A Deep-Learning-Based Fast Counting Methodology Using Density Estimation for Counting Shrimp Larvae
IEEE Sensors Journal(2023)
摘要
In the process of white shrimp farming, shrimp larvae trading is a time-consuming and laborious process. In the traditional shrimp larvae counting process, it is often necessary to count the number of shrimp larvae for sale through manual counting. However, there is not unified standard method for counting. Computer counting methods can only identify a small number of nonoverlapping shrimp larvae, and the accuracy of the methods is not high. In recent years, with the advent of deep learning, there have been many applications of counting objects. It is desirable to develop a method for counting shrimp larvae that is less labor-intensive and can achieve fast results. In this article, a deep-learning-based fast counting methodology using density estimation for counting shrimp larvae is proposed, with noteworthy advantages over traditional shrimp larvae counting methods. The experimental results showed that the average accuracy of the proposed methodology was as high as 98.72% when the number of shrimps was approximately 1000. Moreover, the average execution time of the proposed methodology was around 12.35 s so that the average execution time can be accepted in the real world.
更多查看译文
关键词
Aquaculture,computer vision,deep learning,image recognition,image sensor application,intelligent systems,shrimp larvae counting
AI 理解论文
溯源树
样例
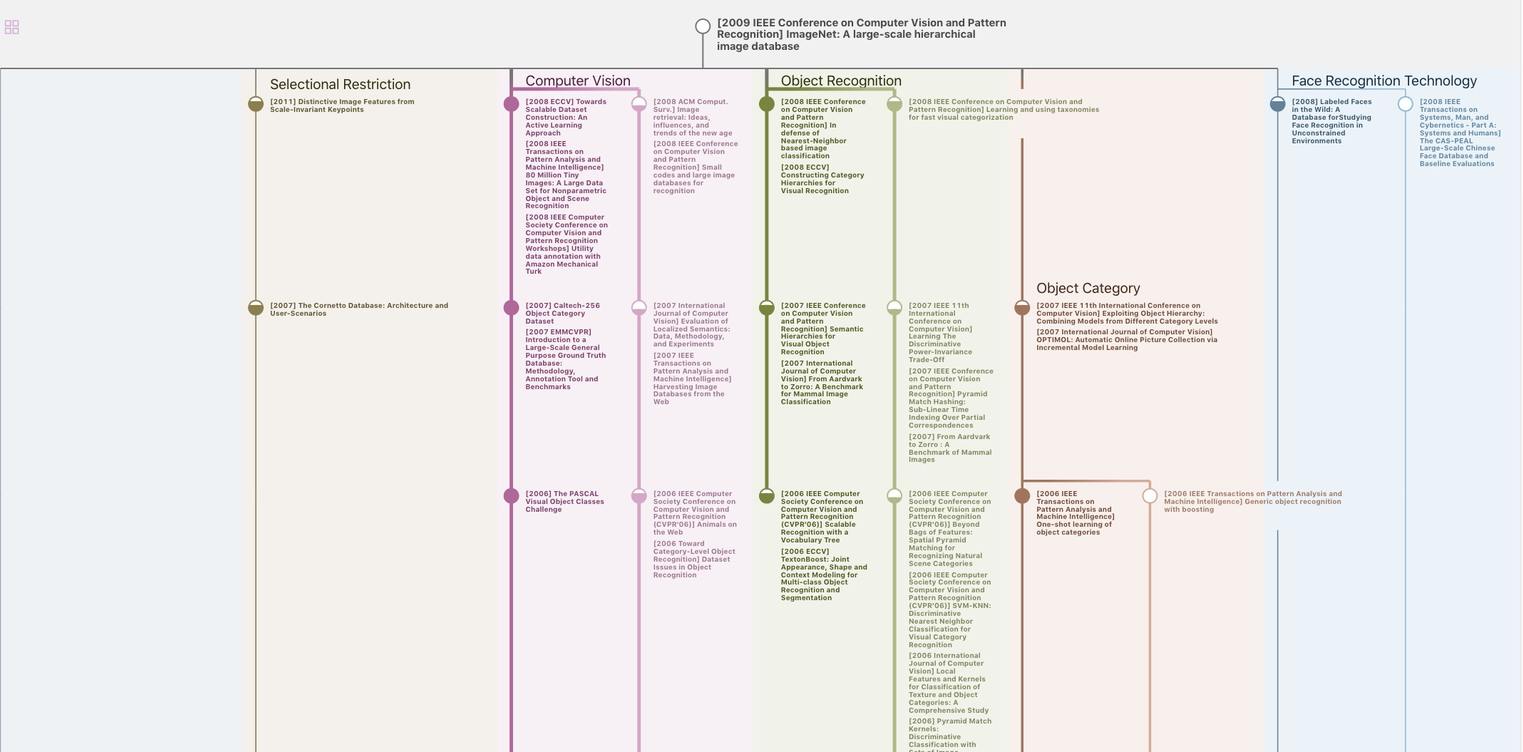
生成溯源树,研究论文发展脉络
Chat Paper
正在生成论文摘要