Text-to-Text Multi-Task Learning to Generate Battlefield Situation Awareness Report
Journal of the Korean Institute of Industrial Engineers(2022)
摘要
Advances in new communication technologies enable commanders to collect various information in battlefield situations. However, it is difficult to make quick and accurate decisions on the battlefield because of vast amount of information. To address this problem, several studies attempt to change tabular data into an easy-to-understand text format. Existing table-to-text studies are not suitable for battlefield situations because they use specific domain data such as WIKIBIO and WIKITABLETEXT. In this study, we propose a table-to-text transfer transformer (TaT4) that uses special tokens to transform log table data into a single sequence to preserve table information. Moreover, the proposed TaT4 uses multi-task learning that can leverage cross-task data of types in a single model to improve generalization performance. We conduct experiments on eight datasets generated from three Korean defense modeling and simulations (M&S) of battlefield situations in the Army, Air Force, and Navy. The proposed TaT4 outperforms the existing table-to-text models.
更多查看译文
关键词
awareness,learning,text-to-text,multi-task
AI 理解论文
溯源树
样例
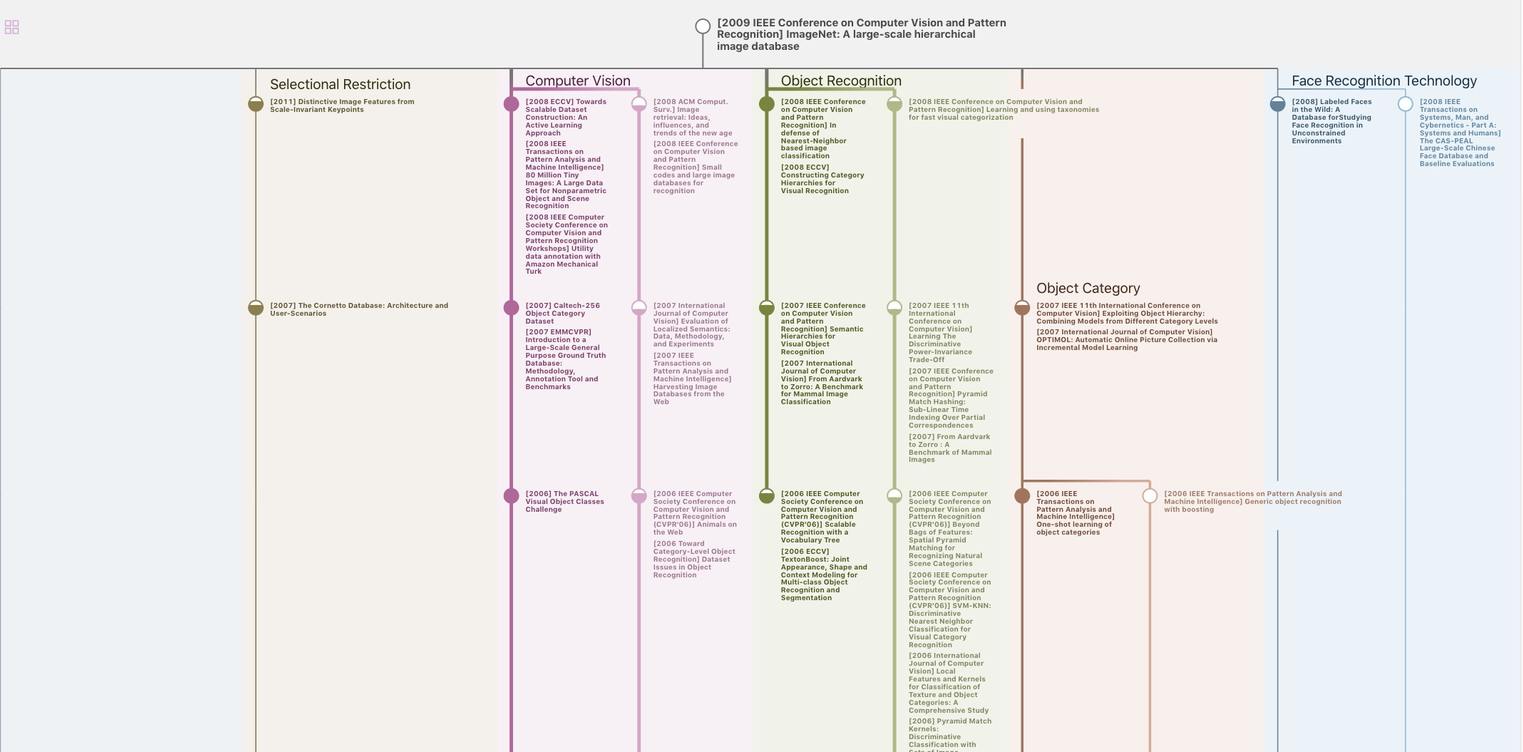
生成溯源树,研究论文发展脉络
Chat Paper
正在生成论文摘要