Differentiating Student Feedbacks for Knowledge Tracing
CoRR(2022)
摘要
In computer-aided education and intelligent tutoring systems, knowledge tracing (KT) raises attention due to the development of data-driven learning methods, which aims to predict students' future performance given their past question response sequences to trace their knowledge states. However, current deep learning approaches only focus on enhancing prediction accuracy, but neglecting the discrimination imbalance of responses. That is, a considerable proportion of question responses are weak to discriminate students' knowledge states, but equally considered compared to other discriminative responses, thus hurting the ability of tracing students' personalized knowledge states. To tackle this issue, we propose DR4KT for Knowledge Tracing, which reweights the contribution of different responses according to their discrimination in training. For retaining high prediction accuracy on low discriminative responses after reweighting, DR4KT also introduces a discrimination-aware score fusion technique to make a proper combination between student knowledge mastery and the questions themselves. Comprehensive experimental results show that our DR4KT applied on four mainstream KT methods significantly improves their performance on three widely-used datasets.
更多查看译文
AI 理解论文
溯源树
样例
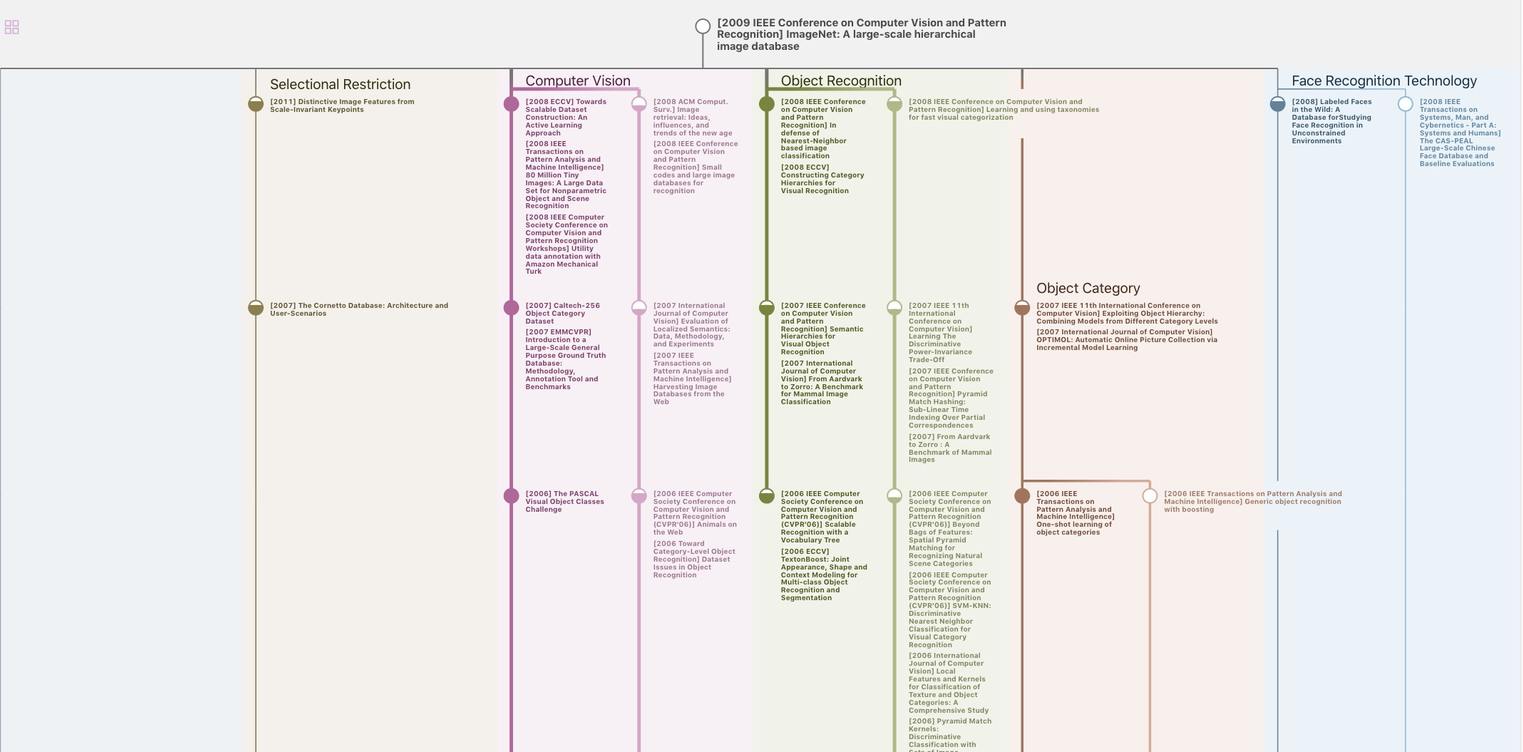
生成溯源树,研究论文发展脉络
Chat Paper
正在生成论文摘要