A high-level programming language for generative protein design
biorxiv(2022)
Key words
generative protein design,programming,high-level
AI Read Science
Must-Reading Tree
Example
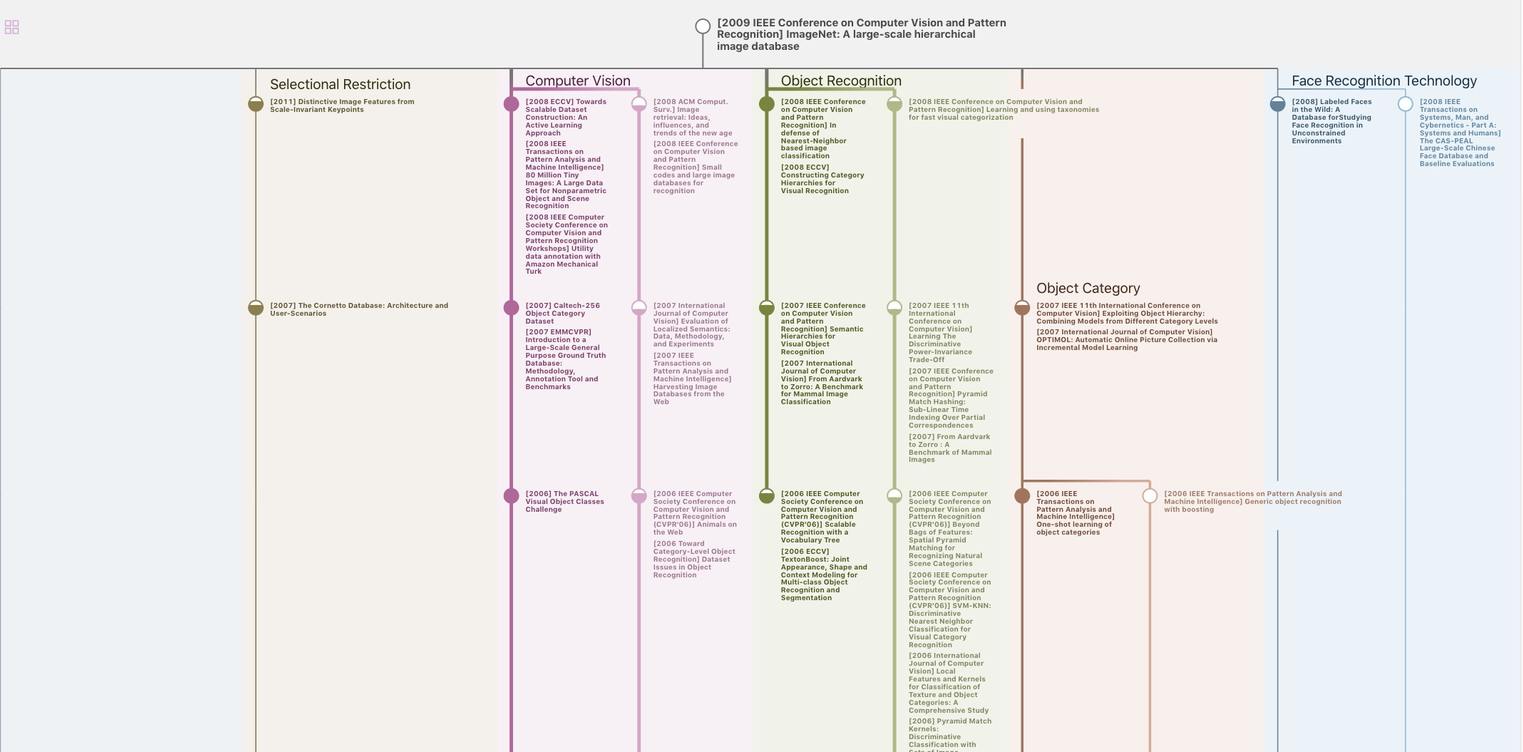
Generate MRT to find the research sequence of this paper
Chat Paper
Summary is being generated by the instructions you defined