Adaptive Significance Levels in Tests for Linear Regression Models: The e -Value and P -Value Cases.
Entropy(2022)
摘要
The full Bayesian significance test (FBST) for precise hypotheses is a Bayesian alternative to the traditional significance tests based on -values. The FBST is characterized by the -value as an evidence index in favor of the null hypothesis (). An important practical issue for the implementation of the FBST is to establish how small the evidence against must be in order to decide for its rejection. In this work, we present a method to find a cutoff value for the -value in the FBST by minimizing the linear combination of the averaged type-I and type-II error probabilities for a given sample size and also for a given dimensionality of the parameter space. Furthermore, we compare our methodology with the results obtained from the test with adaptive significance level, which presents the capital-P -value as a decision-making evidence measure. For this purpose, the scenario of linear regression models with unknown variance under the Bayesian approach is considered.
更多查看译文
关键词
Bayesian test,adaptive significance levels,linear regression,predictive distribution,significance test
AI 理解论文
溯源树
样例
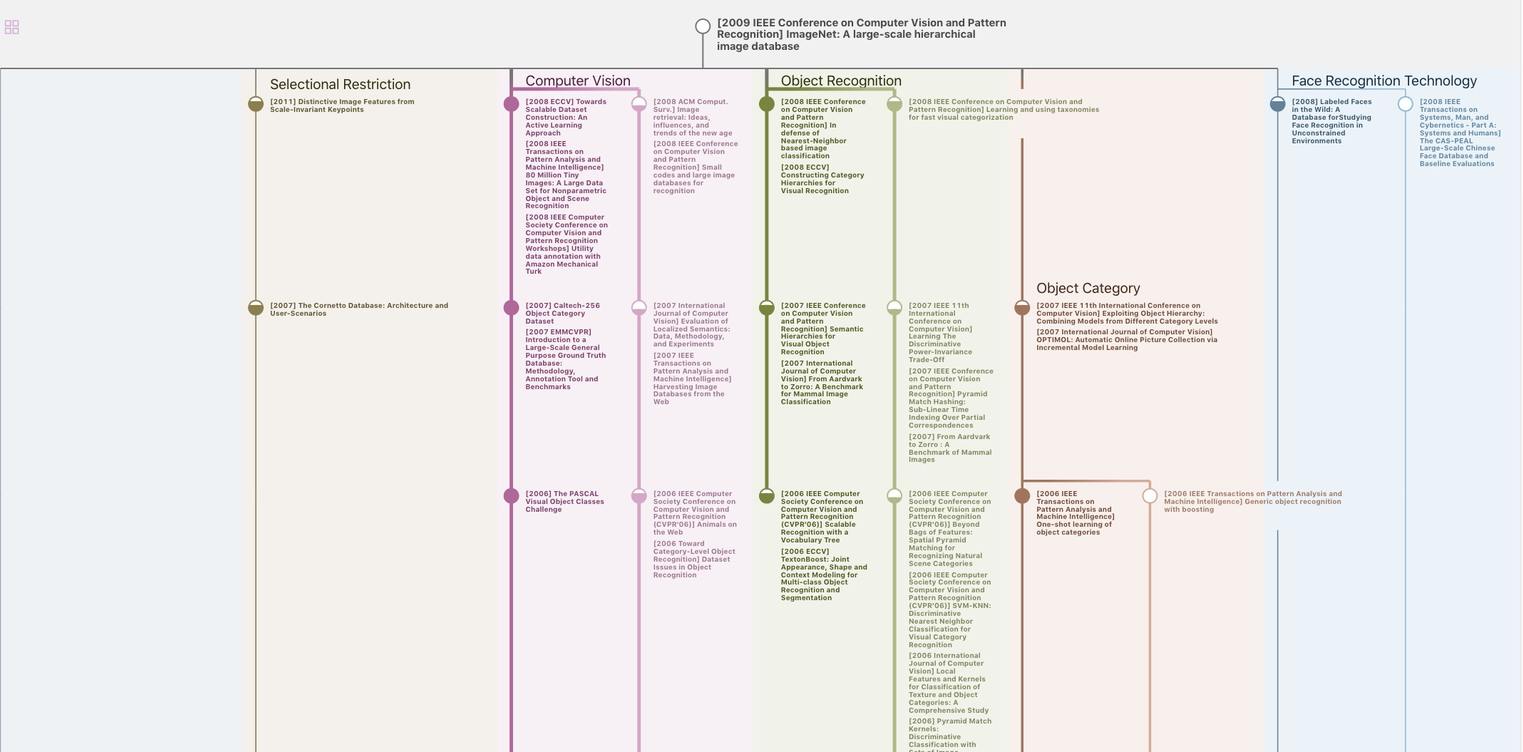
生成溯源树,研究论文发展脉络
Chat Paper
正在生成论文摘要