A pseudo principal component analysis method for multi-dimensional open-high-low-close data in candlestick chart
COMMUNICATIONS IN STATISTICS-THEORY AND METHODS(2024)
摘要
As the most widely-used data form in the field of finance, the open-high-low-close (OHLC) data is being collected by all kinds of financial trading systems all the time. This paper puts forward a pseudo-principal component analysis (PCA) for multi-dimensional OHLC data, which can extract their useful information in a comprehensible way for visualization and easy interpretation. Firstly, a novel feature-based representation for OHLC data is proposed, which contains fruitful and explicit economic implications. Next, we define a full set of numerical characteristics and variance-covariance structures for the feature-based OHLC data. Then, the pseudo-PCA procedure for OHLC data is deduced based on the proposed algebraic operators. Finally, the effectiveness and interpretability of the proposed pseudo-PCA method are verified through finite simulations and three typical empirical experiments. This paper enriches the application scenarios of classical PCA and contributes to the multivariate statistical modeling of symbolic data. The proposed applications can serve as models for related studies.
更多查看译文
关键词
OHLC data,pseudo-PCA,dimension reduction,feature information extraction
AI 理解论文
溯源树
样例
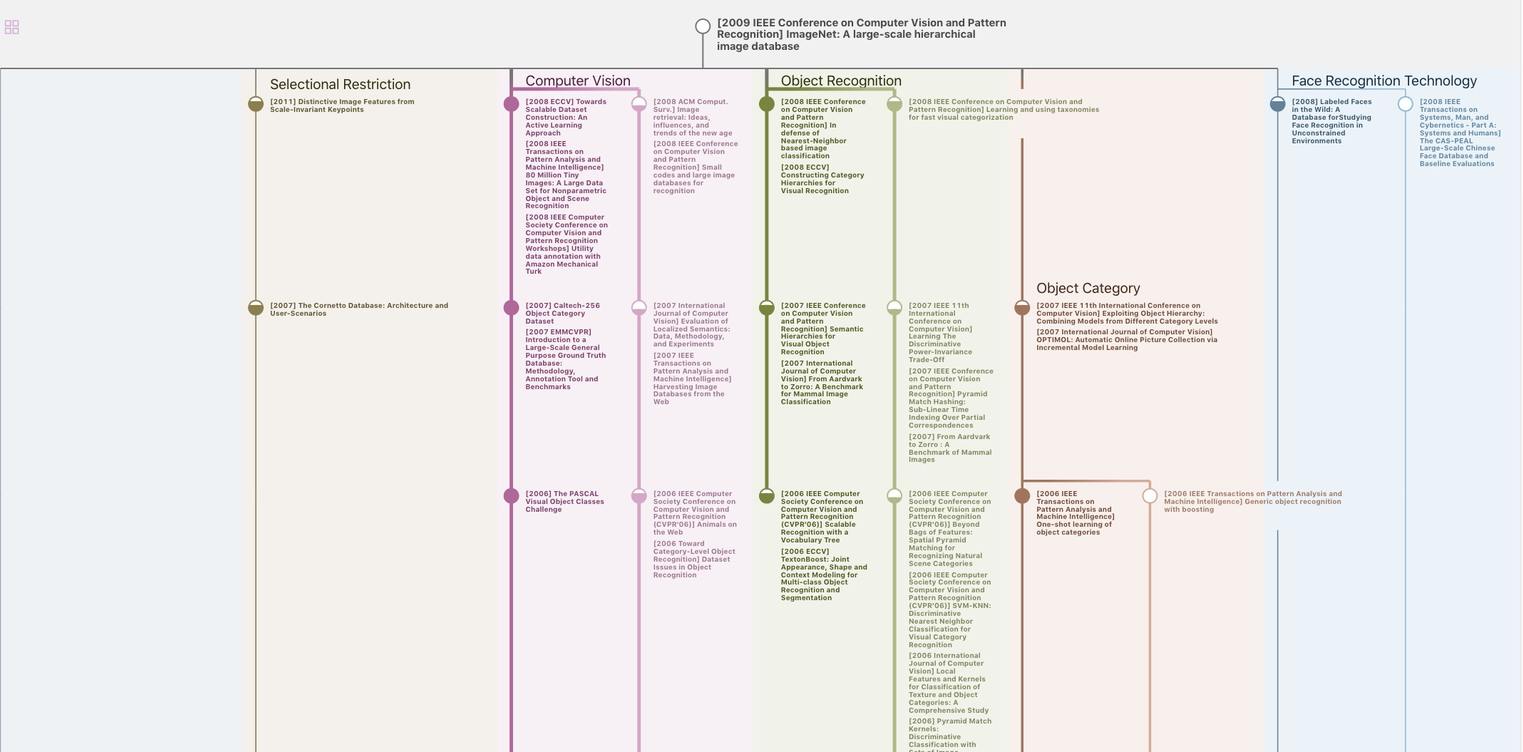
生成溯源树,研究论文发展脉络
Chat Paper
正在生成论文摘要