NG-GAN: A Robust Noise-Generation Generative Adversarial Network for Generating Old-Image Noise.
Sensors (Basel, Switzerland)(2022)
Abstract
Numerous old images and videos were captured and stored under unfavorable conditions. Hence, old images and videos have uncertain and different noise patterns compared with those of modern ones. Denoising old images is an effective technique for reconstructing a clean image containing crucial information. However, obtaining noisy-clean image pairs for denoising old images is difficult and challenging for supervised learning. Preparing such a pair is expensive and burdensome, as existing denoising approaches require a considerable number of noisy-clean image pairs. To address this issue, we propose a robust noise-generation generative adversarial network (NG-GAN) that utilizes unpaired datasets to replicate the noise distribution of degraded old images inspired by the CycleGAN model. In our proposed method, the perception-based image quality evaluator metric is used to control noise generation effectively. An unpaired dataset is generated by selecting clean images with features that match the old images to train the proposed model. Experimental results demonstrate that the dataset generated by our proposed NG-GAN can better train state-of-the-art denoising models by effectively denoising old videos. The denoising models exhibit significantly improved peak signal-to-noise ratios and structural similarity index measures of 0.37 dB and 0.06 on average, respectively, on the dataset generated by our proposed NG-GAN.
MoreTranslated text
Key words
generative adversarial network,image denoising,noise generation,perception-based image quality evaluator,recurrent residual channel and spatial attention
AI Read Science
Must-Reading Tree
Example
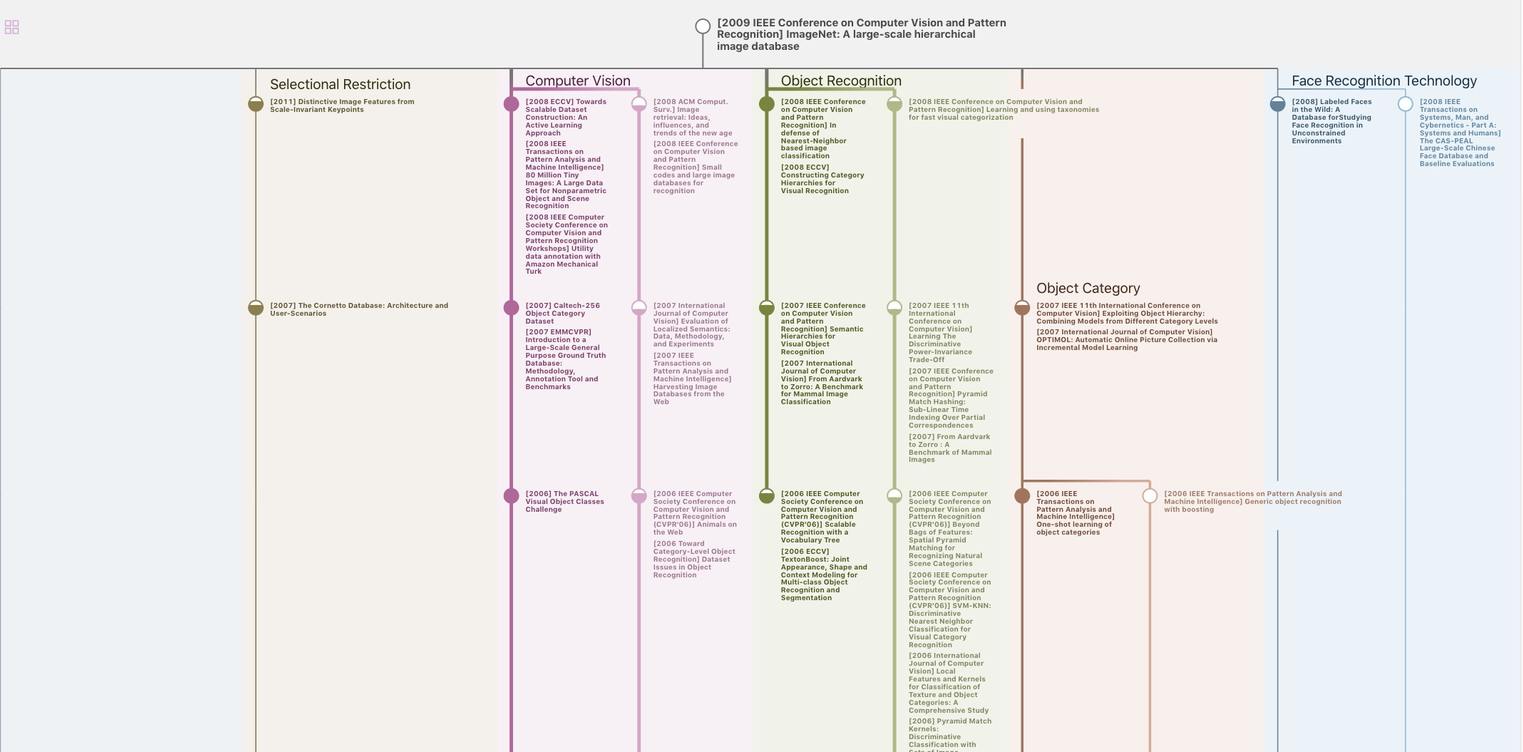
Generate MRT to find the research sequence of this paper
Chat Paper
Summary is being generated by the instructions you defined