Integrating Unsupervised Language Model with Triplet Neural Networks for Protein Gene Ontology Prediction.
PLoS Computational Biology(2022)
AI Read Science
Must-Reading Tree
Example
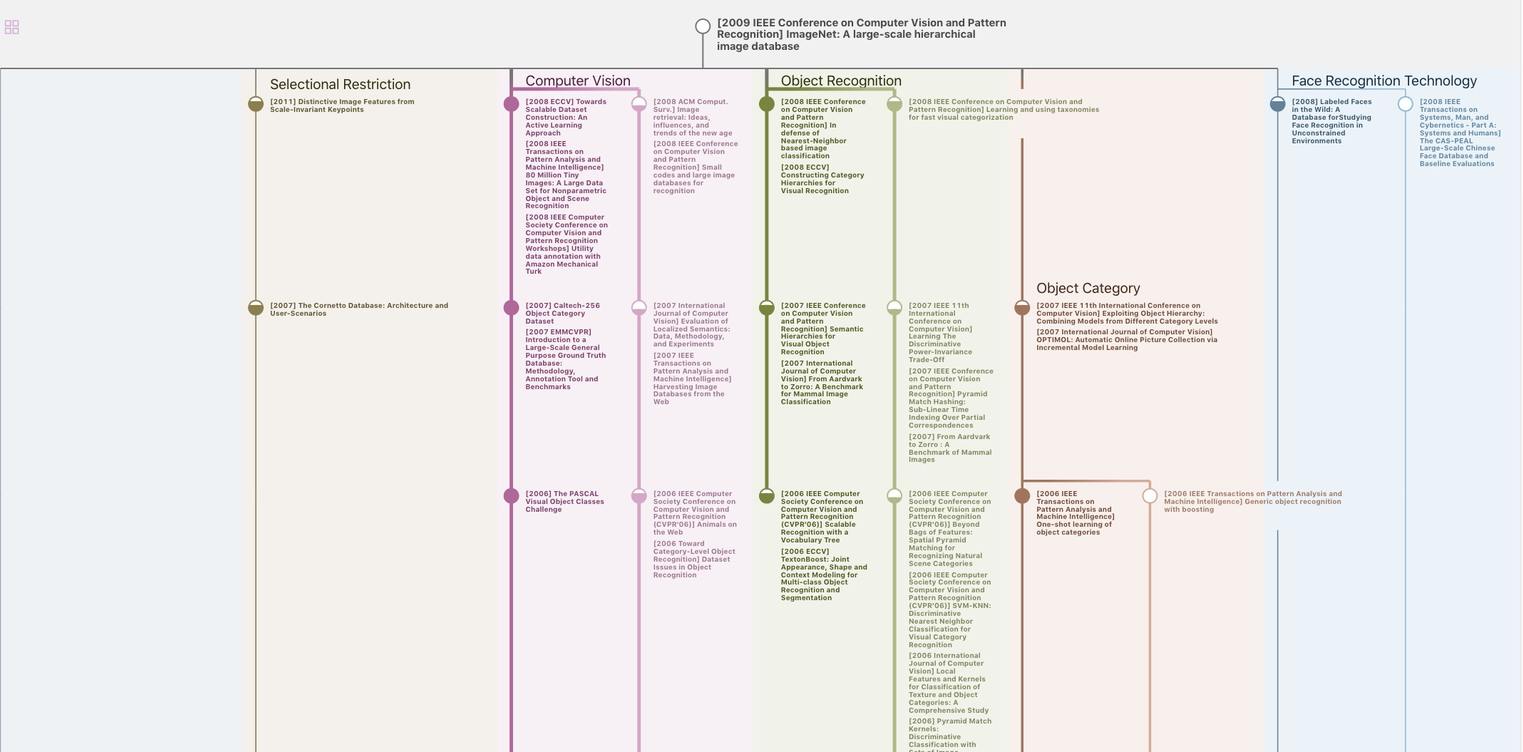
Generate MRT to find the research sequence of this paper
Chat Paper
Summary is being generated by the instructions you defined