MASR: A Model-Agnostic Sparse Routing Architecture for Arbitrary Order Feature Sharing in Multi-Task Learning
Conference on Information and Knowledge Management(2022)
摘要
ABSTRACTMulti-task learning (MTL) has experienced rapid growth in recent years. A typical way of conducting MTL with deep neural networks (DNNs) is either establishing a sort of global feature sharing mechanism across all tasks or assigning each task an individual set of parameters with cross-connections. However, these existing approaches leverage DNNs only to share features of a certain order. Several modelsdemonstrated that explicitly modeling feature sharing with both low-order and high-order features can boost performance. To this end, we propose a model-agnostic sparse routing architecture called MASR, which emphasizes arbitrary order feature sharing for multi-task learning. It is able to choose specific orders of features to route for a given task through learnable latent variables. Moreover, MASR is model-agnostic and can be combined with existing MTL models to share features of both low-order and high-order. Extensive experimental results on several real-world datasets not only confirm the significant improvement of MASR performed to existing MTL models but also outperform existing hybrid architectures in terms of AUC metric.
更多查看译文
关键词
multi-Task learning, sparse routing, model-agnostic
AI 理解论文
溯源树
样例
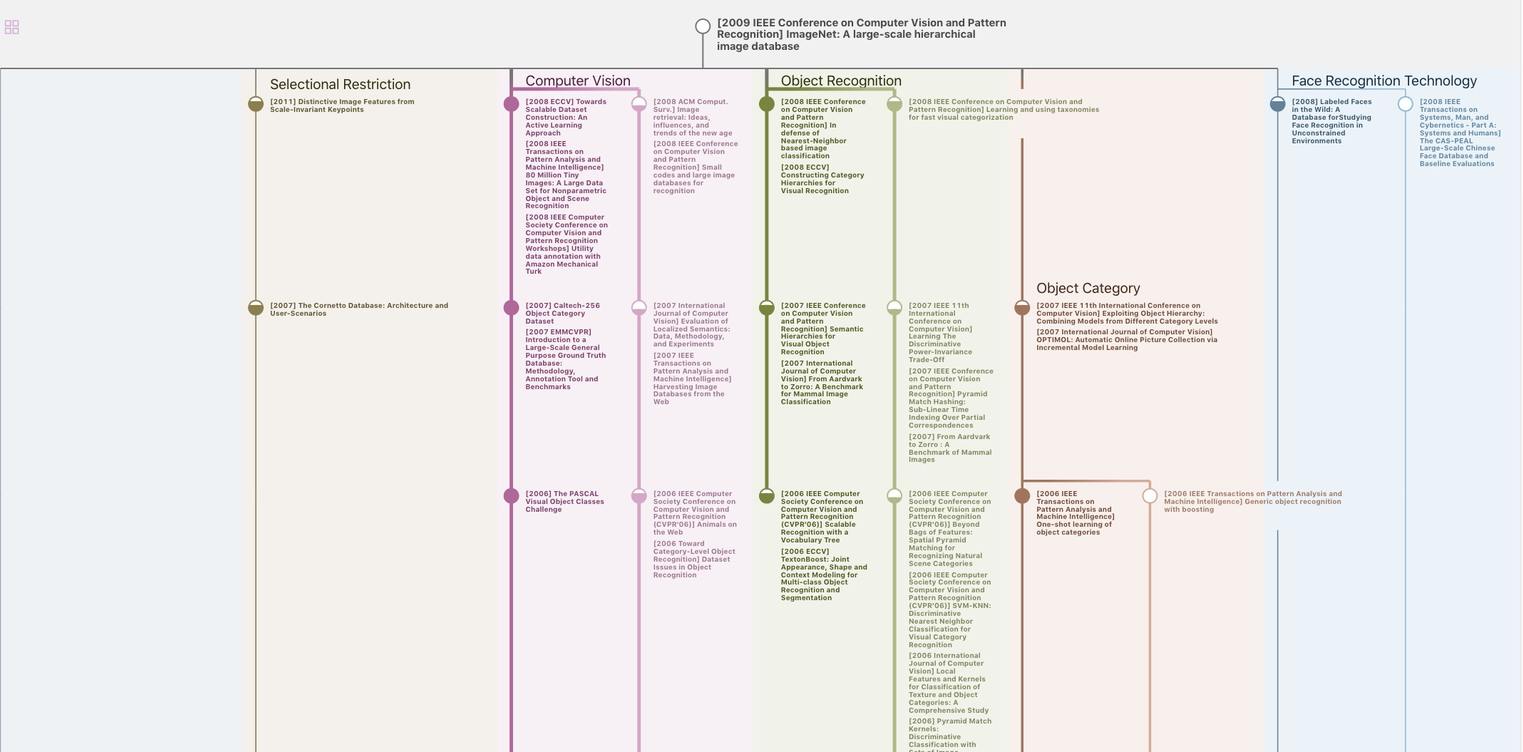
生成溯源树,研究论文发展脉络
Chat Paper
正在生成论文摘要