Revisiting the Application of an Adult Kidney Failure Risk Prediction Equation to Children With CKD.
American journal of kidney diseases : the official journal of the National Kidney Foundation(2022)
Abstract
The Kidney Failure Risk Equation (KFRE), developed in a geriatric cohort, is a widely validated equation to predict kidney failure for adults with mild-to-moderate chronic kidney disease (CKD).1Tangri N. Stevens L.A. Griffith J. et al.A predictive model for progression of chronic kidney disease to kidney failure.JAMA. 2011; 305: 1553-1559https://doi.org/10.1001/jama.2011.451Crossref PubMed Scopus (751) Google Scholar When applied to the Chronic Kidney Disease in Children (CKiD) cohort, the KFRE yielded good discrimination2Winnicki E. McCulloch C.E. Mitsnefes M.M. Furth S.L. Warady B.A. Ku E. Use of the kidney failure risk equation to determine the risk of progression to end-stage renal disease in children with chronic kidney disease.JAMA Pediatr. 2018; 172: 174-180https://doi.org/10.1001/jamapediatrics.2017.4083Crossref PubMed Scopus (36) Google Scholar and was recommended for pediatric practice,3Dart A. Komenda P. Tangri N. Time to implement the kidney failure risk equation into pediatric practice.JAMA Pediatr. 2018; 172: 122-123https://doi.org/10.1001/jamapediatrics.2017.4092Crossref PubMed Scopus (1) Google Scholar despite heterogeneous CKD etiologies and progression in children. However, this analysis only presented limited assessment of calibration—the other metric, along with discrimination, necessary for instrument validation—and this deserves particular attention in the application of an adult instrument for children. While discrimination summarizes a prognostic test’s ability to ascribe a higher risk score to those with versus those without the event, calibration refers to the congruency between predicted and observed risk. Calibration performance is often under-reported,4Wessler B.S. Lana Lai Y. Kramer W. et al.Clinical prediction models for cardiovascular disease: the Tufts PACE CPM Database.Circ Cardiovasc Qual Outcomes. 2015; 8: 368-375https://doi.org/10.1161/CIRCOUTCOMES.115.001693Crossref PubMed Scopus (64) Google Scholar and poorly calibrated instruments, even with good discrimination, can misinform and compromise decision making.5Van Calster B. McLernon D.J. van Smeden M. et al.Calibration: the Achilles heel of predictive analytics.BMC Med. 2019; 17: 230https://doi.org/10.1186/s12916-019-1466-7Crossref PubMed Scopus (438) Google Scholar To quantify calibration of the KFRE in children with CKD, we used a formal statistical test (Greenwood Nam-D’Agostino [GND] goodness-of-fit6Demler O.V. Paynter N.P. Cook N.R. Tests of calibration and goodness-of-fit in the survival setting.Stat Med. 2015; 34: 1659-1680https://doi.org/10.1002/sim.6428Crossref PubMed Scopus (175) Google Scholar) in CKiD data enhanced by additional follow-up and observed kidney failure events. CKiD is a North American longitudinal cohort of children with CKD with a primary end point of incident kidney replacement therapy (KRT; ie, kidney transplant or dialysis) for kidney failure (additional details in Item S1). We replicated the analytic structure in which the KFRE was first assessed,2Winnicki E. McCulloch C.E. Mitsnefes M.M. Furth S.L. Warady B.A. Ku E. Use of the kidney failure risk equation to determine the risk of progression to end-stage renal disease in children with chronic kidney disease.JAMA Pediatr. 2018; 172: 174-180https://doi.org/10.1001/jamapediatrics.2017.4083Crossref PubMed Scopus (36) Google Scholar with an additional 214 participants, extended follow-up, and estimation of albuminuria using pediatric-based equations.7Schneider M.F. Muñoz A. Ku E. Warady B.A. Furth S.L. Schwartz G.J. Estimation of albumin-creatinine ratio from protein-creatinine ratio in urine of children and adolescents with CKD.Am J Kidney Dis. 2021; 77: 824-827https://doi.org/10.1053/j.ajkd.2020.07.015Abstract Full Text Full Text PDF PubMed Scopus (6) Google Scholar The 2- and 5-year risk of KRT based on the 4-variable and 8-variable KFREs were calculated at the first visit when eGFR8Pierce C.B. Muñoz A. Ng D.K. Warady B.A. Furth S.L. Schwartz G.J. Age- and sex-dependent clinical equations to estimate glomerular filtration rates in children and young adults with chronic kidney disease.Kidney Int. 2021; 99: 