Artificial Intelligence-based Detection of Epileptic Discharges from Pediatric Scalp Electroencephalograms: A Pilot Study.
Acta medica Okayama(2022)
摘要
We developed an artificial intelligence (AI) technique to identify epileptic discharges (spikes) in pediatric scalp electroencephalograms (EEGs). We built a convolutional neural network (CNN) model to automatically classify steep potential images into spikes and background activity. For the CNN model' training and validation, we examined 100 children with spikes in EEGs and another 100 without spikes. A different group of 20 children with spikes and 20 without spikes were the actual test subjects. All subjects were ≥ 3 to < 18 years old. The accuracy, sensitivity, and specificity of the analysis were >0.97 when referential and combination EEG montages were used, and < 0.97 with a bipolar montage. The correct classification of background activity in individual patients was significantly better with a referential montage than with a bipolar montage (p=0.0107). Receiver operating characteristic curves yielded an area under the curve > 0.99, indicating high performance of the classification method. EEG patterns that interfered with correct classification included vertex sharp transients, sleep spindles, alpha rhythm, and low-amplitude ill-formed spikes in a run. Our results demonstrate that AI is a promising tool for automatically interpreting pediatric EEGs. Some avenues for improving the technique were also indicated by our findings.
更多查看译文
关键词
children,deep learning,electroencephalogram,neural network,spike
AI 理解论文
溯源树
样例
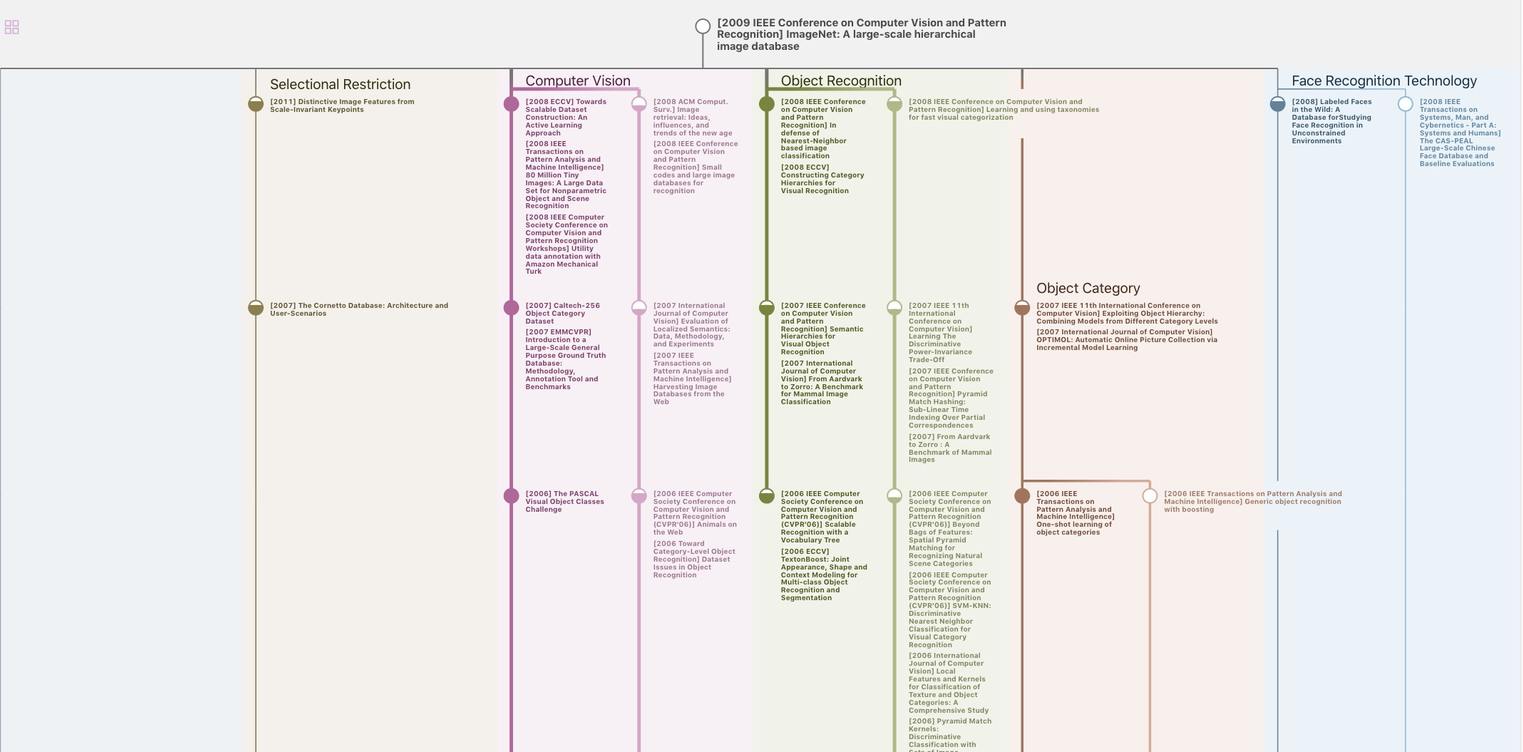
生成溯源树,研究论文发展脉络
Chat Paper
正在生成论文摘要