CrowdGraph: A Crowdsourcing Multi-modal Knowledge Graph Approach to Explainable Fauxtography Detection
Proceedings of the ACM on Human-Computer Interaction(2022)
摘要
AbstractHuman-centric fauxtography is a category of multi-modal posts that spread misleading information on online information distribution and sharing platforms such as online social media. The reason of a human-centric post being fauxtography is closely related to its multi-modal content that consists of diversified human and non-human subjects with complex and implicit relationships. In this paper, we focus on an explainable fauxtography detection problem where the goal is to accurately identify and explain why a human-centric social media post is fauxtography (or not). Our problem is motivated by the limitations of current fauxtography detection solutions that focus primarily on the detection task but ignore the important aspect of explaining their results (e.g., why a certain component of the post delivers the misinformation). Two important challenges exist in solving our problem: 1) it is difficult to capture the implicit relations and attributions of different subjects in a fauxtography post given the fact that many of such knowledge is shared between different crowd workers; 2) it is not a trivial task to create a multi-modal knowledge graph from crowd workers to identify and explain human-centric fauxtography posts with multi-modal contents. To address the above challenges, we develop CrowdGraph, a crowdsourcing based multi-modal knowledge graph approach to address the explainable fauxtography detection problem. We evaluate the performance of CrowdGraph by creating a real-world dataset that consists of human-centric fauxtography posts from Twitter and Reddit. The results show that CrowdGraph not only detects the fauxtography posts more accurately than the state-of-the-arts but also provides well-justified explanations to the detection results with convincing evidence.
更多查看译文
AI 理解论文
溯源树
样例
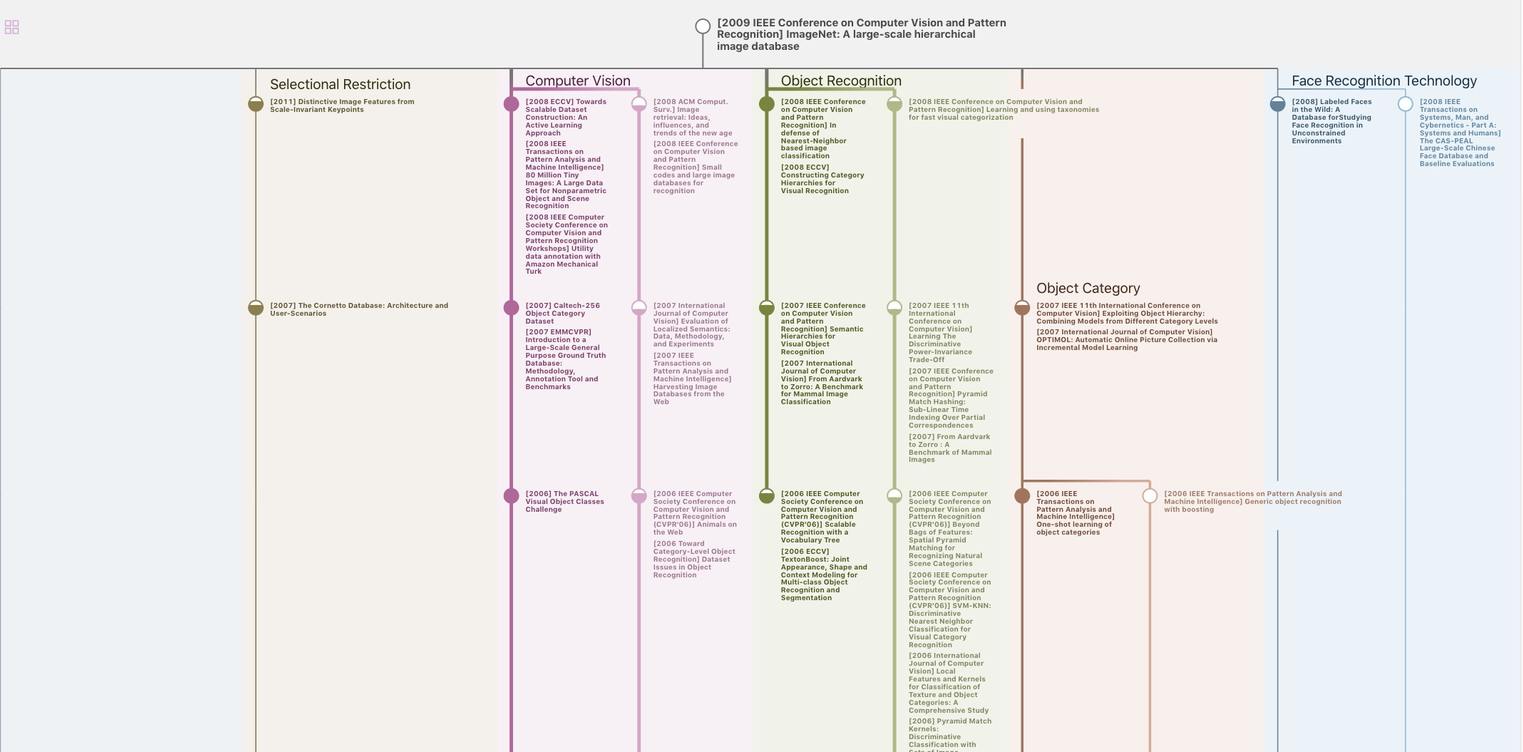
生成溯源树,研究论文发展脉络
Chat Paper
正在生成论文摘要