Neighbor Graph Based Tensor Recovery For Accurate Internet Anomaly Detection
IEEE Transactions on Parallel and Distributed Systems(2023)
摘要
Detecting anomalous traffic is a crucial task for network management. Although many anomaly detection algorithms have been proposed recently, constrained by their matrix-based traffic data model, existing algorithms often suffer from low detection accuracy. To fully utilize the multi-dimensional information hidden in the traffic data, this paper uses the tensor model for more accurate Internet anomaly detection. Only considering the low-rank linearity features hidden in the data, current tensor factorization techniques would result in low anomaly detection accuracy. We propose a novel Graph-based Tensor Recovery model (Graph-TR) to well explore both low-rank linearity features as well as the non-linear proximity information hidden in the traffic data for better anomaly detection. We encode the non-linear proximity information of the traffic data by constructing nearest neighbor graphs and incorporate this information into the tensor factorization using the graph Laplacian. Moreover, to facilitate the quick building of neighbor graph, we propose a nearest neighbor searching algorithm with the simple locality-sensitive hashing (LSH). Besides only detecting random anomalies, our algorithm can also effectively detect structured anomalies that appear as bursts. We have conducted extensive experiments using Internet traffic trace data Abilene and GEANT. Compared with the state of art algorithms on matrix-based anomaly detection and tensor recovery approach, our Graph-TR can achieve higher Accuracy and Recall.
更多查看译文
关键词
Traffic anomaly detection,tensor recovery,neighbor graph
AI 理解论文
溯源树
样例
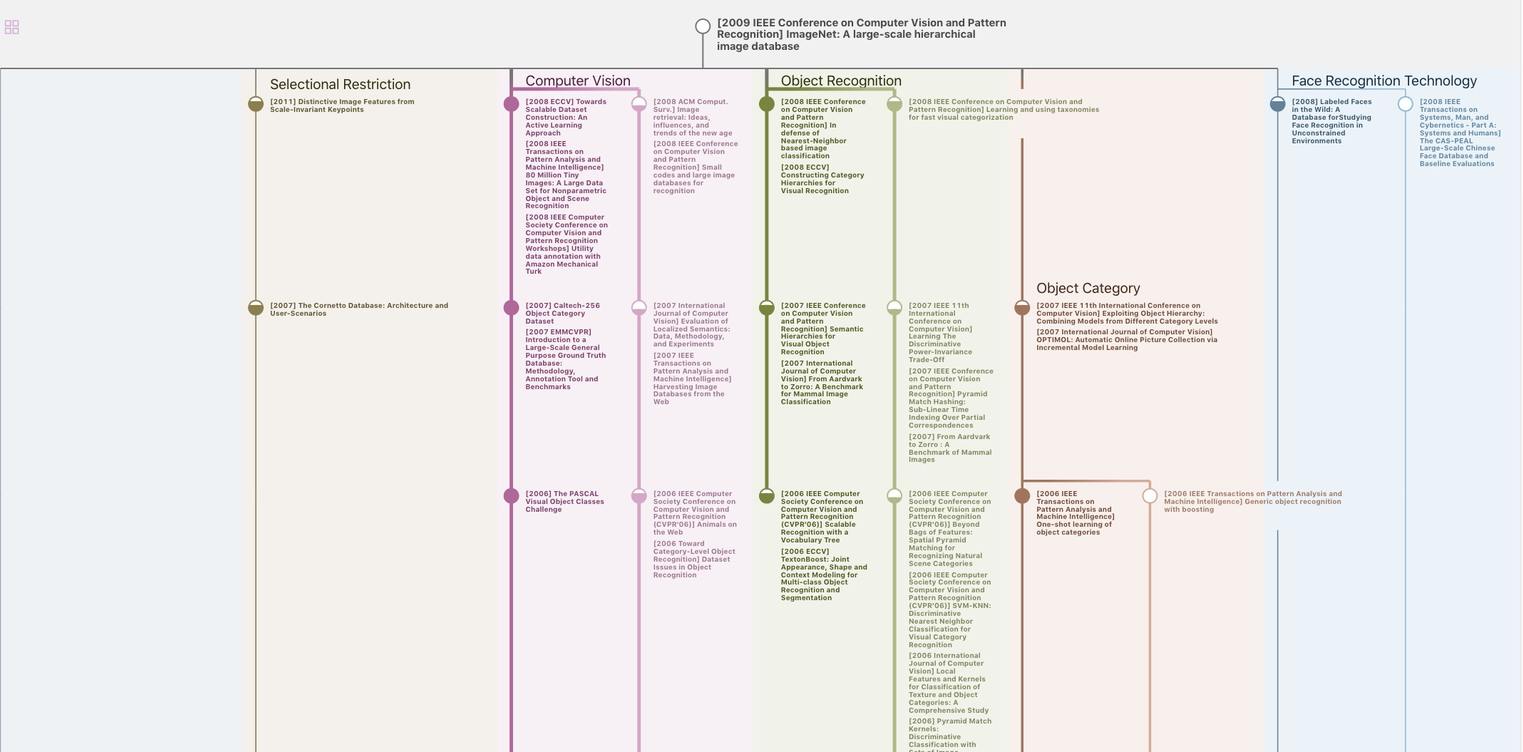
生成溯源树,研究论文发展脉络
Chat Paper
正在生成论文摘要