Neural Brushstroke Engine: Learning a Latent Style Space of Interactive Drawing Tools
ACM TRANSACTIONS ON GRAPHICS(2022)
摘要
We propose Neural Brushstroke Engine, the first method to apply deep generative models to learn a distribution of interactive drawing tools. Our conditional GAN model learns the latent space of drawing styles from a small set (about 200) of unlabeled images in different media. Once trained, a single model can texturize stroke patches drawn by the artist, emulating a diverse collection of brush styles in the latent space. In order to enable interactive painting on a canvas of arbitrary size, we design a painting engine able to support real-time seamless patch-based generation, while allowing artists direct control of stroke shape, color and thickness. We show that the latent space learned by our model generalizes to unseen drawing and more experimental styles (e.g. beads) by embedding real styles into the latent space. We explore other applications of the continuous latent space, such as optimizing brushes to enable painting in the style of an existing artwork, automatic line drawing stylization, brush interpolation, and even natural language search over a continuous space of drawing tools. Our prototype received positive feedback from a small group of digital artists.
更多查看译文
关键词
GANs,example-based media,digital brushes
AI 理解论文
溯源树
样例
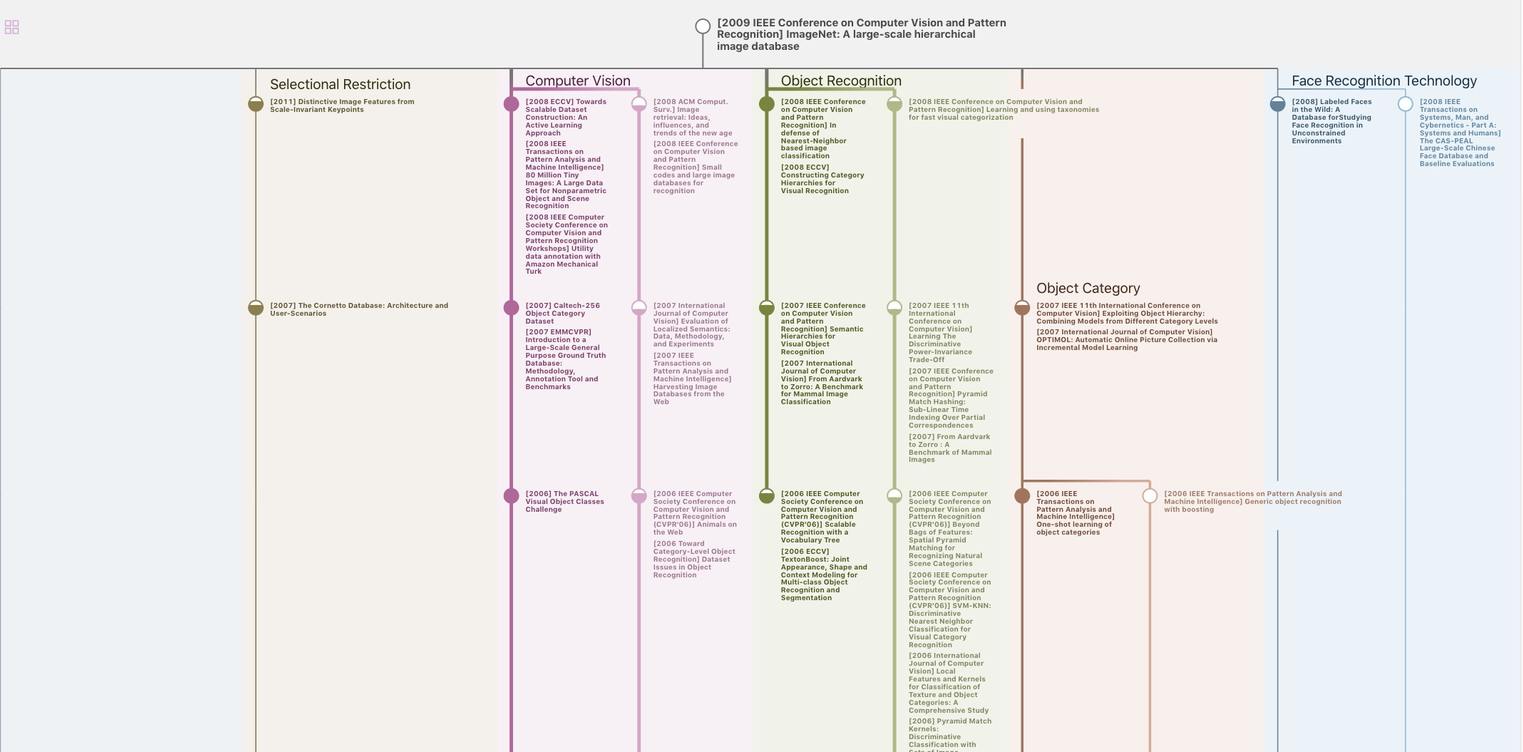
生成溯源树,研究论文发展脉络
Chat Paper
正在生成论文摘要