Near‐term forecasts of stream temperature using deep learning and data assimilation in support of management decisions
JAWRA Journal of the American Water Resources Association(2022)
摘要
Deep learning (DL) models are increasingly used to make accurate hindcasts of management-relevant variables, but they are less commonly used in forecasting applications. Data assimilation (DA) can be used for forecasts to leverage real-time observations, where the difference between model predictions and observations today is used to adjust the model to make better predictions tomorrow. In this use case, we developed a process-guided DL and DA approach to make 7-day probabilistic forecasts of daily maximum water temperature in the Delaware River Basin in support of water management decisions. Our modeling system produced forecasts of daily maximum water temperature with an average root mean squared error (RMSE) from 1.1 to 1.4 degrees C for 1-day-ahead and 1.4 to 1.9 degrees C for 7-day-ahead forecasts across all sites. The DA algorithm marginally improved forecast performance when compared with forecasts produced using the process-guided DL model alone (0%-14% lower RMSE with the DA algorithm). Across all sites and lead times, 65%-82% of observations were within 90% forecast confidence intervals, which allowed managers to anticipate probability of exceedances of ecologically relevant thresholds and aid in decisions about releasing reservoir water downstream. The flexibility of DL models shows promise for forecasting other important environmental variables and aid in decision-making.
更多查看译文
关键词
stream temperature,deep learning,data assimilation,forecasting,water management,drinking water reservoirs,stream habitat
AI 理解论文
溯源树
样例
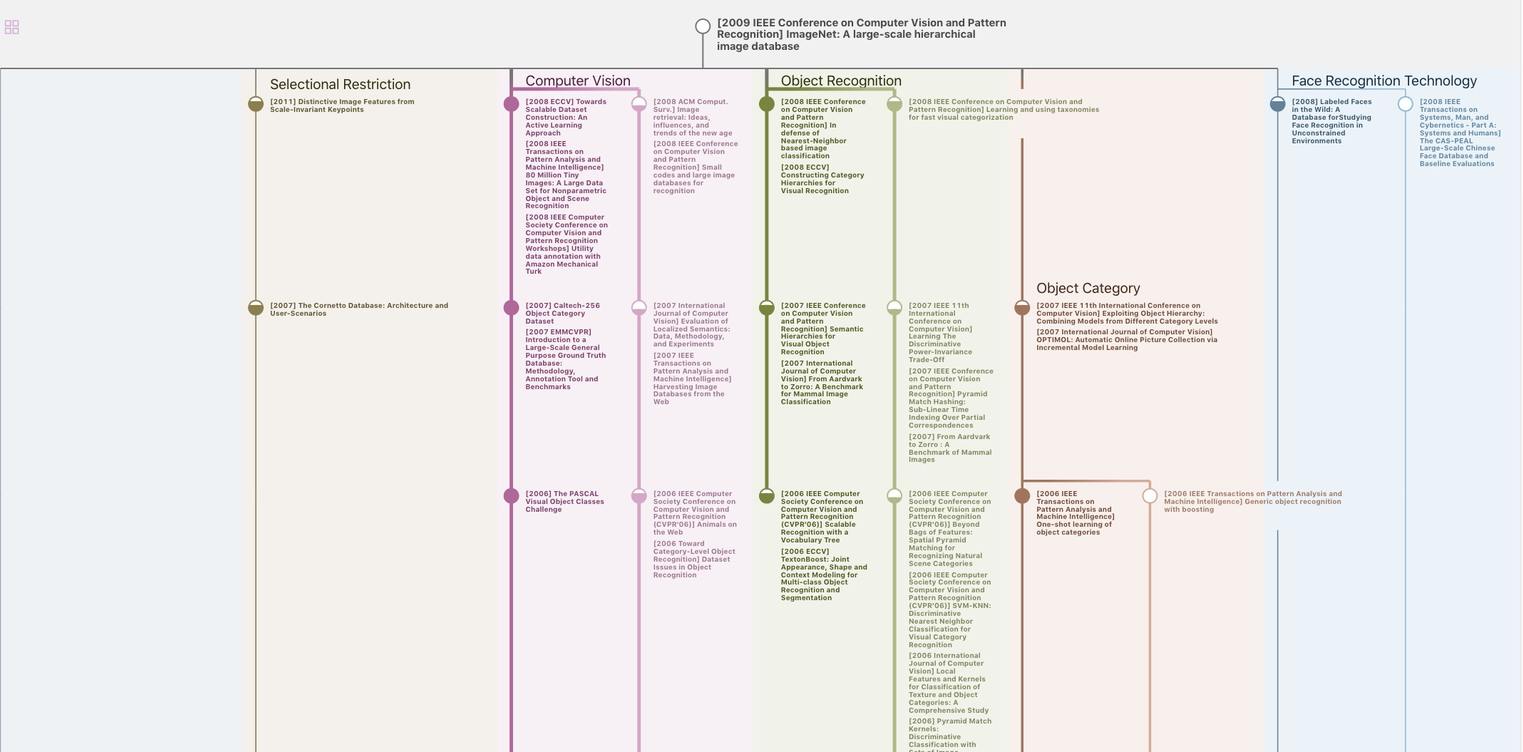
生成溯源树,研究论文发展脉络
Chat Paper
正在生成论文摘要