Language Model Agnostic Gray-Box Adversarial Attack on Image Captioning
IEEE Transactions on Information Forensics and Security(2023)
摘要
Adversarial susceptibility of neural image captioning is still under-explored due to the complex multi-model nature of the task. We introduce a GAN-based adversarial attack to effectively fool encoder-decoder based image captioning frameworks. Unique to our attack is the systematic disruption of the internal representation of an image at the encoder stage which allows control over the captions generated at the decoder stage. We cause the desired disruption with an input perturbation that promotes similarity between the features of the input image with a target image of our choice. The target image provides a convenient handle to control the incorrect captions in our method. We do not assume any knowledge of the decoder module, which makes our attack ‘gray-box’. Moreover, our attack remains agnostic to the type of decoder module, thereby proving effective for RNNs as well as Transformers as the language models. This makes our attack highly pragmatic.
更多查看译文
关键词
Adversarial attack,image captioning,recurrent networks,transformers,language models,generative adversarial networks (GANs)
AI 理解论文
溯源树
样例
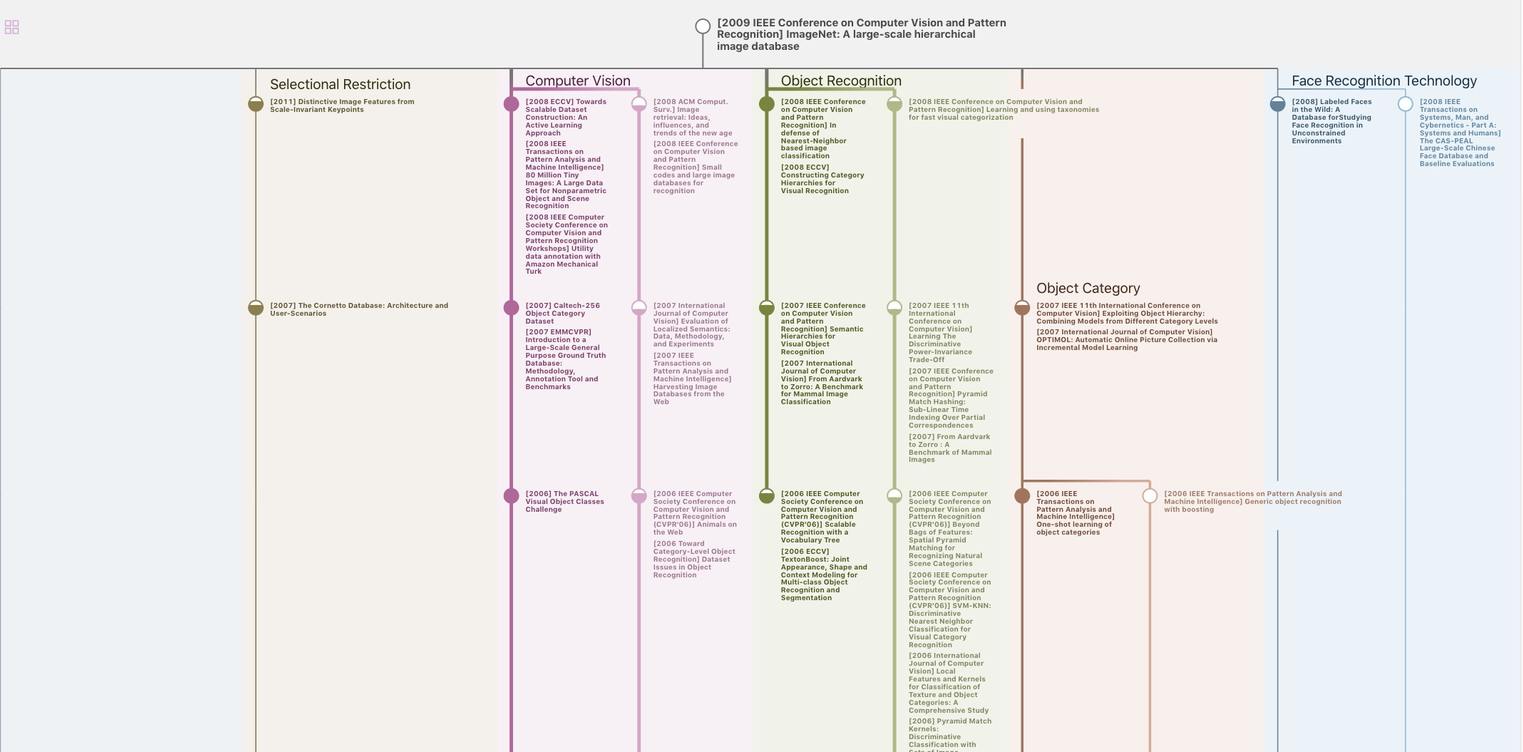
生成溯源树,研究论文发展脉络
Chat Paper
正在生成论文摘要