LDI-MVFNet: A Multi-view fusion deep network for leachate distribution imaging.
Waste management (New York, N.Y.)(2022)
摘要
The accurate monitoring and early warning of groundwater pollution caused by the concealed leakage of landfills is a major challenge globally in the field of solid waste management and groundwater protection. Electrical resistivity tomography (ERT) represents a potential solution with advantages, owing to its fast and nondestructive characteristics. However, traditional ERT based on a single array cannot reveal the distribution and dynamics of pollution in complex underground media owing to the limited information it carries. We designed a novel deep network for multi-view fusion to invert the real resistivity distribution of the medium caused by leachate (named LDI-MVFNet) so as to infer the distribution of leachate. To support model establishment and validation, ERT instances collected from synthetic models and a salt tracer experiment were inverted. We compared the inversion results of the LDI-MVFNet with those of single arrays and found that the LDI-MVFNet performed the best overall. The average root mean square error (RMSE) of synthetic models reached 0.98, performing better than Dipole-Dipole (3.86), Wenner-Schlumberger (3.37), and Pole-Pole (6.61), which were inverted separately. The resultant inverted subsurface true resistivity data were presented in the form of two-dimensional (2D) cross sections. The imaging results of 2D cross sections showed that LDI-MVFNet was superior to others in data noise suppression and inversion accuracy. The results of this study indicate that the data fusion of multiple views can more accurately reflect the real resistivity than the inversion of a single array can.
更多查看译文
AI 理解论文
溯源树
样例
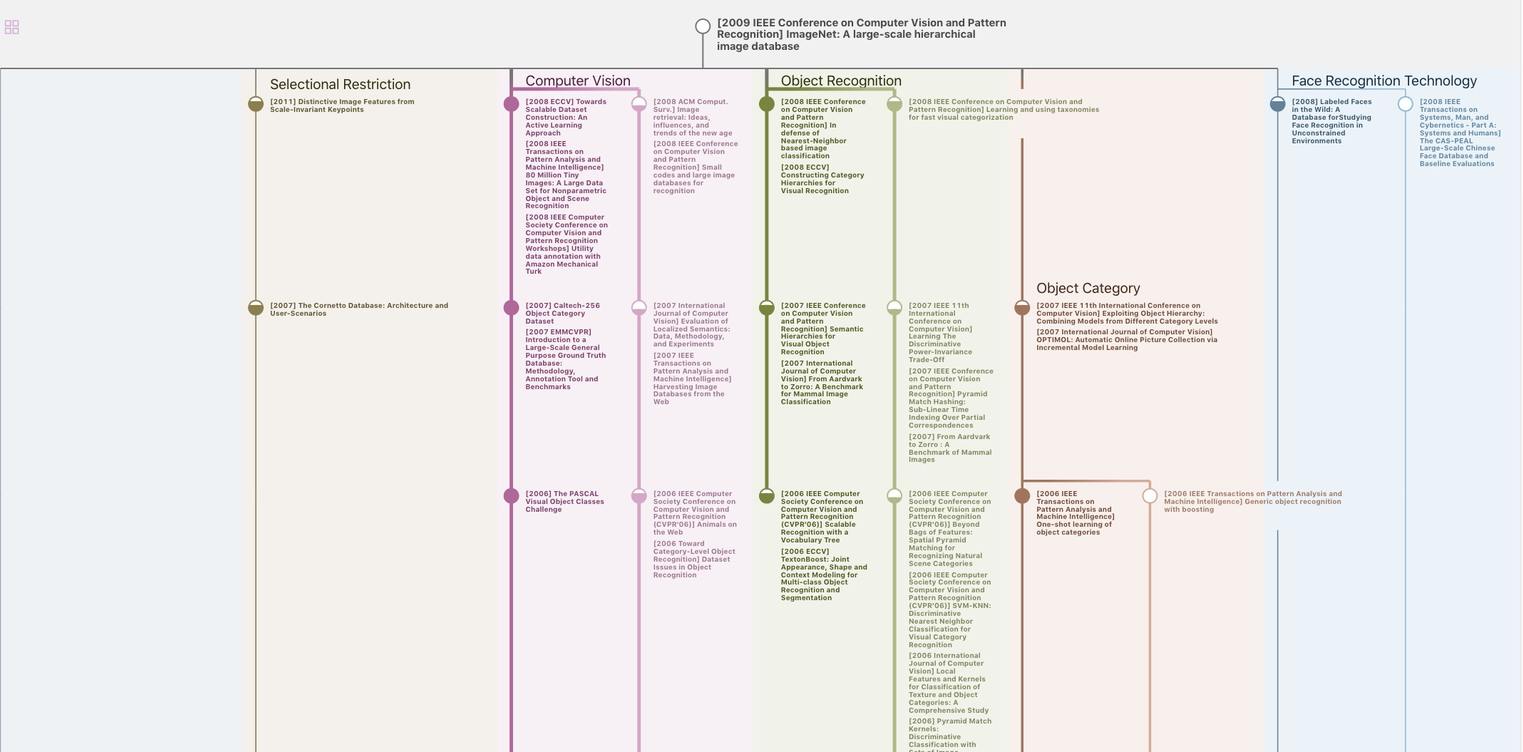
生成溯源树,研究论文发展脉络
Chat Paper
正在生成论文摘要