A deep-learning model for transforming the style of tissue images from cryosectioned to formalin-fixed and paraffin-embedded
Nature Biomedical Engineering(2022)
摘要
Histological artefacts in cryosectioned tissue can hinder rapid diagnostic assessments during surgery. Formalin-fixed and paraffin-embedded (FFPE) tissue provides higher quality slides, but the process for obtaining them is laborious (typically lasting 12–48 h) and hence unsuitable for intra-operative use. Here we report the development and performance of a deep-learning model that improves the quality of cryosectioned whole-slide images by transforming them into the style of whole-slide FFPE tissue within minutes. The model consists of a generative adversarial network incorporating an attention mechanism that rectifies cryosection artefacts and a self-regularization constraint between the cryosectioned and FFPE images for the preservation of clinically relevant features. Transformed FFPE-style images of gliomas and of non-small-cell lung cancers from a dataset independent from that used to train the model improved the rates of accurate tumour subtyping by pathologists.
更多查看译文
关键词
Image processing,Machine learning,Pathology,Biomedicine,general,Biomedical Engineering/Biotechnology
AI 理解论文
溯源树
样例
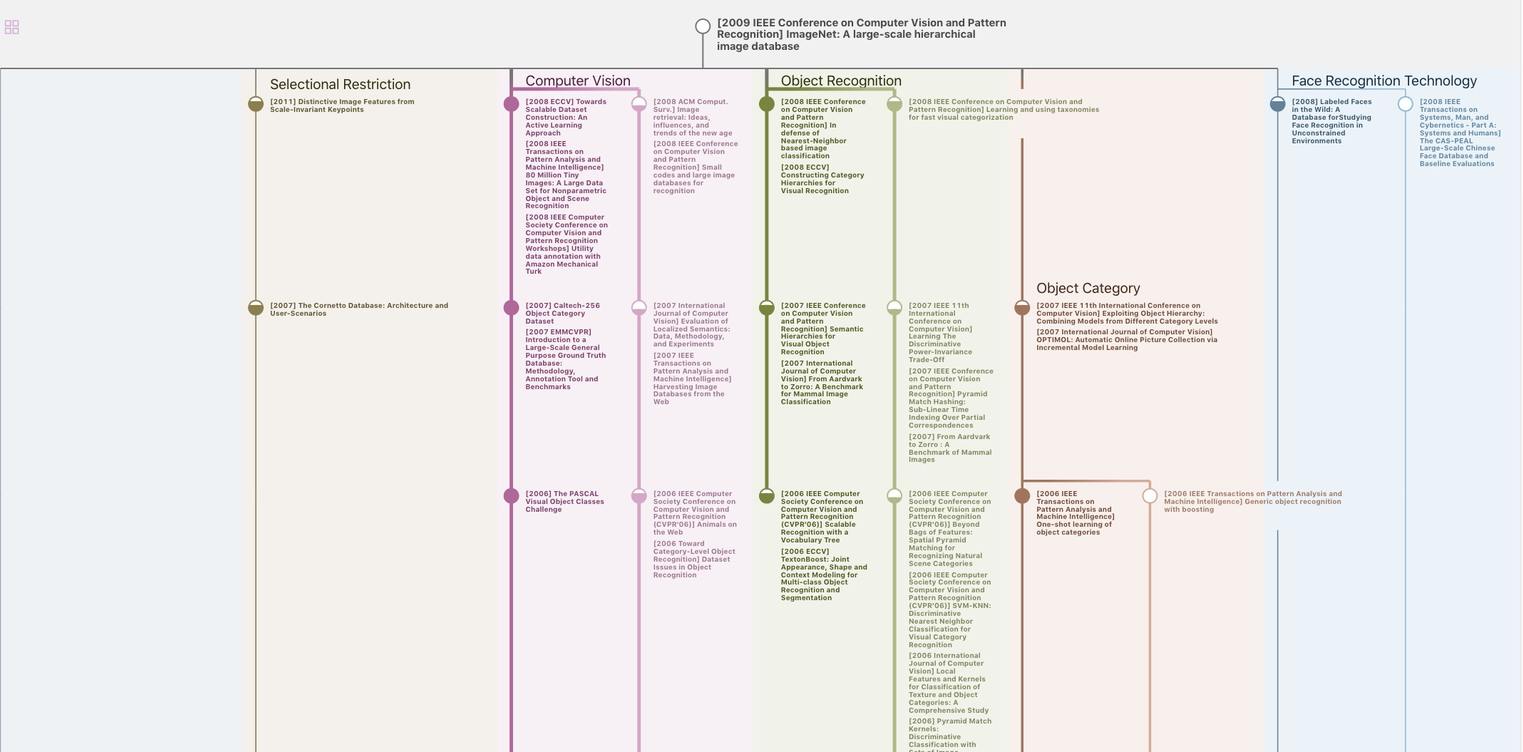
生成溯源树,研究论文发展脉络
Chat Paper
正在生成论文摘要