PD19 Machine Learning Modelling For Clinical Trial Design Using The National Institute for Health and Care Research Innovation Observatory’s ScanMedicine Database
International Journal of Technology Assessment in Health Care(2022)
摘要
IntroductionClinical trials that fail prematurely due to poor design are a waste of resources and deprives us of data for evaluating potentially effective interventions. This study used machine learning modelling to predict clinical trials’ success or failure and to understand feature contributions driving this result. Features to power the modelling were engineered using data collected from the National Institute for Health and Care Research Innovation Observatory’s ScanMedicine database.MethodsUsing ScanMedicine, a large dataset containing 641,079 clinical trial records from 11 global clinical trial registries, was extracted. Sixteen features were generated from the data based on fields relating to trial design and eligibility. Trials were labeled positive if they were completed (or target recruitment was achieved) or negative if terminated/withdrawn (or target recruitment was not achieved). To achieve optimal performance, phase-specific datasets were generated, and we focused on a subsample of Phase 2 trials (n=70,167). Ensemble models using bagging and boosting algorithms, including balanced random forest and extreme gradient boosting classifiers were used for training and evaluating predictive performance. Shapley Additive Explanations was used to explain the output of the best performing model and calculate feature contributions for individual studies.ResultsWe achieved a weighted F1-score of 0.88, Receiver Operator Characteristic Area under the Curve score of 0.75, and balanced accuracy of 0.75 on the test set with the xgBoost model. This result shows that the model can successfully distinguish between classes to predict if a trial will succeed or fail and subsequently output the features driving this outcome. The number of primary outcomes, whether the study was randomized, target sample size and number of exclusion criteria were the most important features affecting the model’s prediction.ConclusionsThis study is the first to use predictive modelling on a large sample of clinical trial data obtained from 11 international trial registries. The prediction outcomes achieved by our novel approach, which uses phase-specific trained models, outperforms previous modelling in this space.
更多查看译文
关键词
clinical trial design,clinical trial,care research innovation observatorys,modelling
AI 理解论文
溯源树
样例
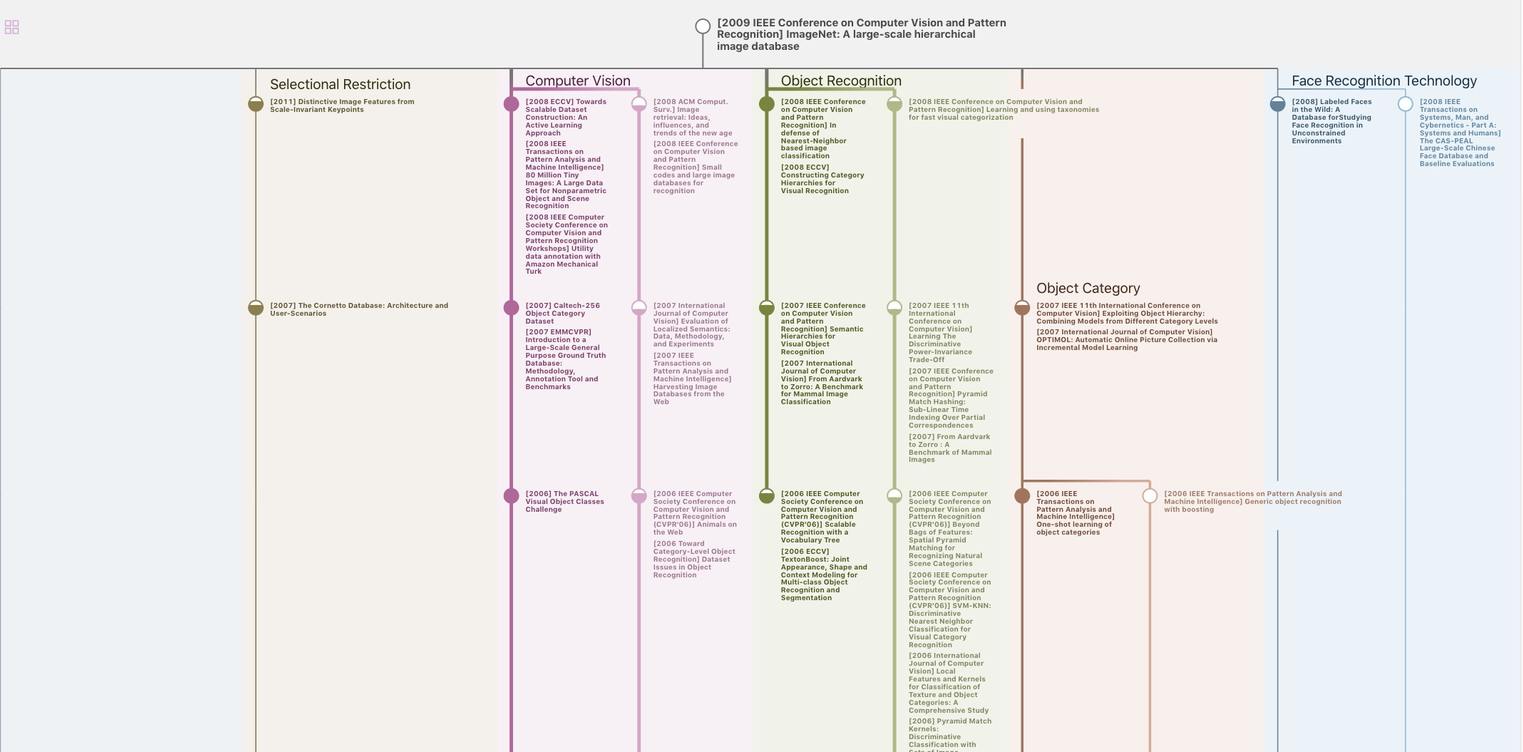
生成溯源树,研究论文发展脉络
Chat Paper
正在生成论文摘要