Feature Grouping and Selection With Graph Theory in Robust Fuzzy Rough Approximation Space
IEEE Transactions on Fuzzy Systems(2023)
摘要
Most extant feature selection works neglect interactive features in the form of groups, leading to the omission of some important discriminative information. Moreover, the prevalence of data with uncertainty, fuzziness, and noise poses a certain obstacle to feature selection. Driven by these two issues, a Feature Grouping and Selection approach in Robust Fuzzy Rough Approximation Space using graph theory (FGS-RFRAS) is proposed in this study. First, a robust fuzzy rough approximation space is constructed by a neighborhood adaptive
$\beta$
-precision fuzzy rough set model to enhance the robustness and antinoise ability of the fuzzy rough set model. Second, uncertainty measures in robust fuzzy rough approximation space are defined to analyze the interactivity and redundancy of pairwise features on graph structure. Then, a strategy of
Interactive Retainment, Weakly Correlated Removal, and Max-Dependent Selection
is devised to guide feature grouping and selection. Experiments are performed on 21 datasets to evaluate the performance of FGS-RFRAS and demonstrate its significance. The robustness test indicates that it is antinoise for mislabeling.
更多查看译文
关键词
Feature grouping,feature interaction,feature selection,fuzzy rough set (FRS),graph theory,robust,uncertainty measures
AI 理解论文
溯源树
样例
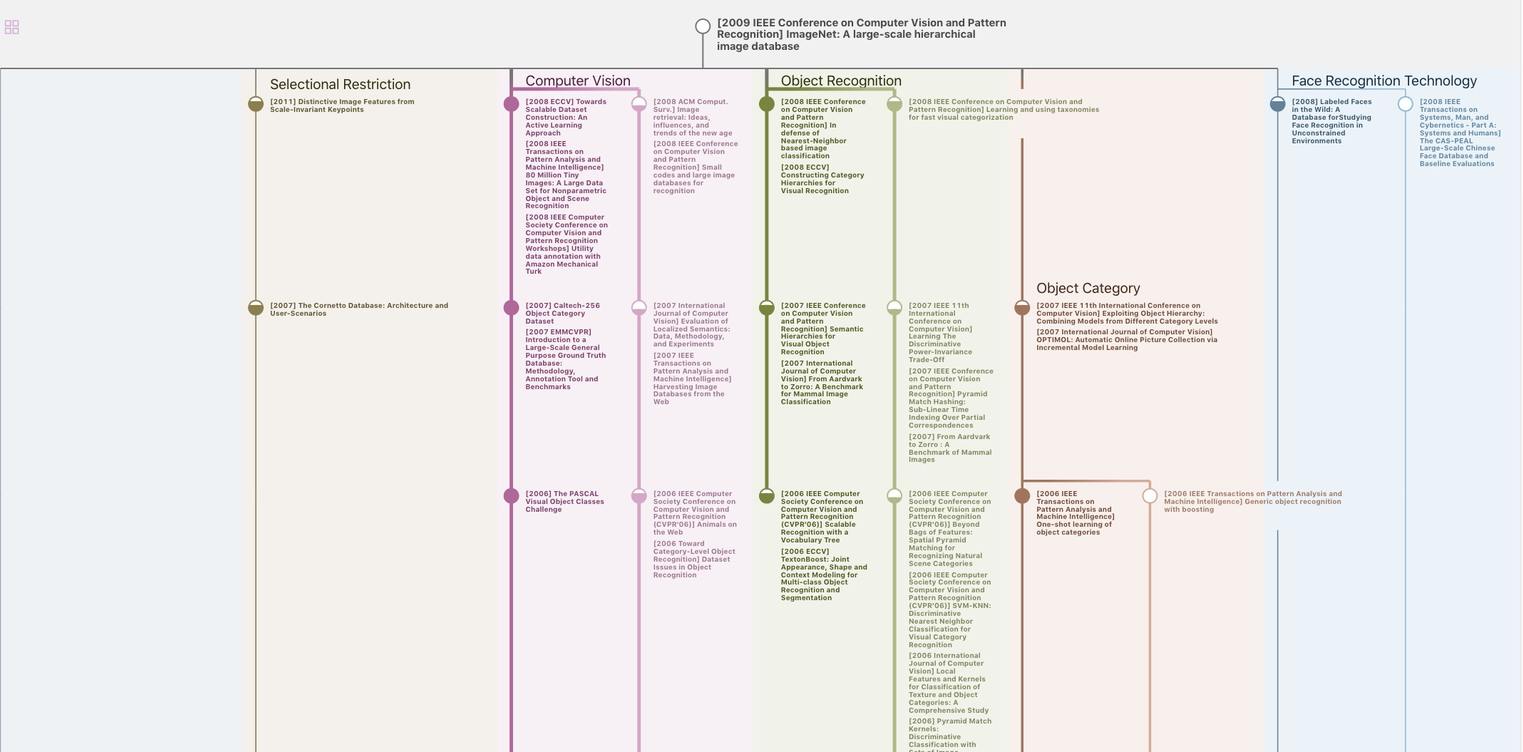
生成溯源树,研究论文发展脉络
Chat Paper
正在生成论文摘要