Independent Relation Representation With Line Graph for Cross-Lingual Entity Alignment
IEEE Transactions on Knowledge and Data Engineering(2023)
摘要
Cross-lingual entity alignment, which is an important task in the field of graph mining, aims to find equivalent entity pairs from two knowledge graphs. Recent methods show that relation representation can be used to improve entity representation and entity alignment. However, relation representation is learned dependently on entity representation, and both representations are learned from node-centered knowledge graphs. This dependency of relation on entity results in poor relation representation and leads to limited enhancement of entity representation. Therefore, to address this challenge, we propose novel relation-aware line graph neural networks for cross-lingual entity alignment (RALG). More specifically, we first propose to learn relation representation with heterogeneous line graphs independently from entities. The constructed heterogeneous line graphs can capture the correlation of relations explicitly. Second, we design a new way of aggregation in the form of triples to strengthen the relevance between entities and their corresponding relations. Experiments conducted on real-world datasets show that independent learning of relation representation with line graphs can represent relations better, and our method achieves better performance than the state-of-the-art methods for entity alignment.
更多查看译文
关键词
Cross-lingual,entity alignment,knowledge graph,line graph
AI 理解论文
溯源树
样例
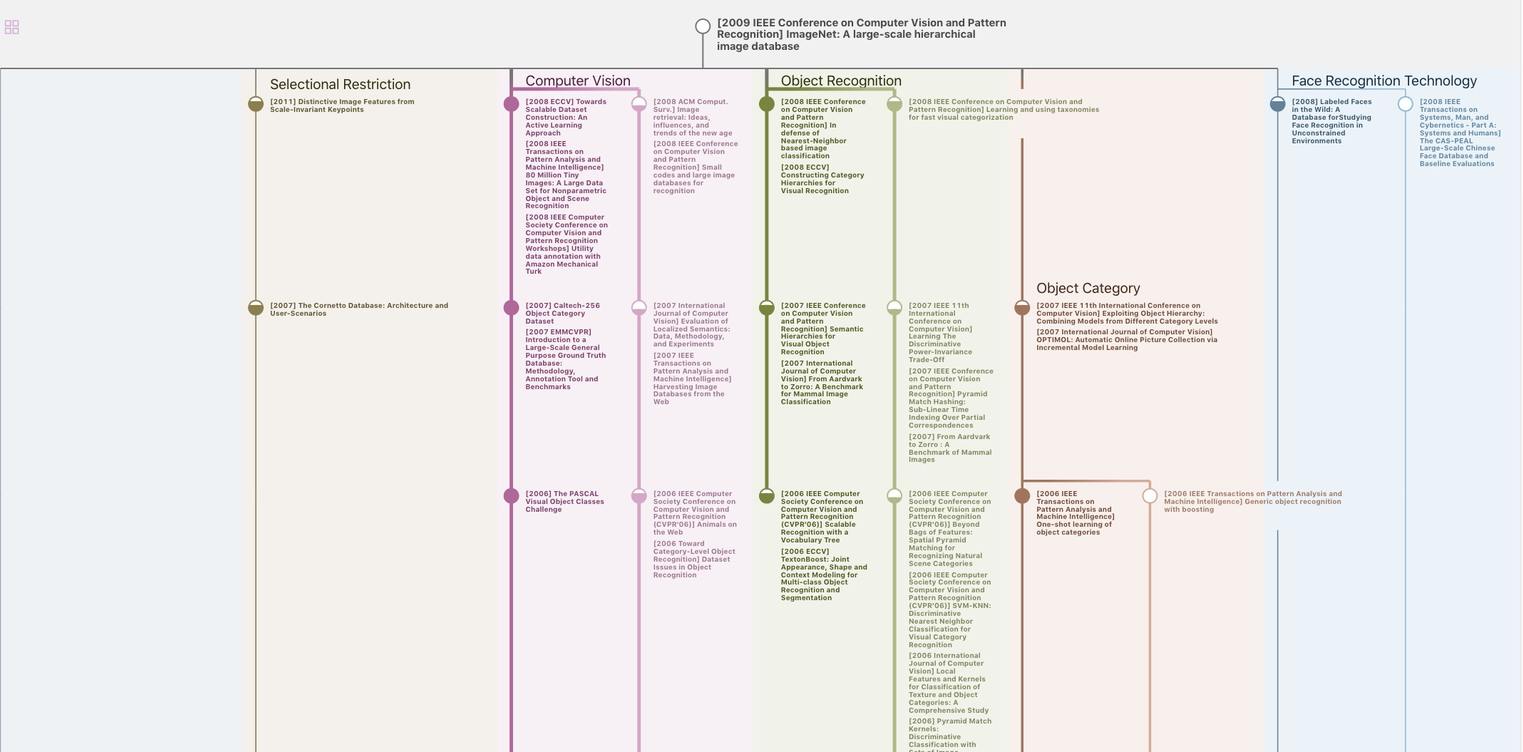
生成溯源树,研究论文发展脉络
Chat Paper
正在生成论文摘要