HeartBEiT: Vision Transformer for Electrocardiogram Data Improves Diagnostic Performance at Low Sample Sizes
arxiv(2022)
摘要
The electrocardiogram (ECG) is a ubiquitous diagnostic modality. Convolutional neural networks (CNNs) applied towards ECG analysis require large sample sizes, and transfer learning approaches result in suboptimal performance when pre-training is done on natural images. We leveraged masked image modeling to create the first vision-based transformer model, HeartBEiT, for electrocardiogram waveform analysis. We pre-trained this model on 8.5 million ECGs and then compared performance vs. standard CNN architectures for diagnosis of hypertrophic cardiomyopathy, low left ventricular ejection fraction and ST elevation myocardial infarction using differing training sample sizes and independent validation datasets. We show that HeartBEiT has significantly higher performance at lower sample sizes compared to other models. Finally, we also show that HeartBEiT improves explainability of diagnosis by highlighting biologically relevant regions of the EKG vs. standard CNNs. Thus, we present the first vision-based waveform transformer that can be used to develop specialized models for ECG analysis especially at low sample sizes.
更多查看译文
关键词
electrocardiogram data,vision transformer,diagnostic performance
AI 理解论文
溯源树
样例
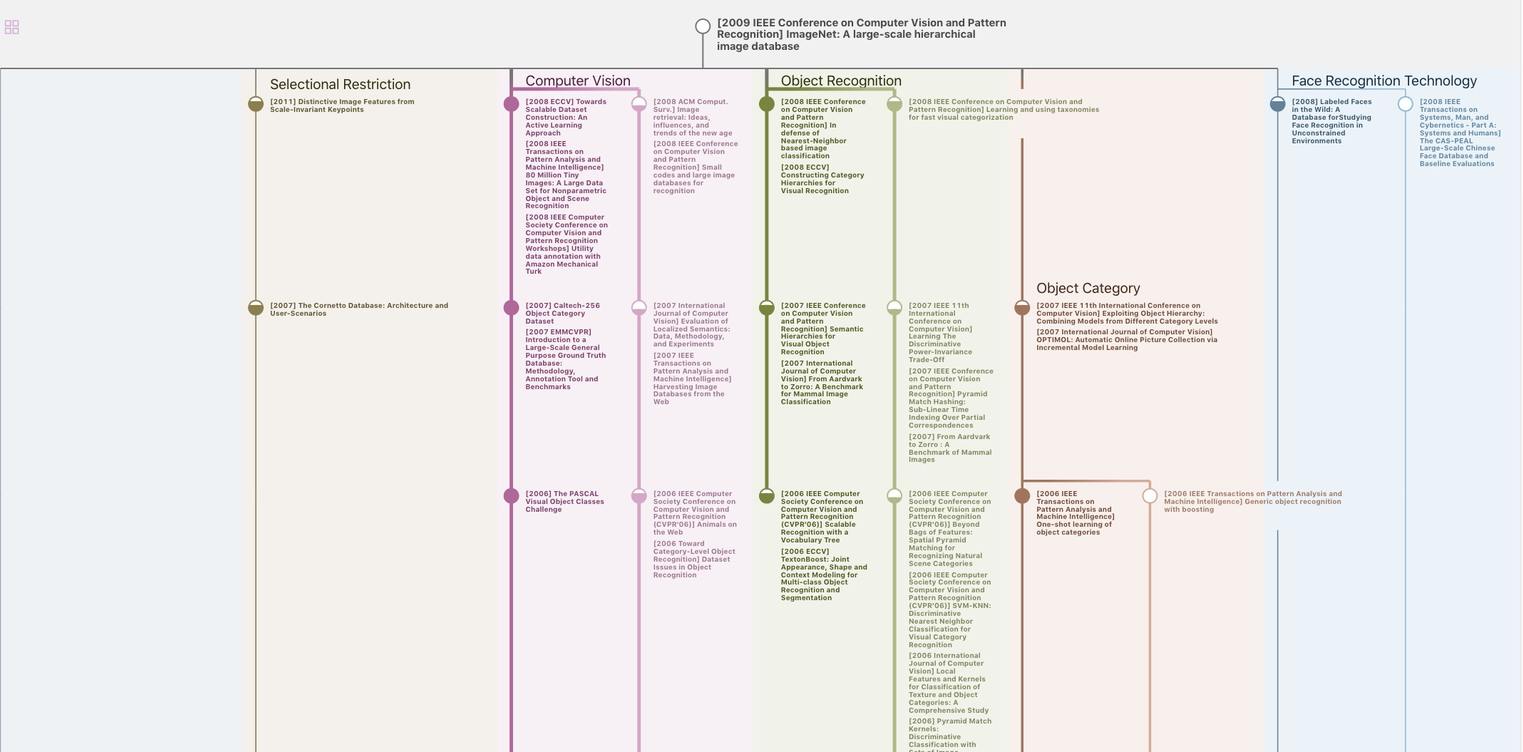
生成溯源树,研究论文发展脉络
Chat Paper
正在生成论文摘要