Multi-task Transfer Learning Facilitated by Segmentation and Denoising for Anomaly Detection of Rail Fasteners
Journal of Electrical Engineering & Technology(2022)
摘要
The rail fastener is the main component of rail tracks that contributes to safe train travel by fixing the rail to railroad ties. Anomalies such as the absence or damage should be checked regularly as they can lead to large-scale accidents. Image-based maintenance systems have been proposed as efficient management, but it is still difficult to detect anomalies due to occlusion by obstacles or noise in the image. Therefore, we propose a deep anomaly detection system for rail fasteners using multi-task transfer learning. First, the U-Net model is trained for an auxiliary task consisting of segmentation and denoising. Second, in the transfer learning process, a machine learning or deep learning-based classifier detects anomalies using the feature map obtained from the trained U-Net encoder. The proposed model is rigorously evaluated with our collected data. The experimental results show that the deep learning-based classifier detected anomalies with an accuracy of about 97.57%, and multi-task transfer learning contributes to the model focusing on the fastener region in the image. It suggests the potential of image-based automatic defect detection systems for many industrial applications.
更多查看译文
关键词
Anomaly detection,Railway fastener,Multi-task transfer learning,Segmentation,Denoising
AI 理解论文
溯源树
样例
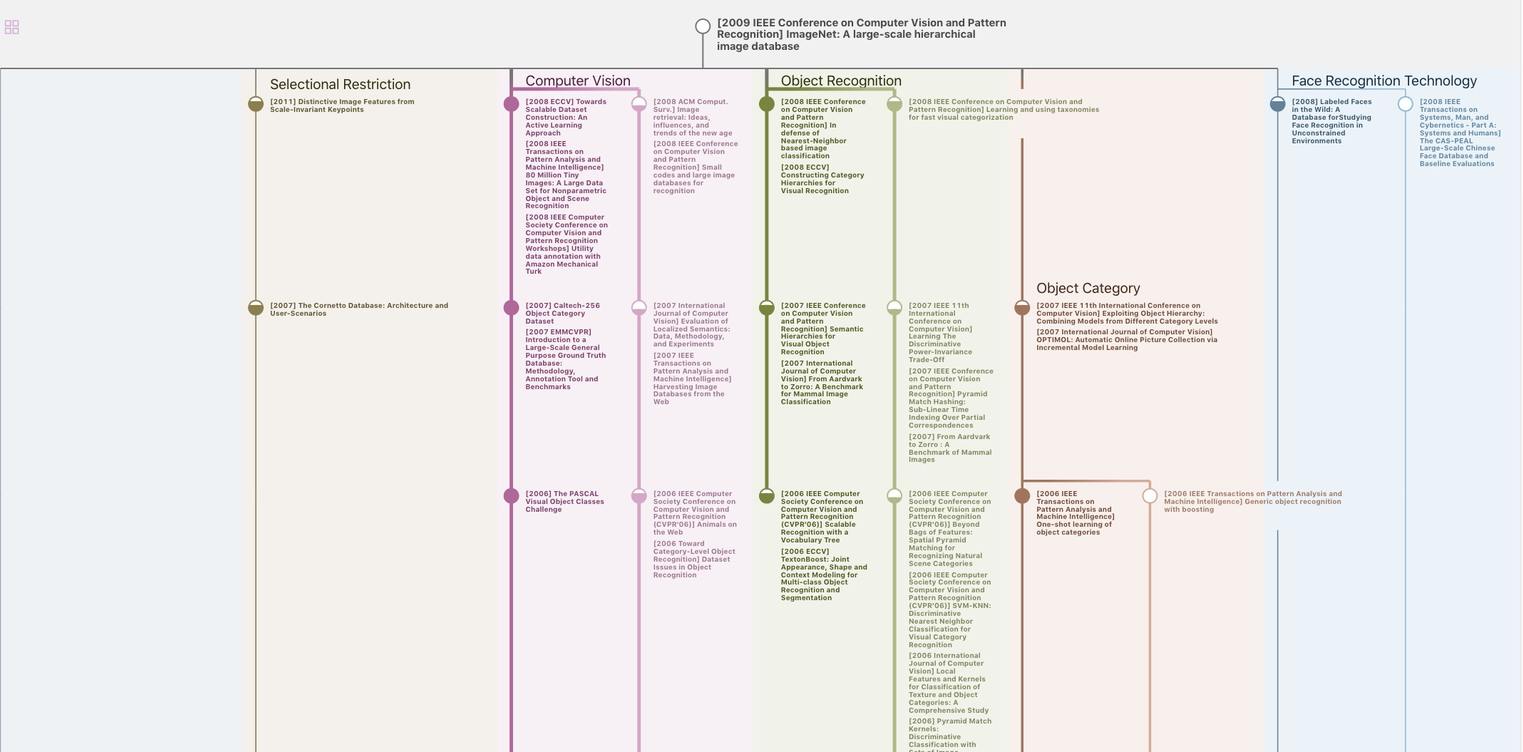
生成溯源树,研究论文发展脉络
Chat Paper
正在生成论文摘要