Disk-Graph Probabilistic Roadmap: Biased Distance Sampling for Path Planning in a Partially Unknown Environment
2022 IEEE/RSJ International Conference on Intelligent Robots and Systems (IROS)(2022)
摘要
In this paper, we propose a new sampling-based path planning approach, focusing on the challenges linked to autonomous exploration. Our method relies on the definition of a disk graph of free-space bubbles, from which we derive a biased sampling function that expands the graph towards known free space for maximal navigability and frontiers discovery. The proposed method demonstrates an exploratory behavior similar to Rapidly-exploring Random Trees, while retaining the connectivity and flexibility of a graph-based planner. We demonstrate the interest of our method by first comparing its path planning capabilities against state-of-the-art approaches, before discussing exploration-specific aspects, namely replanning capabilities and incremental construction of the graph. A simple frontiers-driven exploration controller derived from our planning method is also demonstrated using the Pioneer platform.
更多查看译文
关键词
path planning,biased distance sampling,disk-graph
AI 理解论文
溯源树
样例
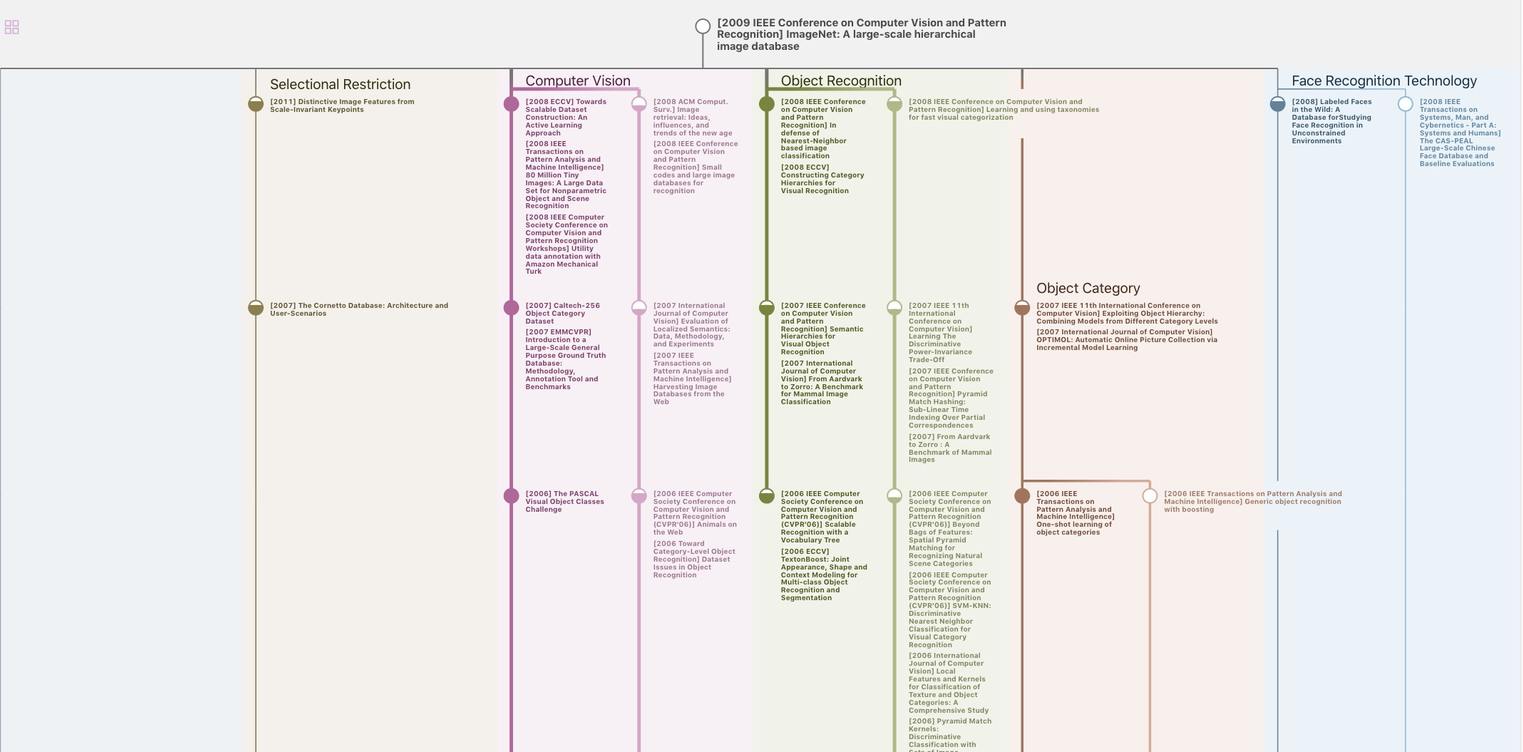
生成溯源树,研究论文发展脉络
Chat Paper
正在生成论文摘要