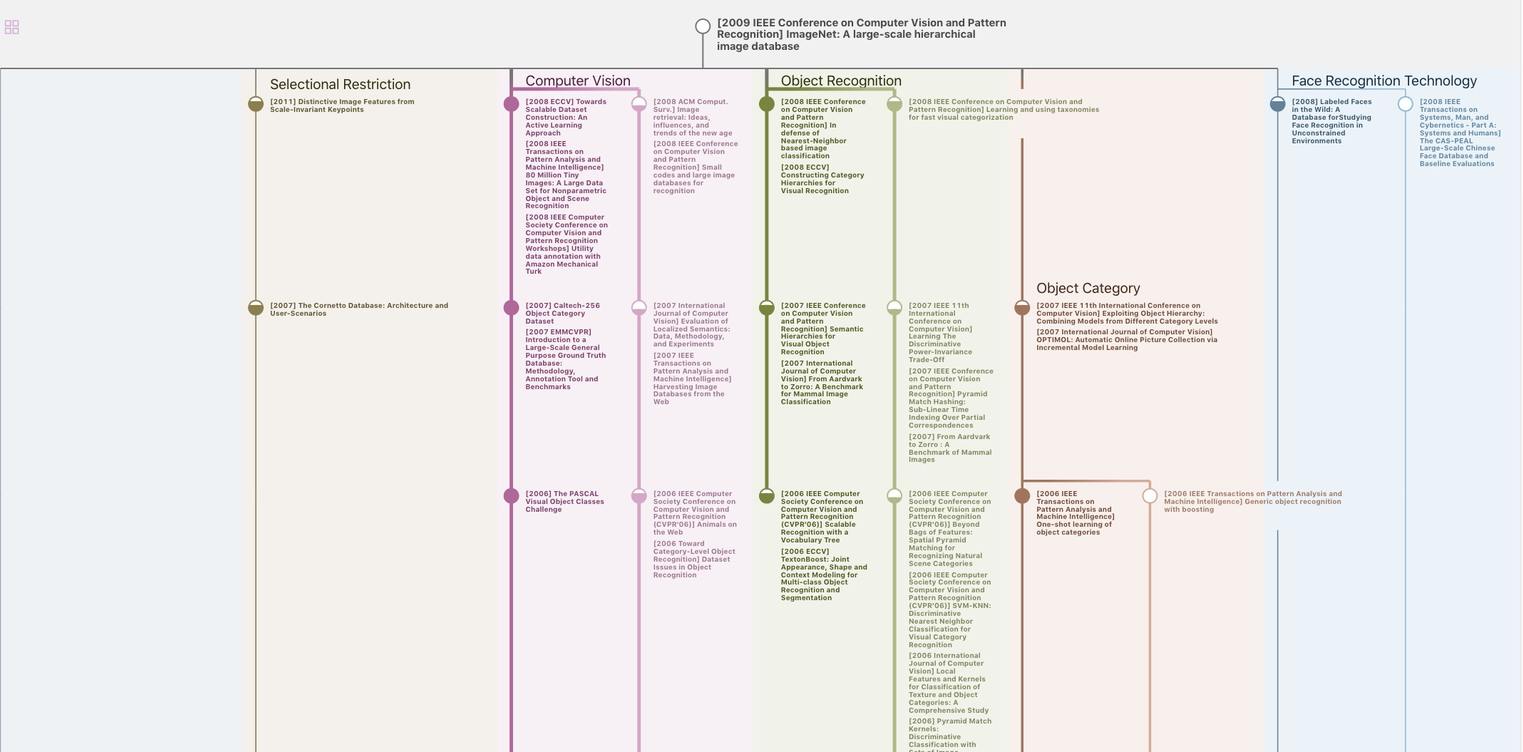
Generate MRT to find the research sequence of this paper
Chat Paper
Summary is being generated by the instructions you defined