Rank-LIME: Local Model-Agnostic Feature Attribution for Learning to Rank
PROCEEDINGS OF THE 2023 ACM SIGIR INTERNATIONAL CONFERENCE ON THE THEORY OF INFORMATION RETRIEVAL, ICTIR 2023(2023)
摘要
Understanding why a model makes certain predictions is crucial when adapting it for real world decision making. LIME is a popular model-agnostic feature attribution method for the tasks of classification and regression. However, the task of learning to rank in information retrieval is more complex in comparison with either classification or regression. In this work, we extend LIME to propose Rank-LIME, a model-agnostic, local, post-hoc linear feature attribution method for the task of learning to rank that generates explanations for ranked lists. We employ novel correlation-based perturbations, differentiable ranking loss functions and introduce new metrics to evaluate ranking based additive feature attribution models. We compare Rank-LIME with a variety of competing systems, with models trained on the MS MARCO datasets and observe that Rank-LIME outperforms existing explanation algorithms in terms of Model Fidelity and Explain-NDCG. With this we propose one of the first algorithms to generate additive feature attributions for explaining ranked lists.
更多查看译文
关键词
ranking interpretability,post-hoc explanations,feature attribution methods,learning to rank,LIME
AI 理解论文
溯源树
样例
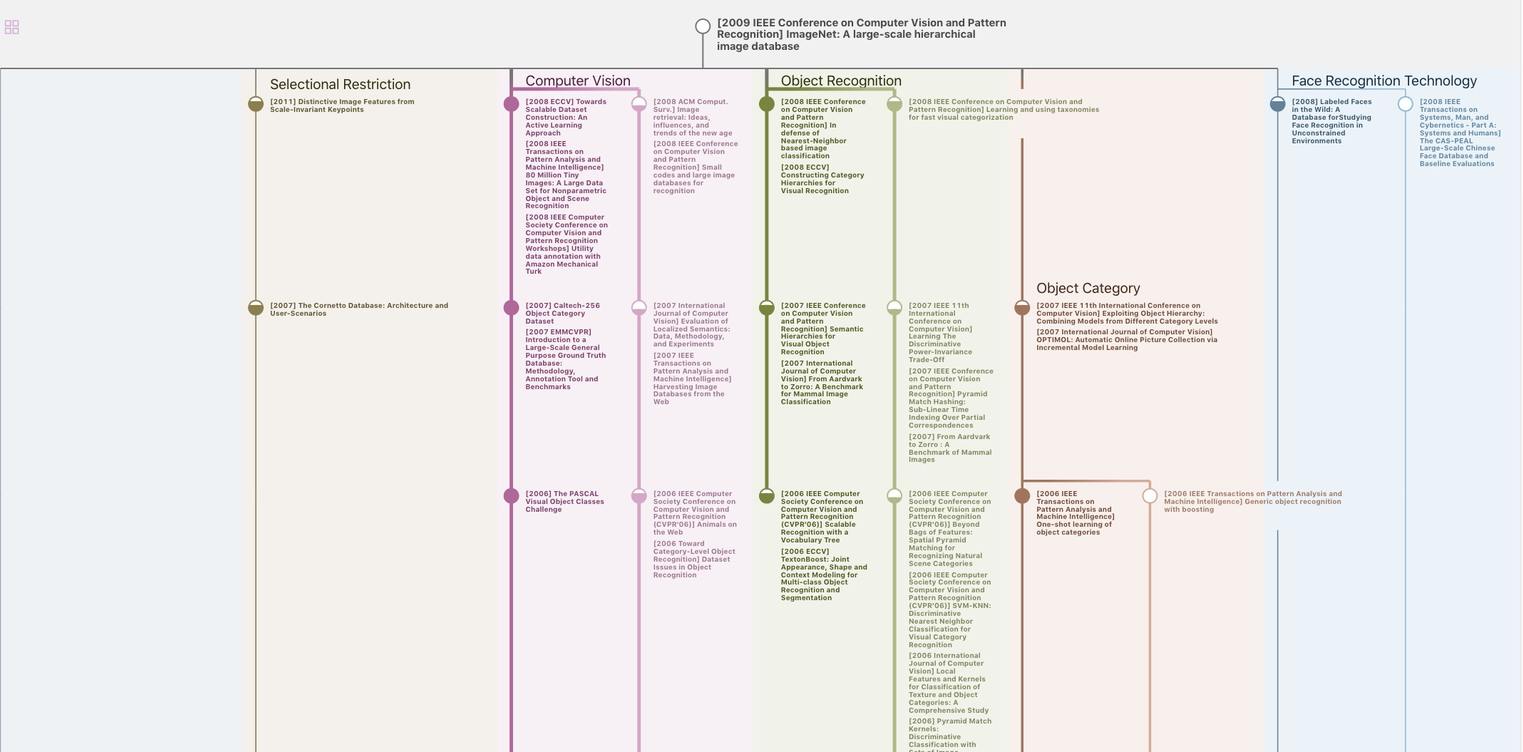
生成溯源树,研究论文发展脉络
Chat Paper
正在生成论文摘要