Towards Adaptive Continuous Control of Soft Robotic Manipulator using Reinforcement Learning
2022 IEEE/RSJ International Conference on Intelligent Robots and Systems (IROS)(2022)
Abstract
Although the soft robot is gaining considerable popularity in dexterous and safe manipulation, accurate motion control is still an open problem to be explored. Recent investigations suggest that reinforcement learning (RL) is a promising solution but lacks efficient adaptability for Sim2Real transfer or environment variations. In this paper, we present a deep deterministic policy gradient (DDPG)-based control system for the continuous task-space manipulation of soft robots. Domain randomization is adopted in simulation for fast control-policy initialization, while an offline retraining strategy is utilized to update the controller parameters for incremental learning. The experiments demonstrate that the proposed RL controller can track a moving target accurately (with RMSE of 1.26 mm), and accommodate to external varying load effectively (with similar to 30% RMSE reduction after retraining). Comparisons among the proposed RL controller and other supervised-learning-based controllers in handling additional tip load were also conducted. The results support that our RL method is appropriate for automatic learning such that there is no need of manual interference for data processing, particularly in cases with external disturbances and actuation redundancy.
MoreTranslated text
Key words
Deep reinforcement learning, Domain randomization, Incremental learning, Learning-based control, Soft robot control
AI Read Science
Must-Reading Tree
Example
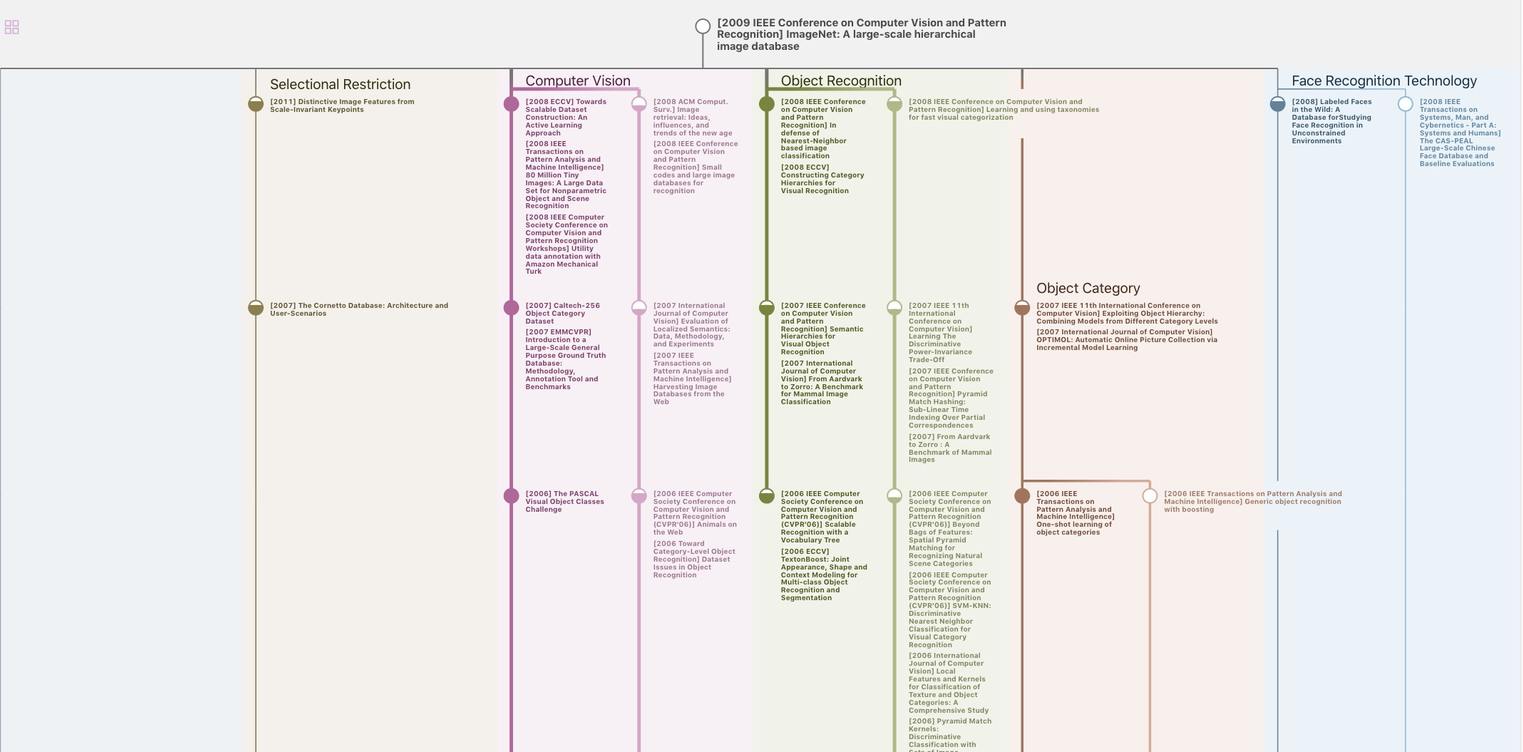
Generate MRT to find the research sequence of this paper
Chat Paper
Summary is being generated by the instructions you defined