FCDT-IWBOA-LSSVR: An innovative hybrid machine learning approach for efficient prediction of short-to-mid-term photovoltaic generation
Journal of Cleaner Production(2023)
摘要
The short-term photovoltaic (PV) power prediction is mainly used to prevent photovoltaic power generation fluctuations, grid overvoltage, reverse current, and islanding effects. At the same time, the mid-term PV power forecast is of great significance to improving the availability of PV power generation and the benefit of PV grid connection. Because of the significant differences in time scales between mid-term and short-term power prediction, the short-term power prediction model or method cannot be directly applied to mid-term power prediction. Therefore, few scholars have developed effective short-to-mid-term power forecasting strategies for photovoltaics. As a result, this research proposes a prediction model based on a fast cull outlier algorithm (FC), a decision tree (DT), least squares support vector regression (LSSVR), and an improved whale bat optimization algorithm (IWBOA), which is used for the prediction of the short-to-mid-term photovoltaic power on different time scales, one day and one month in advance. The FC algorithm is used to analyze the difference between the overall data and the abnormal data in the local density and distance to remove the outliers in the original data set. The revised DT model differentiates the data according to climate characteristics to obtain different input data subsets with the best correlation and constructs an independent LSSVR-based model corresponding to the data subsets. The WOA algorithm is improved by independently designing the Chebyshev chaotic initialization strategy, chaotic adaptive inertia weight and BOA algorithm, and the kernel function width and penalty factor of each short-to-mid-term independent sub-PV power prediction model are optimized by IWBOA, thereby improving the computational speed and prediction accuracy of the LSSVR model. Finally, the effectiveness of the proposed strategy is evaluated using actual data from photovoltaic power plants in China. The regression coefficient (R2), mean square error (MSE), root mean square error (RMSE), and mean absolute error (MAE) of short-term forecasting ability of the FCDT-IWBOA-LSSVR model established in this paper are 0.99657, 0.22009, 0.32515, and 0.20959, respectively, while the R2, MSE, RMSE, and MAE of medium-term forecasting ability are 0.98374, 1.91371, 1.38336, and 0.6256. The calculation results show that the prediction accuracy of the FCDT-IWBOA-LSSVR model is higher than that of the WOA-LSSVR, PSO-LSSVR, PSO-BP, I-ACO-SVR, ANN, EMS-DSM-RT, 5 ML, and ELM models.
更多查看译文
关键词
Photovoltaic short-to-mid-term power generation forecasting,The fast cull outlier algorithm,Decision tree,Least square support vector regression,Improved whale bat optimization algorithm
AI 理解论文
溯源树
样例
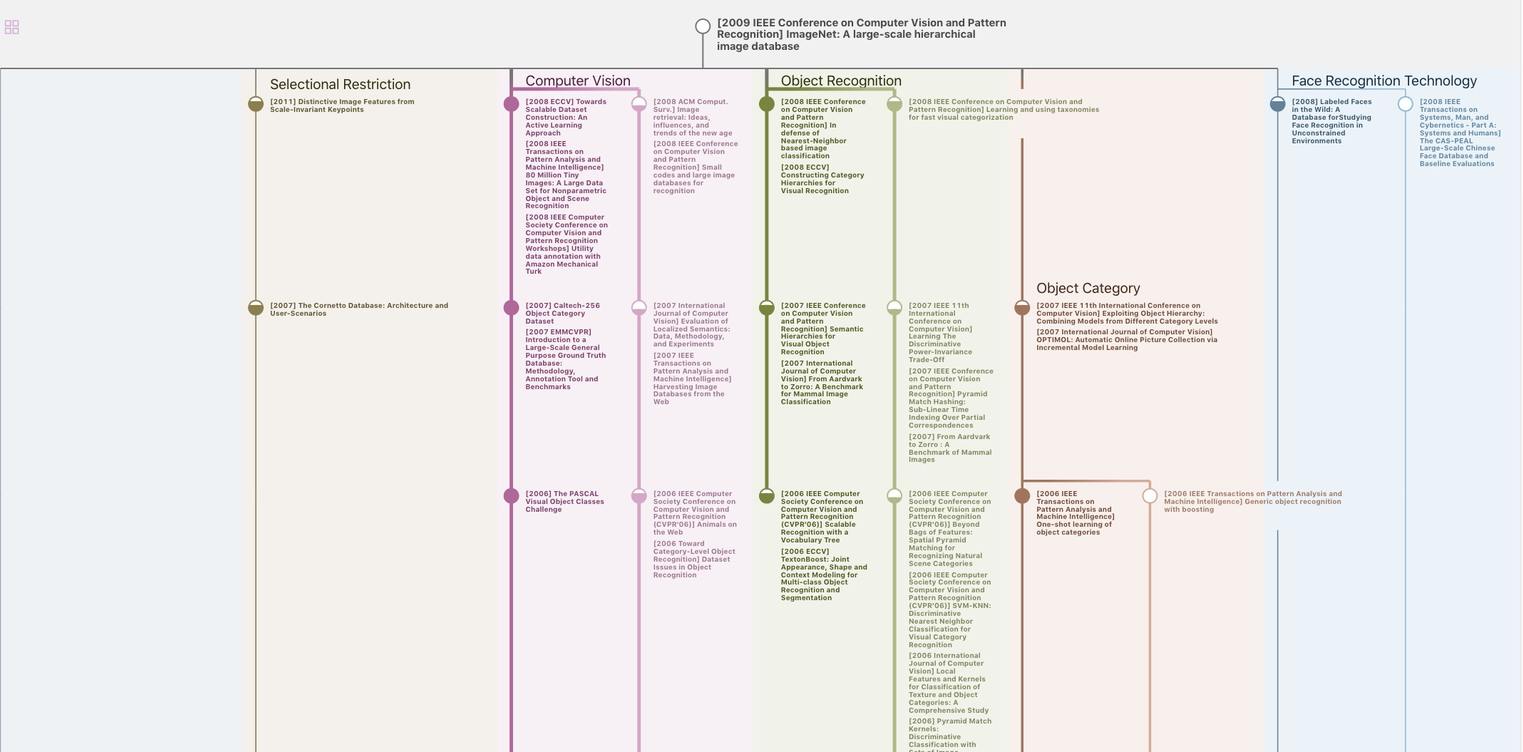
生成溯源树,研究论文发展脉络
Chat Paper
正在生成论文摘要