A Deep-Learning-based System for Indoor Active Cleaning
2022 IEEE/RSJ International Conference on Intelligent Robots and Systems (IROS)(2022)
摘要
Cleaning public areas like commercial complexes is challenging due to their sophisticated surroundings and the vast kinds of real-life dirt. Robots are required to distinguish dirts and apply corresponding cleaning strategies. In this work, we proposed an active-cleaning framework by utilizing deep-learning methods for both solid wastes detection and liquid stains segmentation. Our system consists of 4 components: a Perception module integrated with deep-learning models, a Post-processing module for projection, a Tracking module for map localization, and a Planning and Control module for cleaning strategies. Compared with classic approaches, our vision-based system significantly improves cleaning efficiency. Besides, we released the largest real-world indoor hybrid dirt cleaning dataset (HD10K) containing 10K labeled images, together with a track-level evaluation metric for better cleaning performance measurement. The proposed deep-learning based system is verified with extensive experiments on our dataset, and deployed to Gaussian Robotics's robots operating globally. Dataset is available at: https://gaussianopensource.github.io/projects/active_cleaning.
更多查看译文
关键词
active-cleaning framework,cleaning performance measurement,deep-learning based system,deep-learning methods,deep-learning models,indoor active cleaning,liquid stains segmentation,map localization,Perception module,Planning and Control module,Post-processing module,real-world indoor hybrid dirt cleaning dataset,solid wastes detection,track-level evaluation,Tracking module,vision-based system
AI 理解论文
溯源树
样例
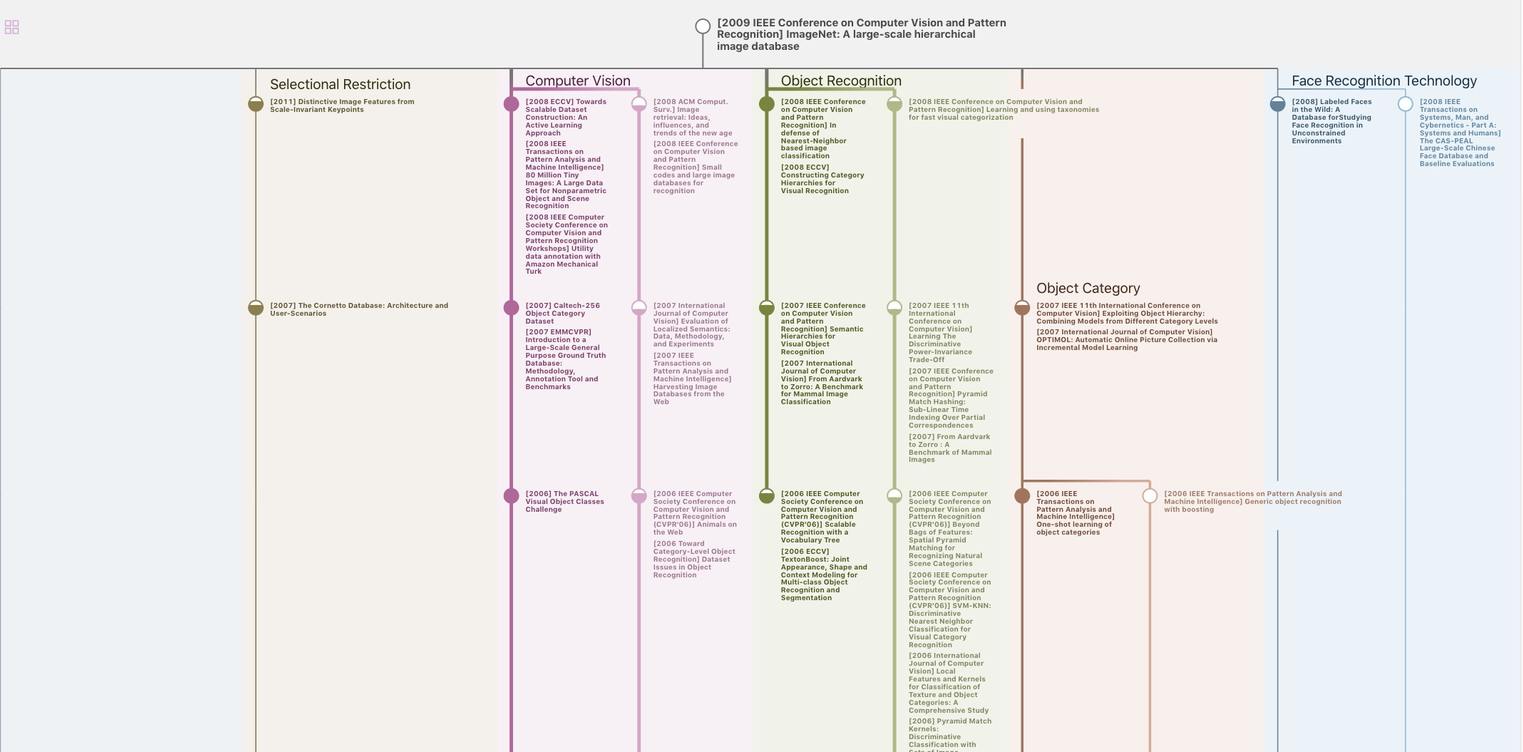
生成溯源树,研究论文发展脉络
Chat Paper
正在生成论文摘要