Detecting Anomalies in Quick Access Recorder Data
2022 IEEE 4th International Conference on Civil Aviation Safety and Information Technology (ICCASIT)(2022)
摘要
For the civil aviation industry, QAR provides a gold mine of data for a wide range of applications. The data quality is an essential precondition for its usage. Thus, to detect the abnormalities or outliers is an important procedure for its further utilization. In this study, we adopted four approaches to detect the abnormalities, i.e. Pauta criterion, boxplot, K-means algorithm and LOF algorithms. Results show that these approaches perform differently for various parameters in a QAR data set. The Pauta criterion seems to be not working well for most of the QAR parameters. In contrast, the K-means clustering and LOF algorithms generally work well with the selected QAR parameters.
更多查看译文
关键词
anomalies,data,access
AI 理解论文
溯源树
样例
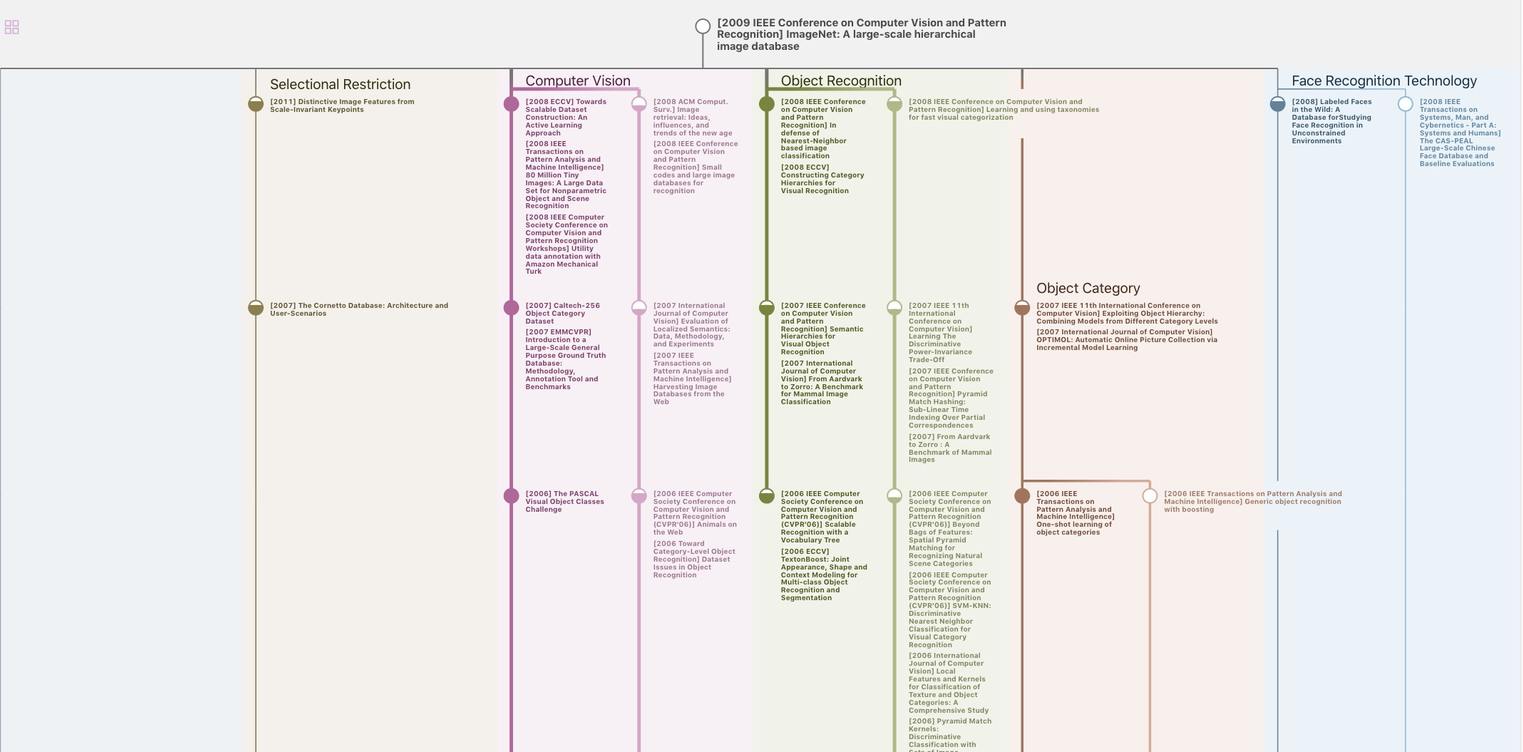
生成溯源树,研究论文发展脉络
Chat Paper
正在生成论文摘要