Multi-level Fusion of Multi-modal Semantic Embeddings for Zero Shot Learning
Multimodal Interfaces and Machine Learning for Multimodal Interaction(2022)
Abstract
ABSTRACT Zero shot learning aims to recognize objects whose instances may not be covered by the training data. To generalize knowledge from seen classes to the novel ones, semantic space is built to embed knowledge from various views into multi-modal semantic embeddings. Existing semantic embeddings neglect the relationships between classes which are essential to transfer knowledge between classes. Moreover, existing zero shot learning models ignore the complementarity between semantic embeddings from different modalities. To tackle these problems, in this work, we resort to graph theory to explicitly model the interdependence between classes and then obtain new modal semantic embeddings. Furthermore, we pioneer to propose a multi-level fusion model to effectively combine knowledge encoded in multi-modal semantic embeddings together. By the virtue of subsequent fusion block, the results of multi-level fusion can be furtherly enriched and fused. Experiments show that our model could achieve promising results on various datasets. Ablation study suggests that our method is well suited for zero shot learning.
MoreTranslated text
Key words
zero shot learning, semantic embeddings, multi-level fusion
AI Read Science
Must-Reading Tree
Example
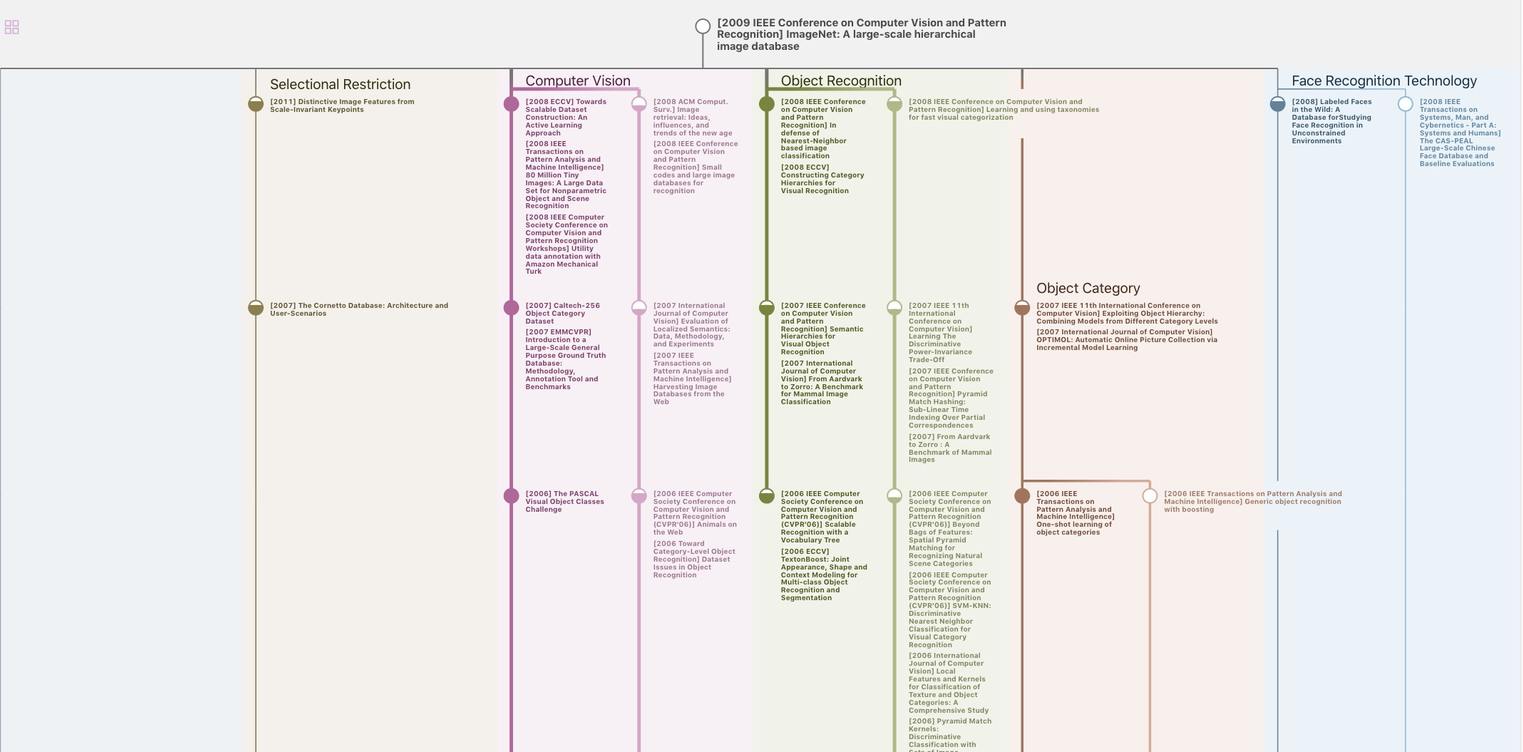
Generate MRT to find the research sequence of this paper
Chat Paper
Summary is being generated by the instructions you defined