Machine learning based models for high-throughput classification of human pregnane X receptor activators
ENVIRONMENTAL SCIENCE-ADVANCES(2023)
摘要
The pregnane X receptor (PXR) is a master receptor in regulating the metabolism and transport of structurally diverse endogenous compounds. Activation of PXR by xenobiotics potentially induces adverse effects and disrupts normal physiological states. Therefore, it is essential to filter out PXR activators despite challenges in the construction of PXR screening models. Herein, we developed a high-throughput model using machine learning to classify human PXR (hPXR) activators and non-activators. Molecular descriptors and eight fingerprints were calculated for a diverse dataset retrieved from the PubChem database. The dimension reduction procedure was adopted to define an optimal subset of fingerprints and 87 molecular descriptors before the model construction. Five machine learning methods coupled with molecular descriptors and fingerprints were compared and the XGBoost method combined with RDKit descriptors yielded the best performance with AUC values of 0.913 and 0.860 for the training set (4144 chemicals) and external test set (1037 chemicals). The model constructed with the XGBoost method has high prediction ability as revealed by the applicability domain analysis. Our built machine learning models are useful for identifying compounds of potential PXR activators and facilitating the prioritization of contaminants of emerging concern. A machine learning-based model was developed for classifying PXR activators with high generalization capability and broad applicability domain.
更多查看译文
关键词
machine learning,classification,receptor,models,high-throughput
AI 理解论文
溯源树
样例
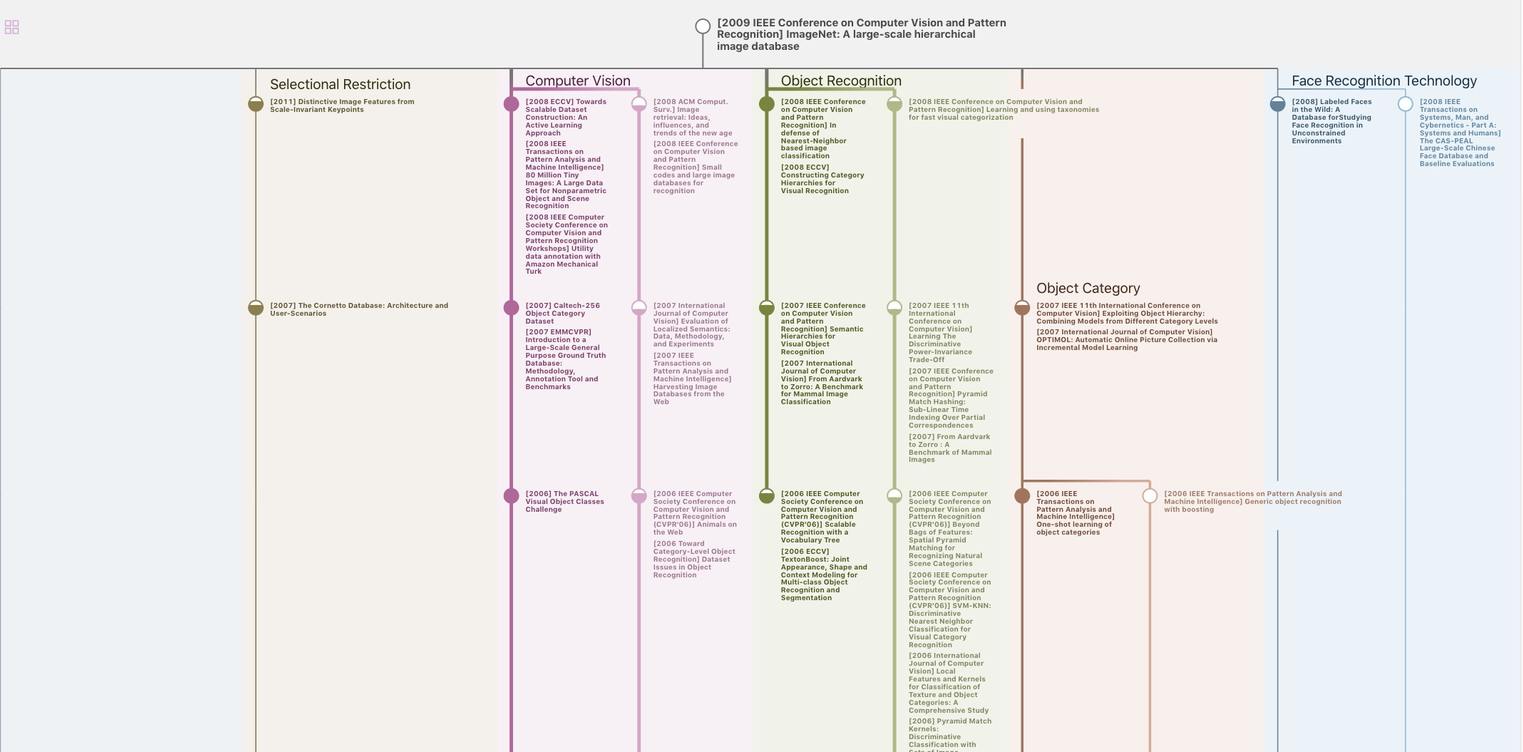
生成溯源树,研究论文发展脉络
Chat Paper
正在生成论文摘要