Emotions Mater: Towards Personalizing Human-System Interactions Using a Two-layer Multimodal Approach
Multimodal Interfaces and Machine Learning for Multimodal Interaction(2022)
摘要
Monitoring and predicting user task performance is critical as it provides valuable insights for developing personalized human-system interactions. Key factors that impact task performance include cognitive workload, physiological responses, and afective states. However, a lack of consideration of any of these factors could lead to inaccurate task performance prediction because of their interplay. To address this challenge, we developed a novel hierarchical machine learning approach that considers these three factors to predict task performance. We exposed twenty-eight participants to a twostep experimental study. The frst step aimed to induce diferent afective states using a validated video database. The second step required participants to perform validated low and high cognitive workload-inducing tasks. To evaluate the performance, we compared the models developed using the hierarchical approach that uses emotional and physiological information, to models that use only physiological information. We observed that our proposed approach always outperformed the models that only use physiological information to predict task performance by achieving a better average person independent mean absolute error. However, information gained across various models using the hierarchical approach was not linear. Additionally, we found that the top predictors for each model varied, and the model with the highest information gain included emotional features. These fndings suggest the importance of choosing the appropriate machine learning model and predictors for building robust models for predicting task performance.
更多查看译文
关键词
Machine learning, Afective Computing, Task performance, Physiological signals, Cognitive workload
AI 理解论文
溯源树
样例
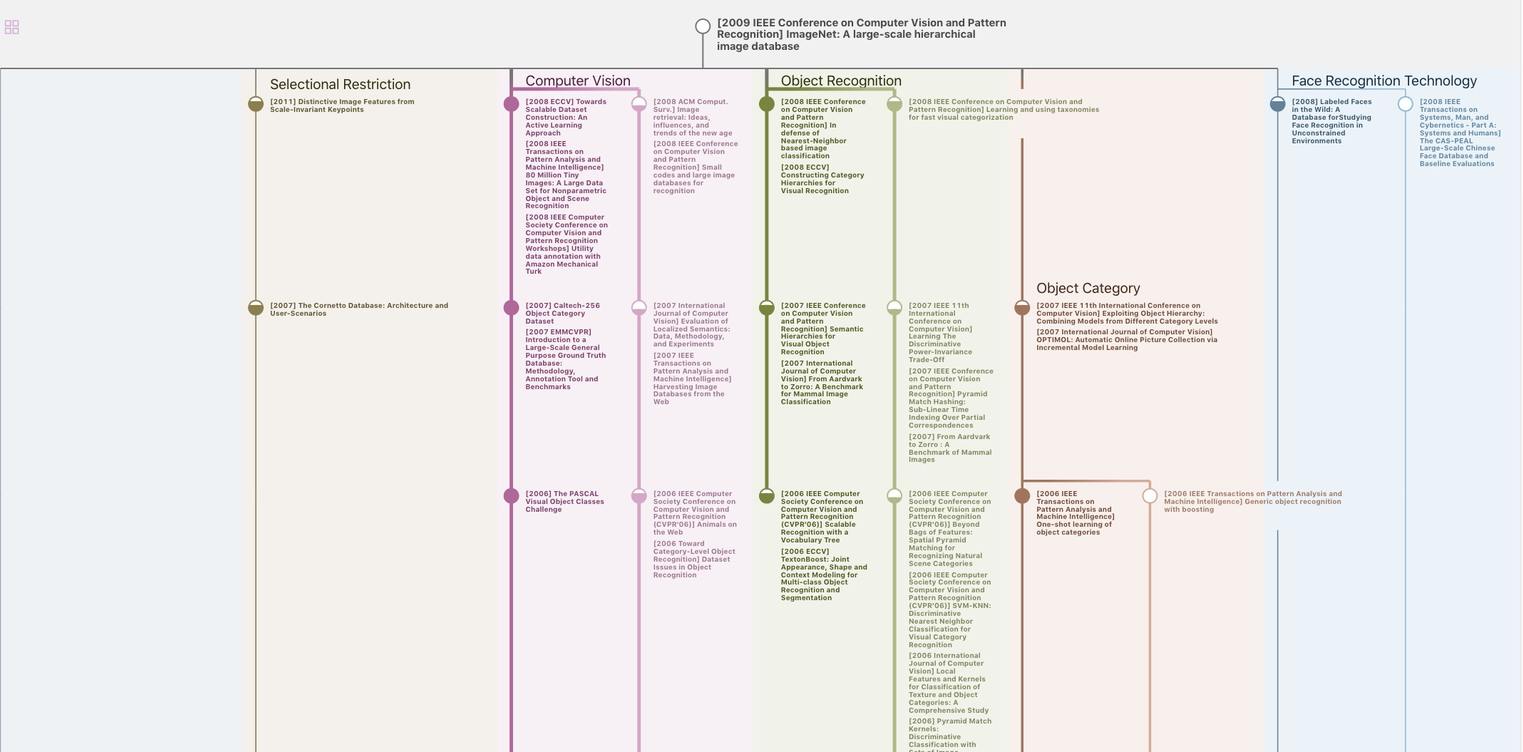
生成溯源树,研究论文发展脉络
Chat Paper
正在生成论文摘要