Learning Symbolic Failure Detection for Grasping and Mobile Manipulation Tasks
2022 IEEE/RSJ International Conference on Intelligent Robots and Systems (IROS)(2022)
摘要
The ability to detect failure during task execution and to recover from failure is vital for autonomous robots performing tasks in previously unknown environments. In this paper, we present an approach for failure detection during the execution of grasping and mobile manipulation tasks by a humanoid robot. The approach combines multi-modal sensory information consisting of proprioceptive, force and visual information to learn task models from multiple successful task executions, in order to detect failures and to externalize them for humans in an interpretable way. To this end, we define symbolic action predicates based on multi-modal sensory information to allow high-level state estimation based on action-specific decision trees. To allow symbolic failure detection, we then learn task models that are represented as Markov chains. We evaluated the approach in several pick-and-place and mobile manipulation tasks performed by a humanoid robot in a decommissioning and a household scenario. The evaluation shows that the learned task models are capable of detecting failure with an F1-score of 93%.
更多查看译文
关键词
action-specific decision trees,F1-score,failure detection,high-level state estimation,household scenario,humanoid robot,mobile manipulation tasks,multimodal sensory information,pick-and-place,symbolic failure detection,task execution,task models,visual information
AI 理解论文
溯源树
样例
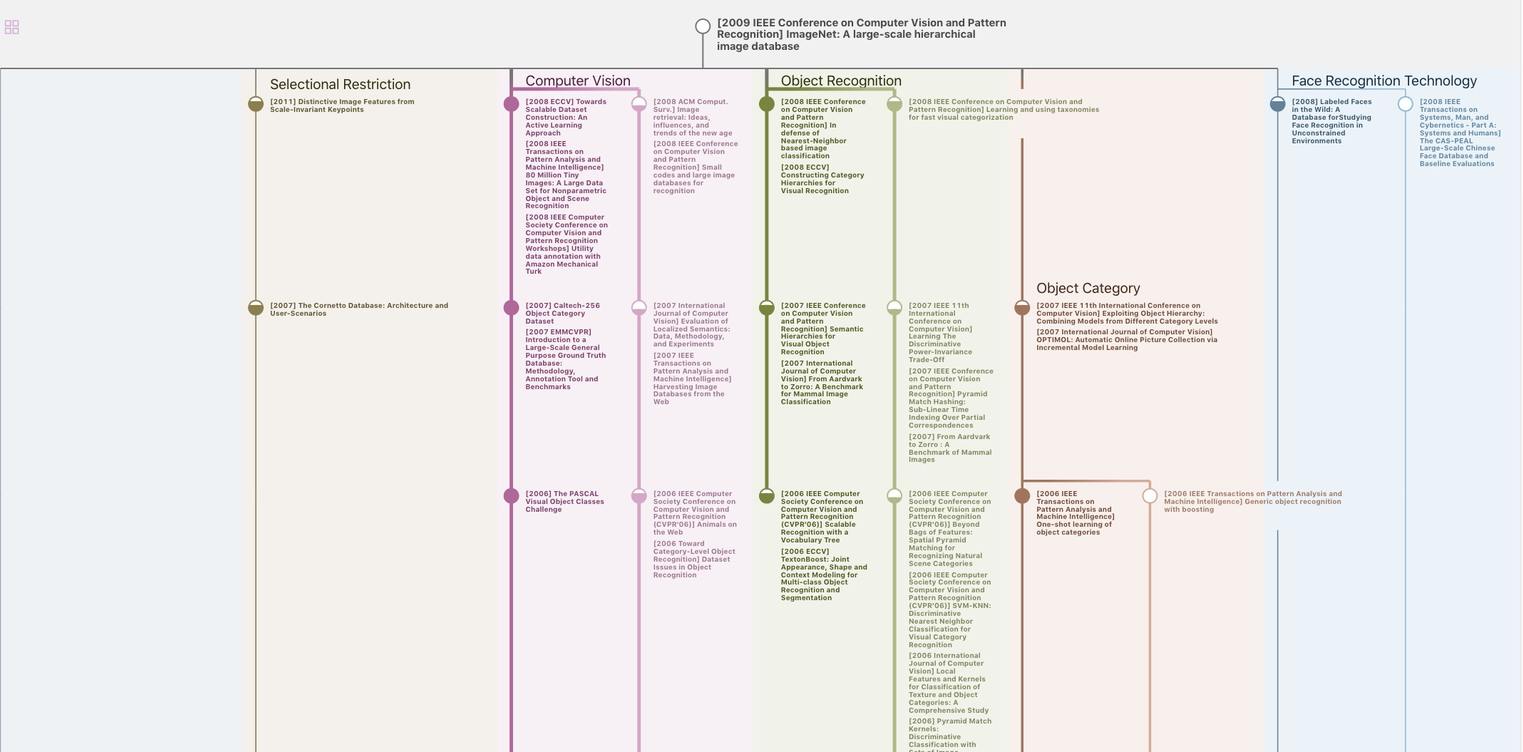
生成溯源树,研究论文发展脉络
Chat Paper
正在生成论文摘要