Influence of language on perception and concept formation in a brain-constrained deep neural network model.
Philosophical transactions of the Royal Society of London. Series B, Biological sciences(2023)
摘要
A neurobiologically constrained model of semantic learning in the human brain was used to simulate the acquisition of concrete and abstract concepts, either with or without verbal labels. Concept acquisition and semantic learning were simulated using Hebbian learning mechanisms. We measured the network's category learning performance, defined as the extent to which it successfully (i) grouped partly overlapping perceptual instances into a single (abstract or concrete) conceptual representation, while (ii) still distinguishing representations for distinct concepts. Co-presence of linguistic labels with perceptual instances of a given concept generally improved the network's learning of categories, with a significantly larger beneficial effect for abstract than concrete concepts. These results offer a neurobiological explanation for causal effects of language structure on concept formation and on perceptuo-motor processing of instances of these concepts: supplying a verbal label during concept acquisition improves the cortical mechanisms by which experiences with objects and actions along with the learning of words lead to the formation of neuronal ensembles for specific concepts and meanings. Furthermore, the present results make a novel prediction, namely, that such 'Whorfian' effects should be modulated by the concreteness/abstractness of the semantic categories being acquired, with language labels supporting the learning of abstract concepts more than that of concrete ones. This article is part of the theme issue 'Concepts in interaction: social engagement and inner experiences'.
更多查看译文
关键词
Hebbian learning,cognition,concepts,deep neural networks,linguistic relativity,neurocomputational modelling
AI 理解论文
溯源树
样例
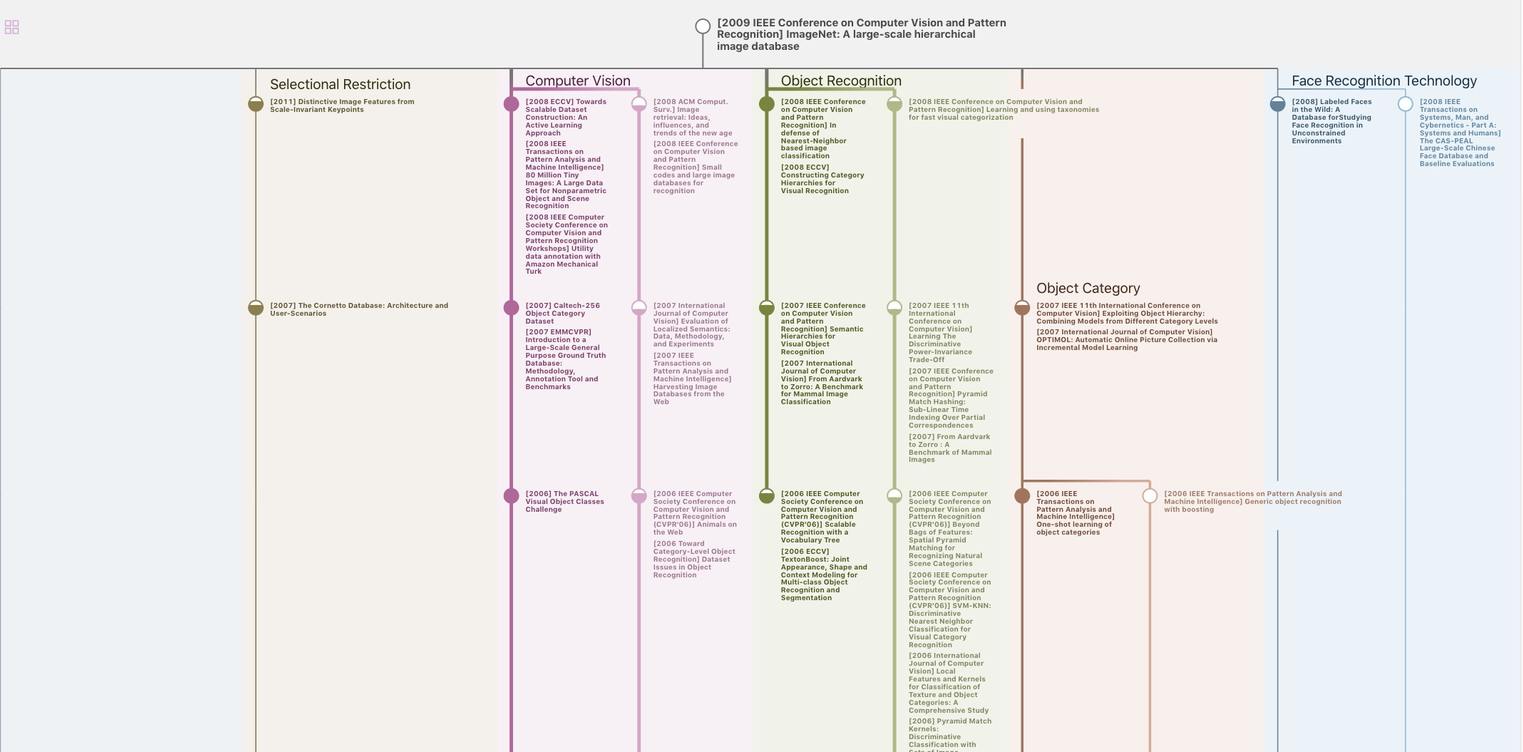
生成溯源树,研究论文发展脉络
Chat Paper
正在生成论文摘要