Skip-Concatenated Image Super-Resolution Network for Mobile Devices.
IEEE Access(2023)
摘要
Single-image super-resolution technology has been widely studied in various applications to improve the quality and resolution of degraded images acquired from noise-sensitive low-resolution sensors. As most studies on single-image super-resolution focused on the development of deep learning networks operating on high-performance GPUs, this study proposed an efficient and lightweight super-resolution network that enables real-time performance on mobile devices. To replace the relatively slow element-wise addition layer on mobile devices, we introduced a skip connection layer by directly concatenating a low-resolution input image with an intermediate feature map. In addition, we introduced weighted clipping to reduce the quantization errors commonly encountered during float-to-int8 model conversion. Moreover, a reparameterization method was selectively applied without increasing the cost in terms of inference time and number of parameters. Based on the contributions, the proposed network has been recognized as the best solution in Mobile AI & AIM 2022 Real-Time Single-Image Super-Resolution Challenge with PSNR of 30.03 dB and NPU runtime of 19.20 ms.
更多查看译文
关键词
Quantization (signal),Training,Superresolution,Mobile handsets,Image reconstruction,Image quality,Task analysis,Concatenation,mobile super resolution,quantization-aware training,single image super resolution,TFLite
AI 理解论文
溯源树
样例
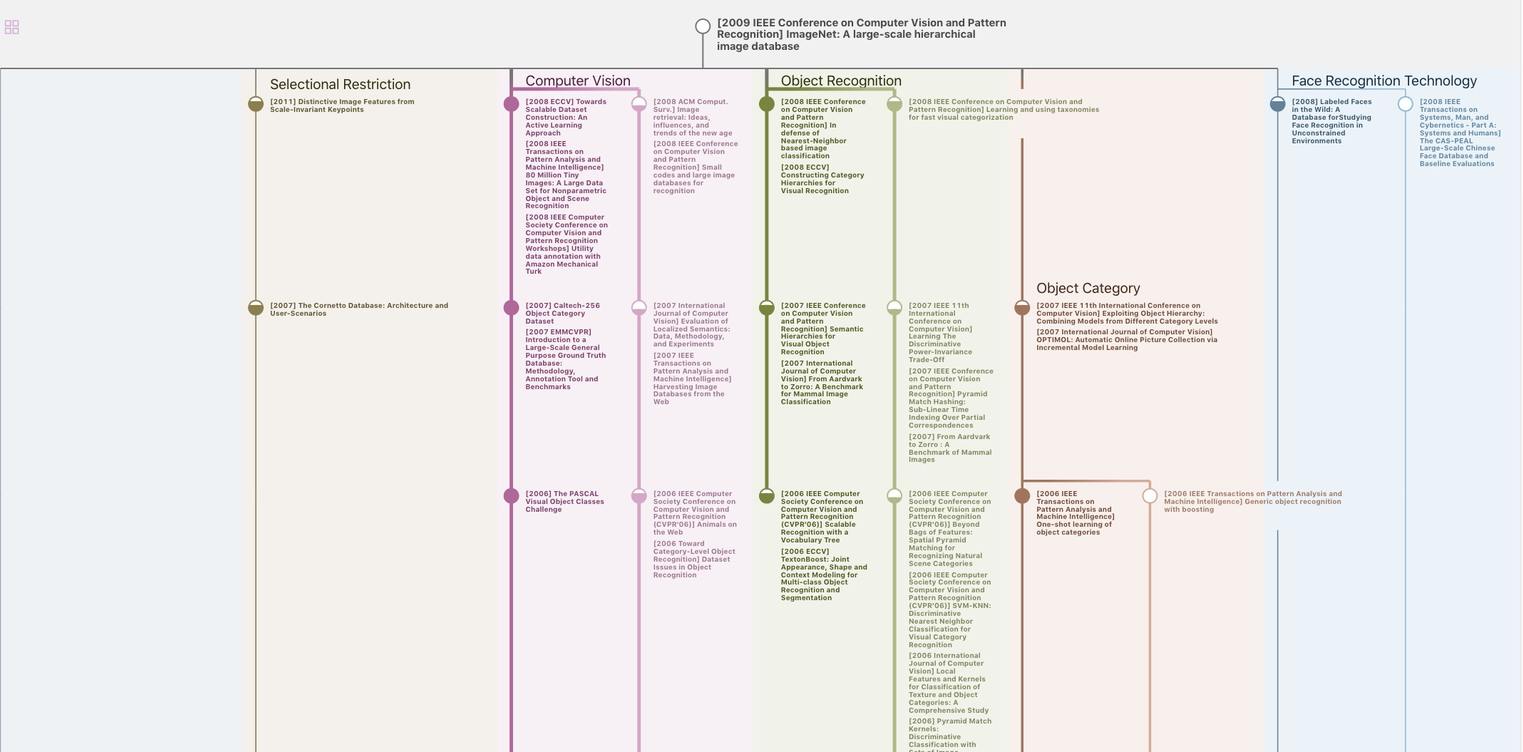
生成溯源树,研究论文发展脉络
Chat Paper
正在生成论文摘要