LOCKS: User Differentially Private and Federated Optimal Client Sampling
arxiv(2022)
摘要
With changes in privacy laws, there is often a hard requirement for client data to remain on the device rather than being sent to the server. Therefore, most processing happens on the device, and only an altered element is sent to the server. Such mechanisms are developed by leveraging differential privacy and federated learning. Differential privacy adds noise to the client outputs and thus deteriorates the quality of each iteration. This distributed setting adds a layer of complexity and additional communication and performance overhead. These costs are additive per round, so we need to reduce the number of iterations. In this work, we provide an analytical framework for studying the convergence guarantees of gradient-based distributed algorithms. We show that our private algorithm minimizes the expected gradient variance by approximately $d^2$ rounds, where d is the dimensionality of the model. We discuss and suggest novel ways to improve the convergence rate to minimize the overhead using Importance Sampling (IS) and gradient diversity. Finally, we provide alternative frameworks that might be better suited to exploit client sampling techniques like IS and gradient diversity.
更多查看译文
关键词
federated optimal client sampling
AI 理解论文
溯源树
样例
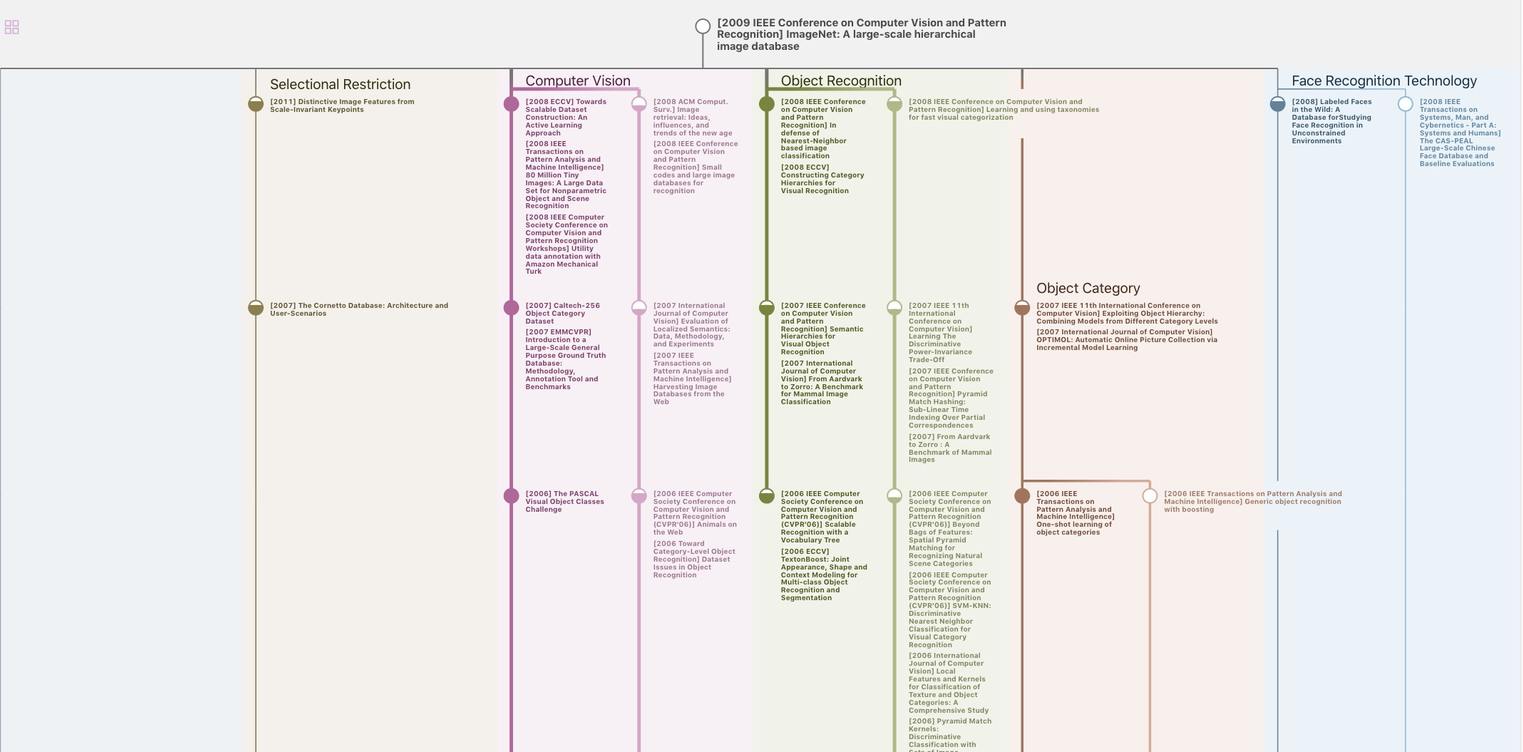
生成溯源树,研究论文发展脉络
Chat Paper
正在生成论文摘要