Early Time Series Classification Using TCN-Transformer
2022 IEEE 4th International Conference on Civil Aviation Safety and Information Technology (ICCASIT)(2022)
摘要
Early time series classification is of great significance for time-sensitive applications such as fault detection and earthquake prediction. This task aims to classify time series with the least timestamps at desired accuracy. Recent deep learning methods usually used the Recurrent Neural Networks (RNNs) as the classification backbone and the exiting subnet for early quitting. However, the RNNs suffer from the ‘forgetting’ defect and insufficient local feature extraction. Besides, the balance between earliness and accuracy is not fully considered. In this paper, a framework named TCN-Transformer is proposed. To overcome the defects of RNNs, we combined Temporal Convolutional Network and Transformer to extract both local and global features. Then, a loss function is designed to ensure the classification performance, while focusing more on earlier features. The experimental results on ten univariate datasets.
更多查看译文
关键词
early classification,Transformer,deep learning
AI 理解论文
溯源树
样例
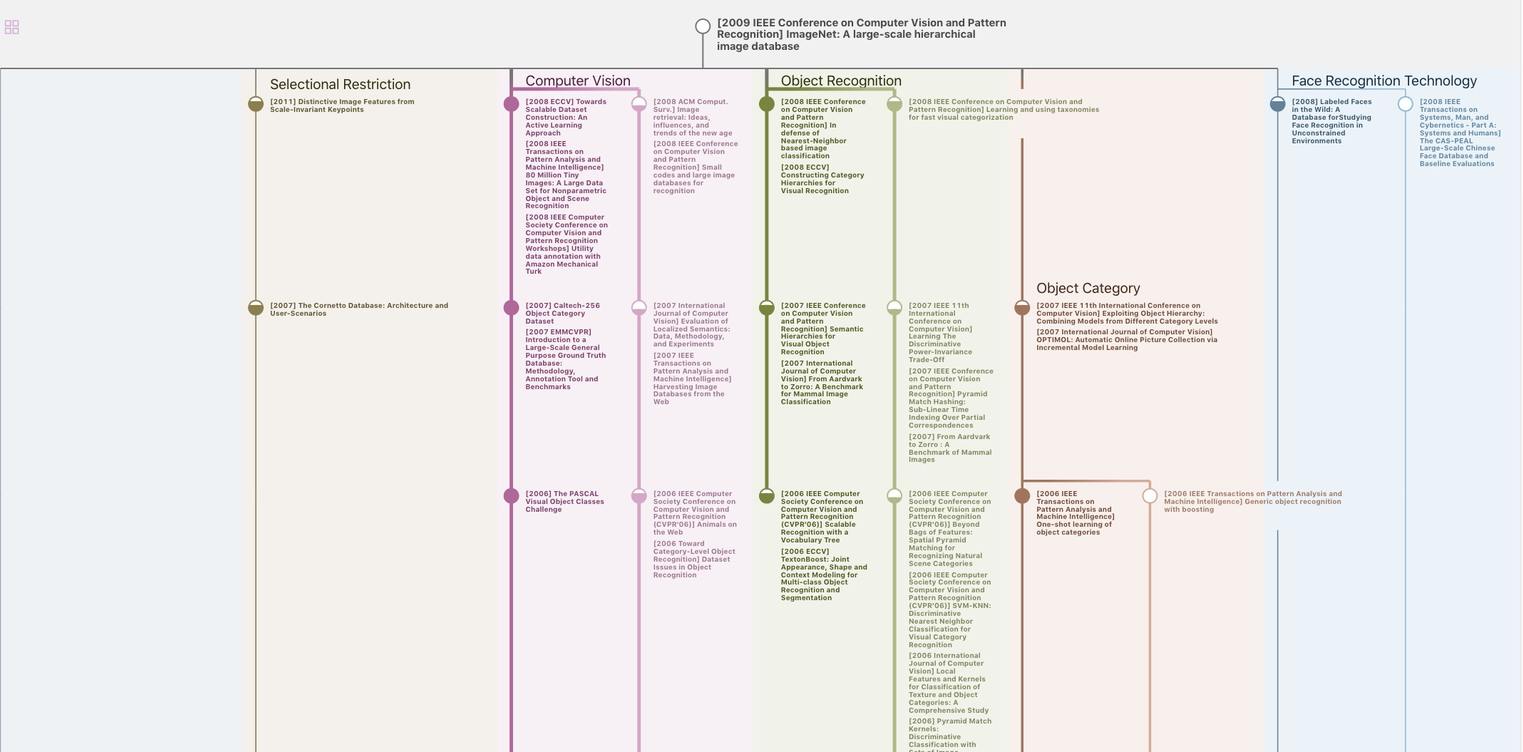
生成溯源树,研究论文发展脉络
Chat Paper
正在生成论文摘要