948-956https://doi.org/10.1016/j.kint.2020.10.047Abstract Full Text Full Text PDF PubMed Scopus (78) Google Scholar <60 mL/min/1.73 m2 and all predictors were available. Albuminuria was measured centrally; if unavailable, it was estimated using validated equations.7Schneider M.F. Muñoz A. Ku E. Warady B.A. Furth S.L. Schwartz G.J. Estimation of albumin-creatinine ratio from protein-creatinine ratio in urine of children and adolescents with CKD.Am J Kidney Dis. 2021; 77: 824-827https://doi.org/10.1053/j.ajkd.2020.07.015Abstract Full Text Full Text PDF PubMed Scopus (6) Google Scholar Calibration plots and the GND goodness-of-fit test for survival data evaluated calibration. Using g bins based on similar KFRE 2- and 5-year probabilities of KRT with at least 5 events per bin,6Demler O.V. Paynter N.P. Cook N.R. Tests of calibration and goodness-of-fit in the survival setting.Stat Med. 2015; 34: 1659-1680https://doi.org/10.1002/sim.6428Crossref PubMed Scopus (175) Google Scholar the GND test compares the predicted (expected) risk with the observed risk estimated by 1 − Kaplan-Meier (KM) failure probability for the gth bin at time t and incorporates the variance of KMg(t). Under the null hypothesis, the test statistic follows a χ2 distribution with (g − 1) degrees of freedom with α = 0.05. Discrimination was quantified by the C statistic. All analyses were conducted in R version 4.0 (R Foundation for Statistical Computing). Table 1 presents clinical characteristics of this cohort of 817 children who provided complete data to calculate the 4-variable KFRE. Of these, 75% were followed >2.1 years, with 81 and 189 KRT events by 2 and 5 years, respectively. For 8-variable calculation, 799 participants had complete data. No deaths were observed within 5 years of follow-up.Table 1Clinical Characteristics of CKiD ParticipantsVariableCKiD Participants (N = 817)4-Variable KFRE predictors Age, y10.6 [5.9-14.3] Male sex509 (62%) eGFRaBased on the CKiD Under 25 (U25) estimated glomerular filtration rate (eGFR) equation., mL/min/1.73 m244 [33-52] Albuminuria,bWhen measured albuminuria was not available, it was estimated from total urine protein (in 489 [60%]). mg/g156 [35-522]Additional predictors for 8-variable KFREcN = 799 (18 had missing serum biomarker levels and were excluded). Serum albumin, mg/dL4.3 [4.1-4.6] Serum phosphate, mg/dL4.5 [4.1-5.0] Serum bicarbonate, mmol/L23 [20-25] Serum calcium, mg/dL9.6 [9.3-9.9]CKD characteristics Nonglomerular (congenital) CKD diagnosis627 (77%) Urinary protein-creatinine ratio, mg/g450 [180-1,310] Nephrotic-range proteinuria: >2,000 mg/g135 (17%)Longitudinal data and outcomes Median duration of follow-up, y4.6 [2.1-9.3] KRT within 2 y81 (10%) KRT within 5 y189 (23%)Values given as median [IQR] or count (percent).a Based on the CKiD Under 25 (U25) estimated glomerular filtration rate (eGFR) equation.b When measured albuminuria was not available, it was estimated from total urine protein (in 489 [60%]).c N = 799 (18 had missing serum biomarker levels and were excluded). Open table in a new tab Values given as median [IQR] or count (percent). Fig 1 presents the observed risk (1 − KM(2) and 1 − KM(5)) plotted against KFRE-predicted 2- and 5-year risk. For 2-year risk, both the 4- and 8-variable KFREs overestimated observed risk among those with higher KFRE scores. For 5-year risk, both KFREs underestimated risk at lower predicted probabilities and overestimated risk at higher probabilities. For all 4 equations, predicted and observed risk were significantly different (P < 0.001), indicating poor calibration. Similar results and inferences were observed in sensitivity analyses (stratified by glomerular versus nonglomerular diagnoses and restricted to measured albuminuria only; Tables S1 and S2). Discrimination was high (C statistic of 0.814-0.871). The KFRE demonstrated poor calibration for both 2- and 5-year risk of KRT in this external cohort. This was despite strong discrimination (replicating previous results2Winnicki E. McCulloch C.E. Mitsnefes M.M. Furth S.L. Warady B.A. Ku E. Use of the kidney failure risk equation to determine the risk of progression to end-stage renal disease in children with chronic kidney disease.JAMA Pediatr. 