Policy Optimization to Learn Adaptive Motion Primitives in Path Planning With Dynamic Obstacles
IEEE Robotics and Automation Letters(2023)
摘要
This letter addresses the kinodynamic motion planning for non-holonomic robots in dynamic environments with both static and dynamic obstacles – a challenging problem that lacks a universal solution yet. One of the promising approaches to solve it is decomposing the problem into the smaller sub-problems and combining the local solutions into the global one. The crux of any planning method for non-holonomic robots is the generation of motion primitives that generates solutions to local planning sub-problems. In this work we introduce a novel learnable steering function (policy), which takes into account kinodynamic constraints of the robot and both static and dynamic obstacles. This policy is efficiently trained via the policy optimization. Empirically, we show that our steering function generalizes well to unseen problems. We then plug in the trained policy into the sampling-based and lattice-based planners, and evaluate the resultant POLAMP algorithm (Policy Optimization that Learns Adaptive Motion Primitives) in a range of challenging setups that involve a car-like robot operating in the obstacle-rich parking-lot environments. We show that POLAMP is able to plan collision-free kinodynamic trajectories with success rates higher than 92%, when 50 simultaneously moving obstacles populate the environment showing better performance than the state-of-the-art competitors.
更多查看译文
关键词
Motion and path planning,task and motion planning,reinforcement learning
AI 理解论文
溯源树
样例
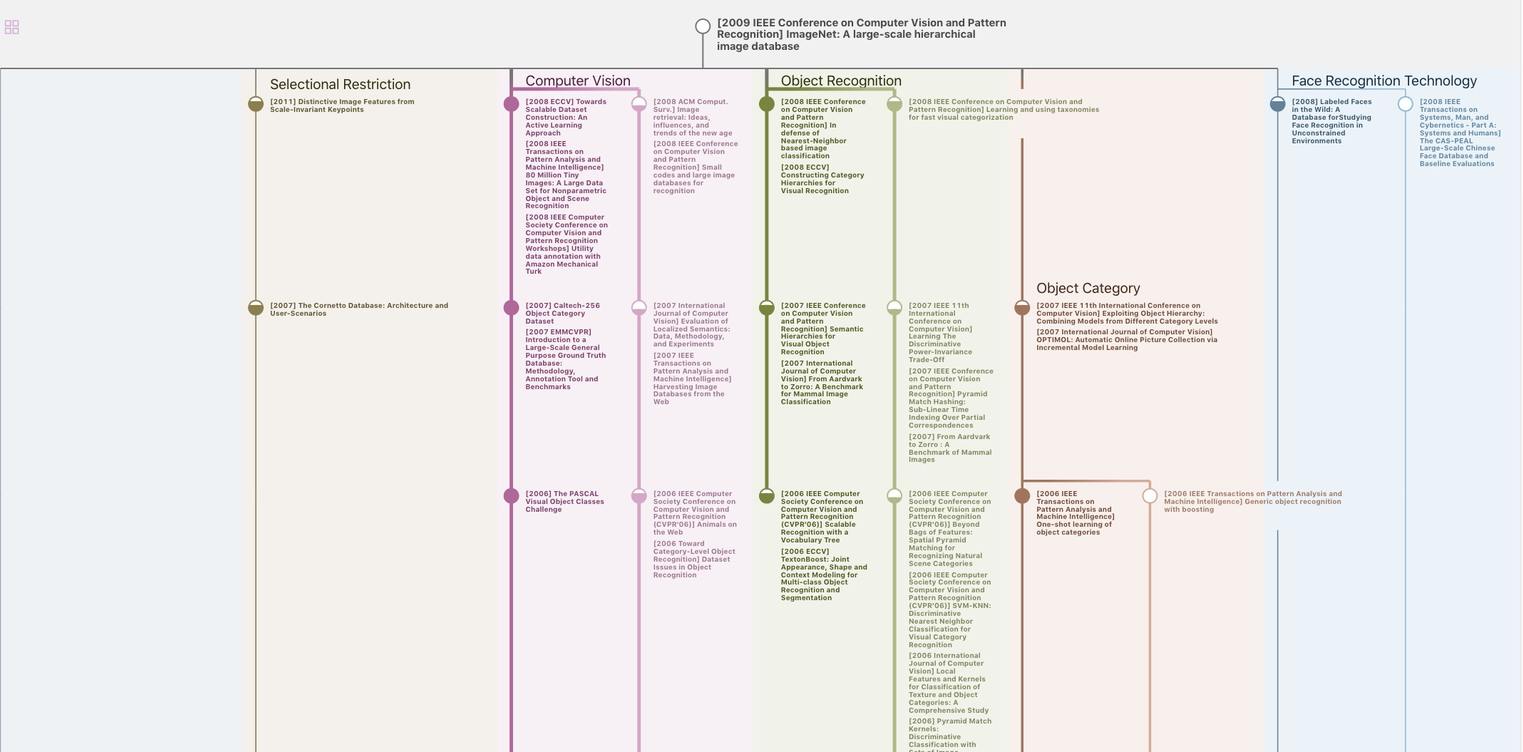
生成溯源树,研究论文发展脉络
Chat Paper
正在生成论文摘要