End-to-end Multi-task Learning Framework for Spatio-Temporal Grounding in Video Corpus
Conference on Information and Knowledge Management(2022)
摘要
ABSTRACTIn this paper, we consider a novel task, Video Corpus Spatio-Temporal Grounding (VCSTG) for material selection and spatio-temporal adaption in intelligent video editing. Given a text query depicting an object and a corpus of untrimmed and unsegmented videos, VCSTG aims to localize a sequence of spatio-temporal object tubes from the video corpus. Existing methods tackle the VCSTG task in a multi-stage approach, which encodes the query and video representation independently for each task, leading to local optimum. In this paper, we propose a novel one-stage multi-task learning based framework named MTSTG for the VCSTG task. MTSTG learns unified query and video representation for video retrieval, temporal grounding and spatial grounding tasks. Video-level, frame-level and object-level contrastive learning are introduced to measure the mutual information between query and video at different granularity. Comprehensive experiments demonstrate our newly proposed framework outperforms the state-of-the-art multi-stage methods on VidSTG dataset.
更多查看译文
关键词
Video Retrieval, Temporal Grounding, Spatial Grounding, Video Corpus Moment Retrieval
AI 理解论文
溯源树
样例
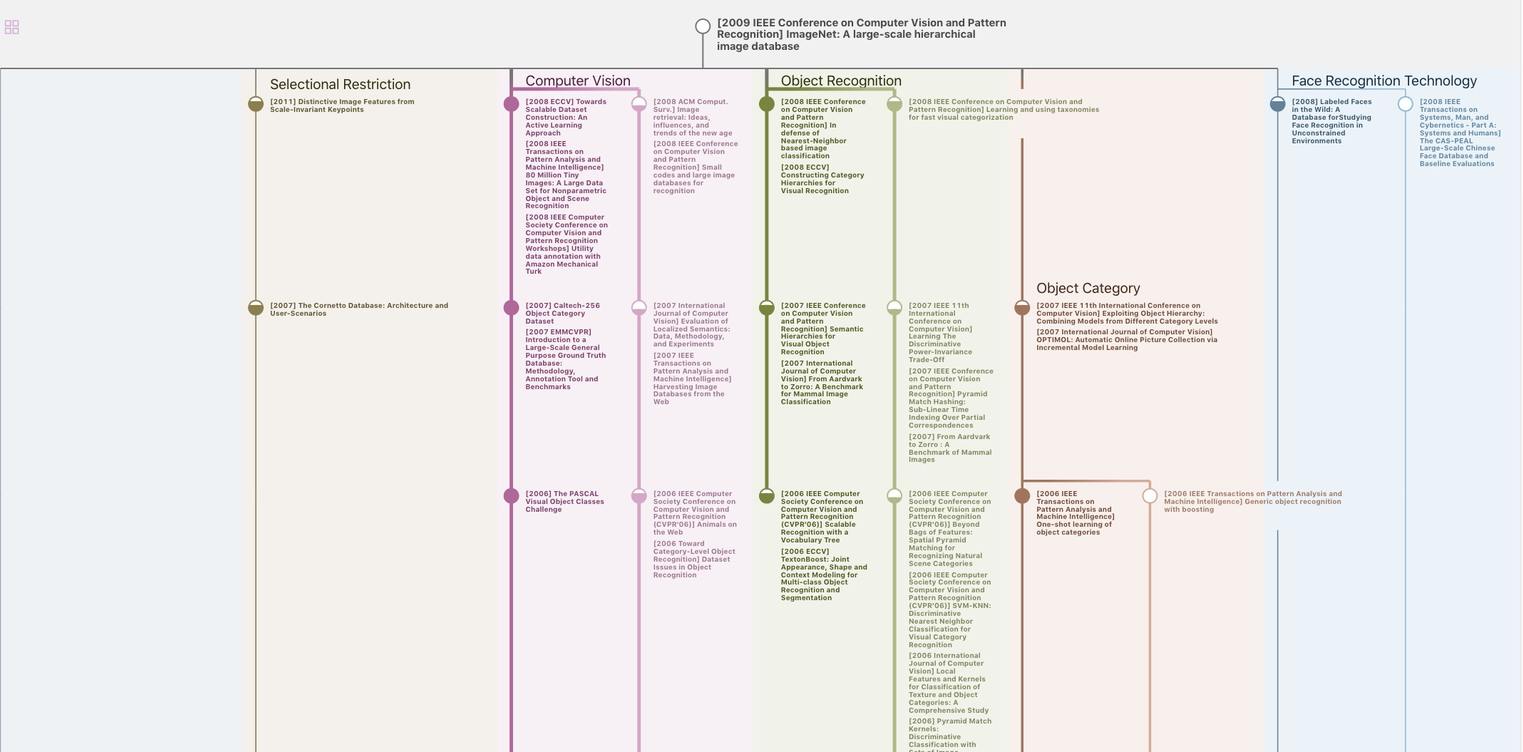
生成溯源树,研究论文发展脉络
Chat Paper
正在生成论文摘要