2018; 172: 174-180https://doi.org/10.1001/jamapediatrics.2017.4083Crossref PubMed Scopus (36) Google Scholar) and underscores the distinction between calibration and discrimination as 2 necessary but disparate measures of validity. The KFRE predictors are key biomarkers related to CKD progression in both children and adults, which explains why discrimination was high. Specifically, eGFR is often used to decide to initiate KRT. In addition to poor calibration, there are epidemiologic considerations when deciding to apply the KFRE to a pediatric CKD population. The interpretation of the KFRE age coefficient is that as age increases, the risk of KRT decreases (ie, 20% lower hazard per 10 years older). In marked contrast, pediatric patients with CKD are known to experience increasing KRT risk with older age.9Ng D.K. Matheson M.B. Warady B.A. Mendley S.R. Furth S.L. Muñoz A. Incidence of initial renal replacement therapy over the course of kidney disease in children.Am J Epidemiol. 2019; 188: 2156-2164https://doi.org/10.1093/aje/kwz220Crossref PubMed Scopus (8) Google Scholar Also, the KFRE was developed among older adults with CKD (average age: 70 years) with stark differences in etiology and natural history compared to a young pediatric CKD population with mostly congenital kidney or urinary tract diseases. Another structural incongruity is that the 8-variable KFRE includes absolute level of phosphorus, yet normative phosphorus levels differ substantially between children and adults. Prognostic tools are critical for clinical management of CKD. Clinicians should therefore have confidence that equations they use are designed for, and specific to, their patient population, with both strong discrimination and calibration, especially for children. Despite valid application across adult populations, the KFRE had poor calibration in children with CKD but may be suitable if discrimination only were of interest. For pediatric clinical settings where calibration is necessary, we recommend pediatric-specific kidney failure risk prediction calculators.10Furth S.L. Pierce C. Hui W.F. et al.Estimating time to ESRD in children with CKD.Am J Kidney Dis. 2018; 71: 783-792https://doi.org/10.1053/j.ajkd.2017.12.011Abstract Full Text Full Text PDF PubMed Scopus (48) Google Scholar A list of the CKiD Study Investigators can be found in Table S3 and at https://statepi.jhsph.edu/ckid/site-investigators/. Research idea and study design: DKN, GM; data acquisition: DKN; data analysis/interpretation: GM, DKN, CBP; statistical analysis: GM, DKN; supervision or mentorship: DKN, CBP; obtained funding: DKN. Each author contributed important intellectual content during manuscript drafting or revision and agrees to be personally accountable for the individual’s own contributions and to ensure that questions pertaining to the accuracy or integrity of any portion of the work, even one in which the author was not directly involved, are appropriately investigated and resolved, including with documentation in the literature if appropriate. Data used herein were collected by the CKiD Study with clinical coordinating centers at Children’s Mercy Hospital and the University of Missouri–Kansas City (Principal Investigator [PI]: Bradley Warady, MD) and Children’s Hospital of Philadelphia (PI: Susan Furth, MD, PhD), Central Biochemistry Laboratory (PI: George Schwartz, MD) at the University of Rochester Medical Center, and data coordinating center (PIs: Alvaro Muñoz, PhD and DKN) at the Johns Hopkins Bloomberg School of Public Health. CKiD is funded by the National Institute of Diabetes and Digestive and Kidney Diseases, with additional funding from the National Institute of Child Health and Human Development and the National Heart, Lung, and Blood Institute (U01-DK-66143, U01-DK-66174, U24-DK-082194, U24-DK-66116). The authors declare that they have no relevant financial interests. Received June 20, 2022. Evaluated by 3 external peer reviewers and a Statistics/Methods Editor, with editorial input from an Acting Editor-in-Chief (Editorial Board Member Joseph Chin-Chi Kuo, MD, PhD). Accepted in revised form November 5, 2022. The involvement of an Acting Editor-in-Chief to handle the peer-review and decision-making processes was to comply with AJKD’s procedures for potential conflicts of interest for editors, described in the Information for Authors & Journal Policies. Download .pdf (.19 MB) Help with pdf files Supplementary File (PDF)Item S1; Tables S1-S3.
