Non-Iterative Constrained Compressive Beamforming
SSRN Electronic Journal(2022)
摘要
Compressive beamforming is increasingly researched and employed in underwater acoustic applications. Sparse Bayesian learning (SBL) induces the prior distribution and encourages the sparsity of the source vector, achieving improved accuracy compared with other deterministic compressive beamforming approaches, where the constraint optimization is solved explicitly. Considering the structural complexity of targets and underwater environment, it benefits the reconstruction performance to employ the multiconstraint SBL (MSBL) approach, exploring the sparsity in a joint sparse representation domain. However, the computation load of MSBL is heavy and unpredictable due to the requisite iterative implementation, and regular acceleration approaches are difficult to employ. To overcome such obstacles, an online constrained SBL is proposed, which is non-iterative and computationally efficient. Moreover, the estimation convergence of hyperparameters is proved and guaranteed theoretically. Through empirical and experimental results, the efficacy of the proposed method is validated and compared with other state-of-the-art beamforming approaches.
更多查看译文
关键词
Sparse Bayesian learning, Compressive beamforming, Multiconstraint, Sawtooth lag, Kalman filtering
AI 理解论文
溯源树
样例
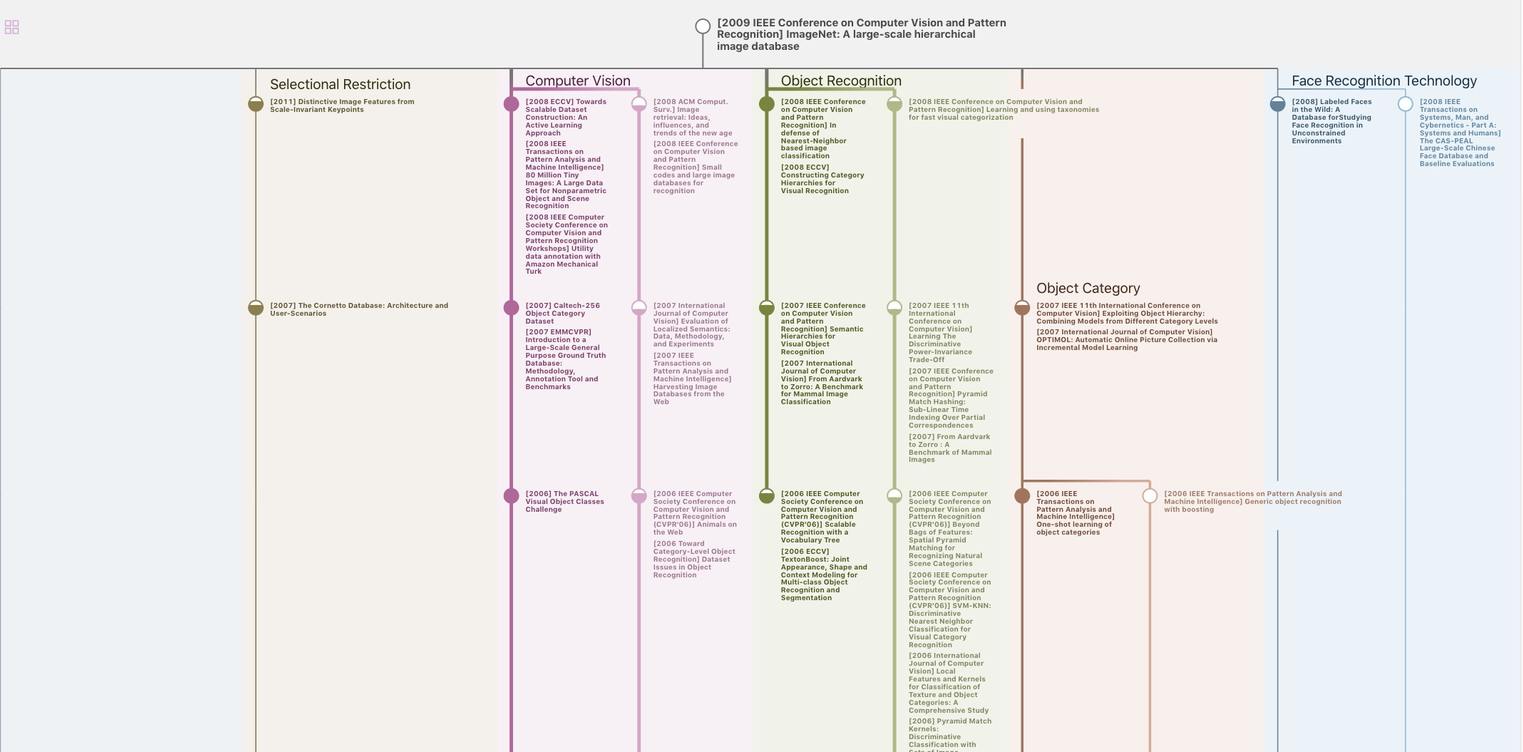
生成溯源树,研究论文发展脉络
Chat Paper
正在生成论文摘要