An Energy-Efficient Transformer Processor Exploiting Dynamic Weak Relevances in Global Attention
IEEE Journal of Solid-State Circuits(2023)
摘要
Transformer-based models achieve tremendous success in many artificial intelligence (AI) tasks, outperforming conventional convolution neural networks (CNNs) from natural language processing (NLP) to computer vision (CV). Their success relies on the self-attention mechanism that provides a global rather than local receptive field as CNNs. Despite its superiority, the global–level self-attention consumes
$\sim 100\times $
more operations than CNNs and cannot be effectively handled by the existing CNN processor due to the distinct operations. It inspires an urgent requirement to design a dedicated Transformer processor. However, global self-attention involves massive naturally existent weakly related tokens (WR-Tokens) due to the redundant contents in human languages or images. These WR-Tokens generate zero and near-zero attention results that introduce energy consumption bottleneck, redundant computations, and hardware under-utilization issues, making it challenging to achieve energy-efficient self-attention computing. This article proposes a Transformer processor effectively handling the WR-Tokens to solve these challenges. First, a big-exact-small-approximate processing element (PE) reduces multiply-and-accumulate (MAC) energy for WR-Tokens by adaptively computing the small values approximately while computing the large values exactly. Second, a bidirectional asymptotical speculation unit captures and removes redundant computations of zero attention outputs by exploiting the local property of self-attention. Third, an out-of-order PE-line computing scheduler improves hardware utilization for near-zero values by reordering the operands to dovetail two operations into one multiplication. Fabricated in a 28-nm CMOS technology, the proposed processor occupies an area of 6.82 mm2. When evaluated with a 90% of approximate computing for the generative pre-trained transformer 2 (GPT-2) model, the peak energy efficiency is 27.56 TOPS/W under 0.56 V at 50 MHz,
$17.66\times $
higher than A100 graphics processing unit (GPU). Compared with the state-of-the-art Transformer processor, it reduces energy by
$4.57\times $
and offers
$3.73\times $
speedup.
更多查看译文
关键词
Approximate computing,out-of-order computing,processor,self-attention,speculating,Transformer
AI 理解论文
溯源树
样例
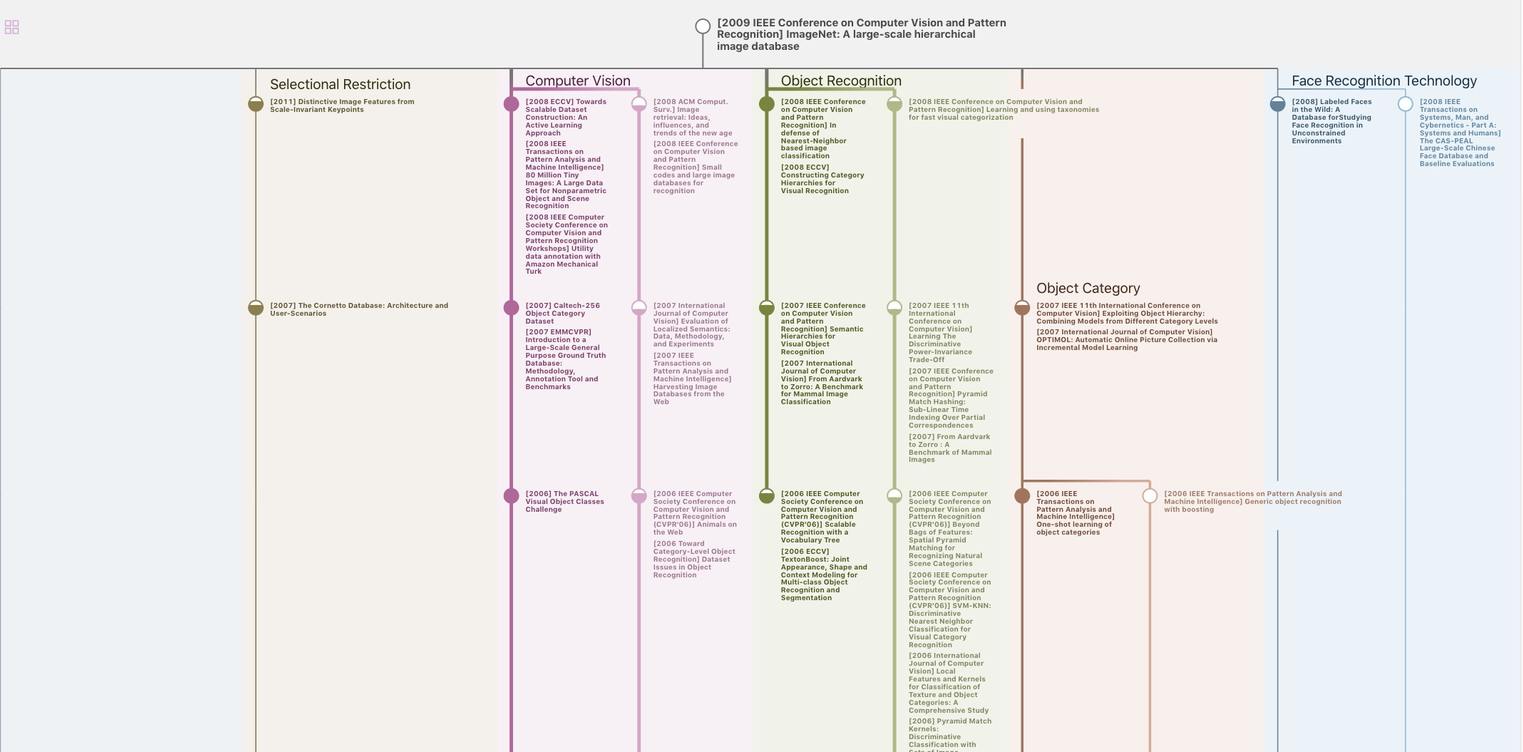
生成溯源树,研究论文发展脉络
Chat Paper
正在生成论文摘要