MoreTranslated text
AI Read Science
Must-Reading Tree
Example
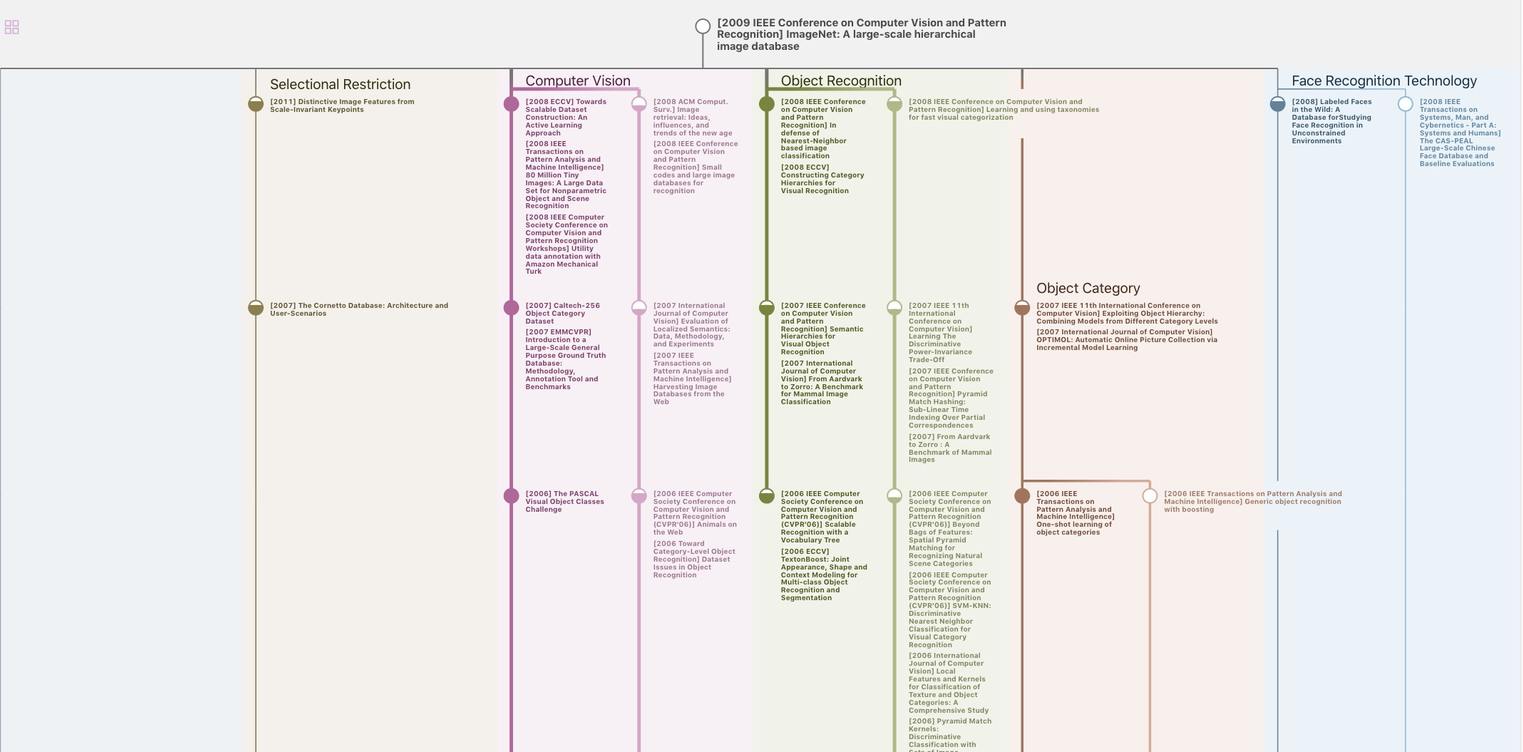
Generate MRT to find the research sequence of this paper
Chat Paper
Summary is being generated by the instructions you defined