An Automatic Segmentation Method for Lung Tumor Based on Improved Region Growing Algorithm.
Diagnostics (Basel, Switzerland)(2022)
摘要
In medical image processing, accurate segmentation of lung tumors is very important. Computer-aided accurate segmentation can effectively assist doctors in surgery planning and treatment decisions. Although the accurate segmentation results of lung tumors can provide a reliable basis for clinical treatment, the key to obtaining accurate segmentation results is how to improve the segmentation performance of the algorithm. We propose an automatic segmentation method for lung tumors based on an improved region growing algorithm, which uses the prior information on lung tumors to achieve an automatic selection of the initial seed point. The proposed method includes a seed point expansion mechanism and an automatic threshold update mechanism and takes the combination of multiple segmentation results as the final segmentation result. In the lung image database consortium (LIDC-IDRI) dataset, we designed 10 experiments to test the proposed method and compare it with 4 popular segmentation methods. The experimental results show that the average dice coefficient obtained by the proposed method is 0.936 ± 0.027, and the average Jaccard distance is 0.114 ± 0.049. The average dice coefficient obtained by the proposed method is 0.107, 0.053, 0.040, and 0.156, higher than that of the other four methods, respectively. This study proves that the proposed method can automatically segment lung tumors in CT slices and has suitable segmentation performance.
更多查看译文
关键词
automatically update thresholds,growth restriction conditions,improved region growing algorithm,lung tumor segmentation
AI 理解论文
溯源树
样例
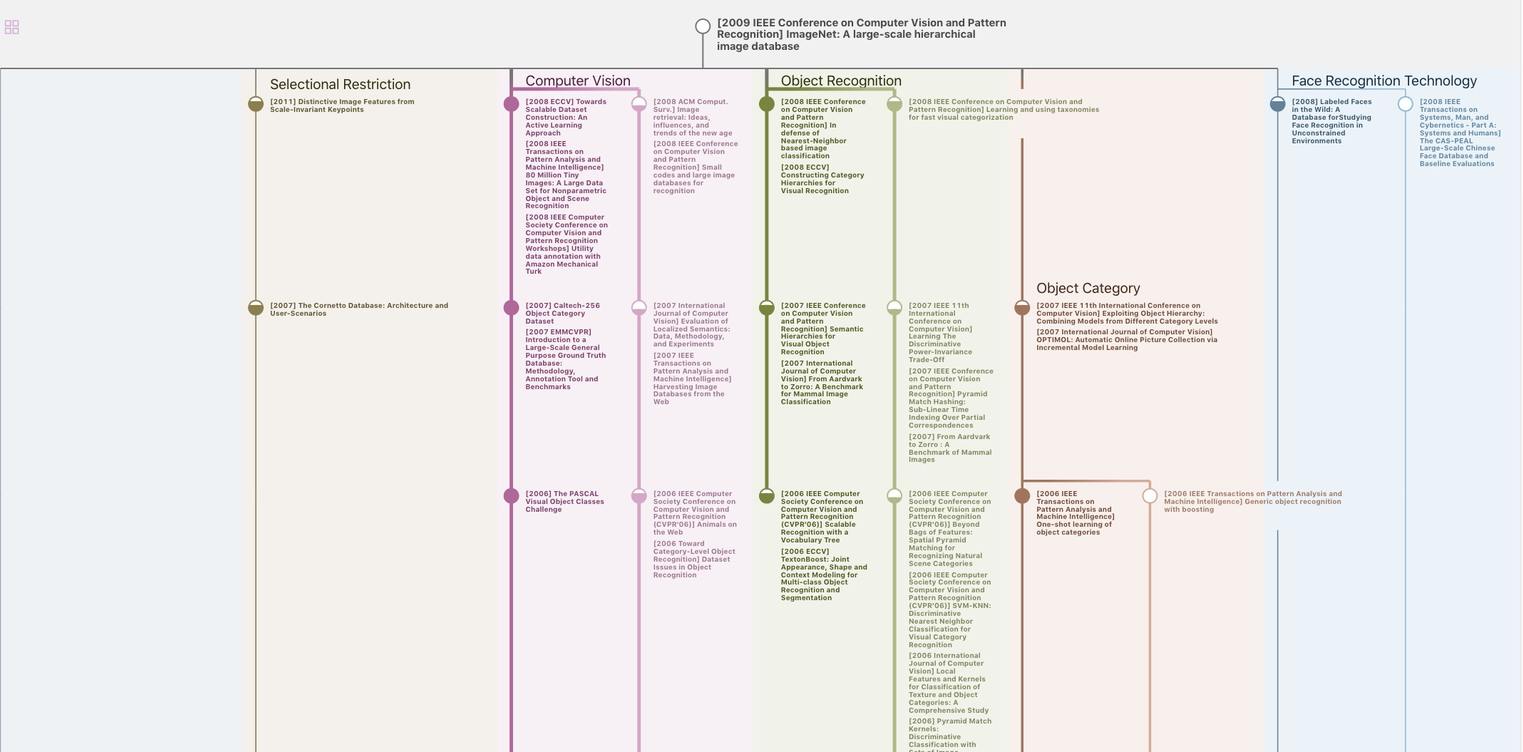
生成溯源树,研究论文发展脉络
Chat Paper
正在生成论文摘要