Affective Interaction: Attentive Representation Learning for Multi-Modal Sentiment Classification
ACM Transactions on Multimedia Computing, Communications, and Applications(2022)
摘要
AbstractThe recent booming of artificial intelligence (AI) applications, e.g., affective robots, human-machine interfaces, autonomous vehicles, and so on, has produced a great number of multi-modal records of human communication. Such data often carry latent subjective users’ attitudes and opinions, which provides a practical and feasible path to realize the connection between human emotion and intelligence services. Sentiment and emotion analysis of multi-modal records is of great value to improve the intelligence level of affective services. However, how to find an optimal manner to learn people’s sentiments and emotional representations has been a difficult problem, since both of them involve subtle mind activity. To solve this problem, a lot of approaches have been published, but most of them are insufficient to mine sentiment and emotion, since they have treated sentiment analysis and emotion recognition as two separate tasks. The interaction between them has been neglected, which limits the efficiency of sentiment and emotion representation learning. In this work, emotion is seen as the external expression of sentiment, while sentiment is the essential nature of emotion. We thus argue that they are strongly related to each other where one’s judgment helps the decision of the other. The key challenges are multi-modal fused representation and the interaction between sentiment and emotion. To solve such issues, we design an external knowledge enhanced multi-task representation learning network, termed KAMT. The major elements contain two attention mechanisms, which are inter-modal and inter-task attentions and an external knowledge augmentation layer. The external knowledge augmentation layer is used to extract the vector of the participant’s gender, age, occupation, and of overall color or shape. The main use of inter-modal attention is to capture effective multi-modal fused features. Inter-task attention is designed to model the correlation between sentiment analysis and emotion classification. We perform experiments on three widely used datasets, and the experimental performance proves the effectiveness of the KAMT model.
更多查看译文
关键词
Multi-modal sentiment analysis,emotion recognition,representation learning,deep learning,artificial intelligence
AI 理解论文
溯源树
样例
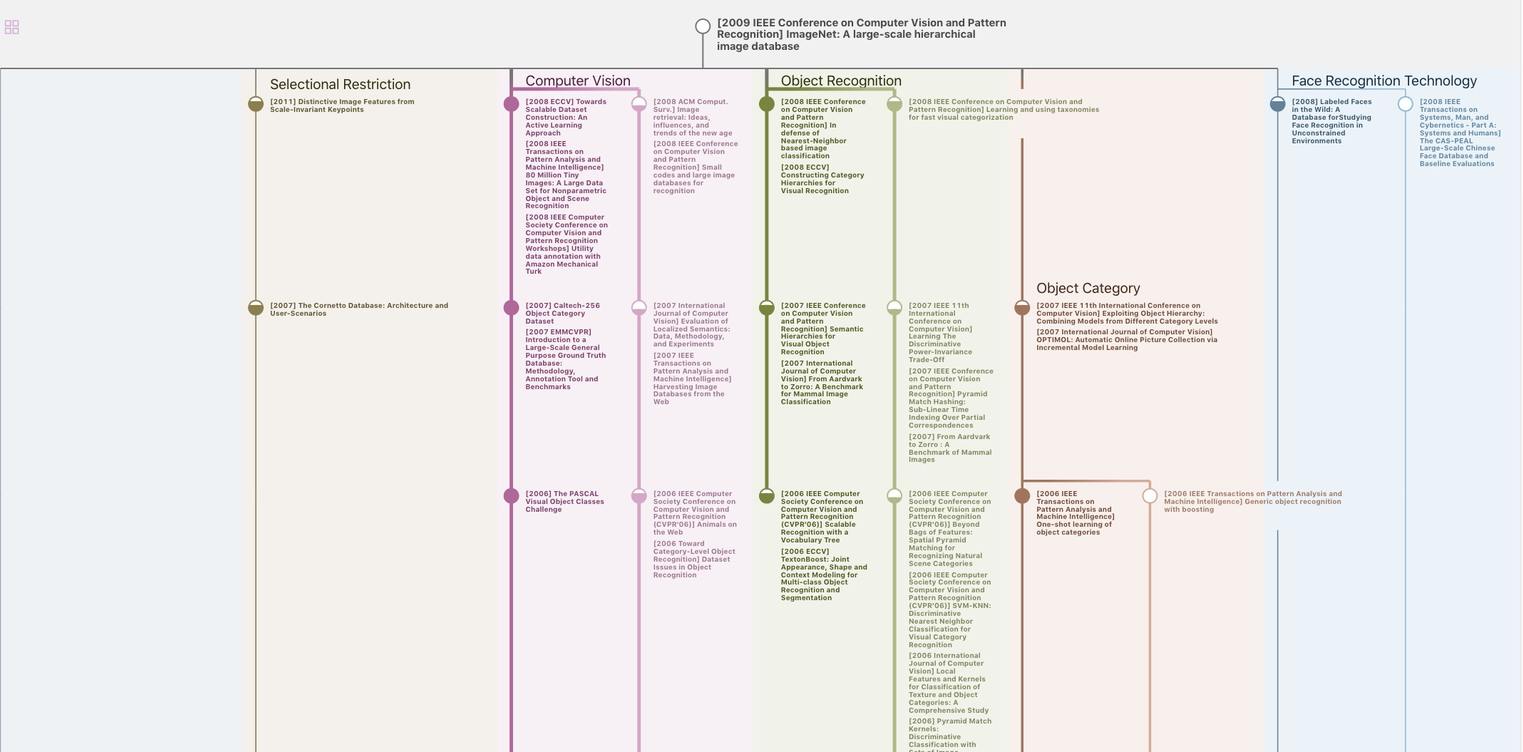
生成溯源树,研究论文发展脉络
Chat Paper
正在生成论文摘要