Multistep Ahead Prediction of Vehicle Lateral Dynamics Based on Echo State Model
IEEE Sensors Journal(2023)
摘要
Lateral dynamics are critical for high-level autonomous driving, and multistep forecasting can significantly improve vehicle safety. However, the dynamics prediction method is rarely announced due to the difficulty of accurate prediction. In contrast to conventional time-series prediction, this article proposes a novel method for predicting a dynamical system with implicit parameters. Specifically, we construct an echo state model (ESM) incorporating a rational data organization and an efficient neural network structure. We observe the fading information property (FIP) of dynamic vehicle parameters and use temporal data to construct equivalent states for implicit parameters. Finally, a gated recurrent unit (GRU) neural network is designed and trained to accomplish the complex mapping of a vehicle dynamical system. Its performance is compared to that of several benchmark networks. The proposed method is capable of performing four-step-ahead prediction with high accuracy in both dry and slippery road conditions, implying the increased potential for some safe and comfortable driving applications of autonomous vehicles.
更多查看译文
关键词
Echo state model (ESM),fading information property (FIP),implicit parameters learning,multistep ahead prediction
AI 理解论文
溯源树
样例
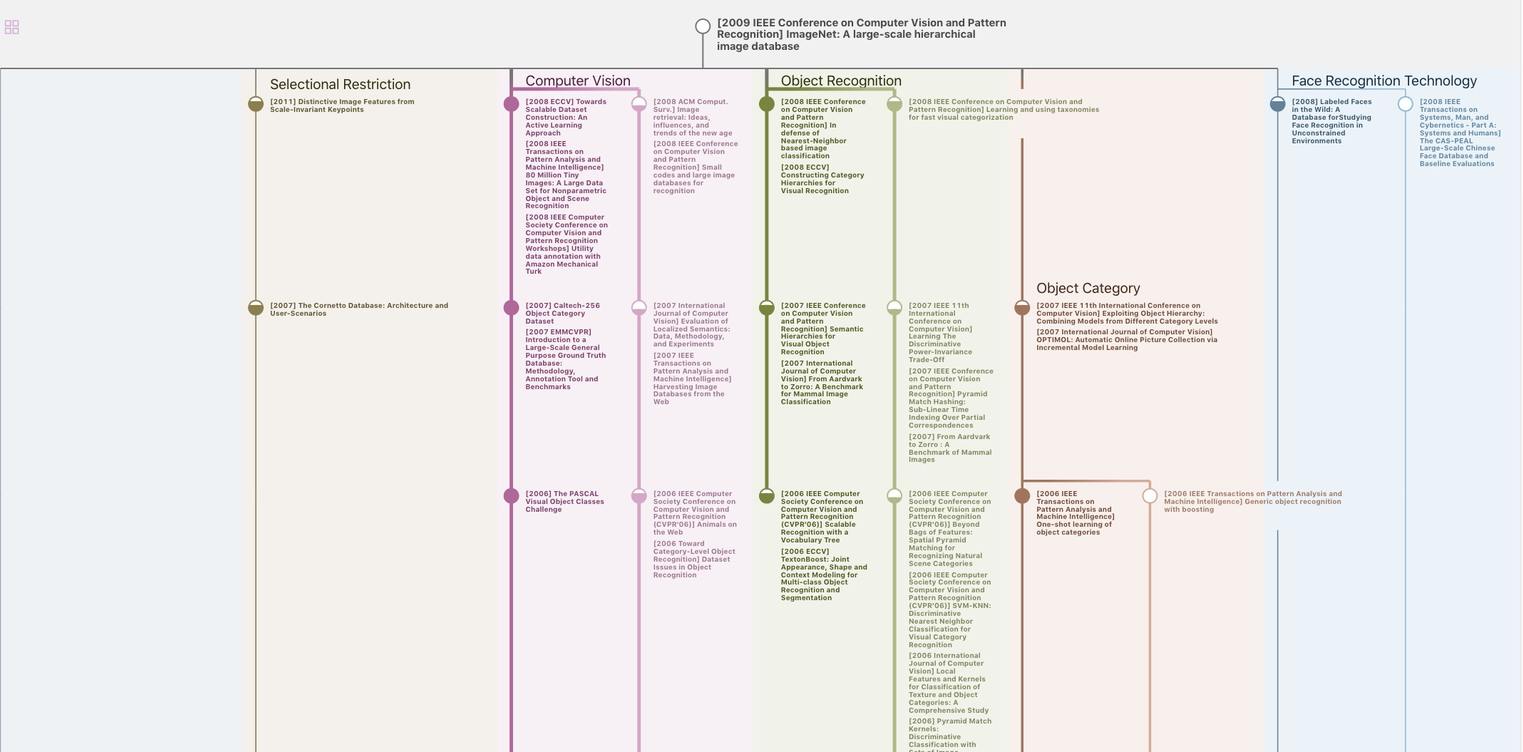
生成溯源树,研究论文发展脉络
Chat Paper
正在生成论文摘要