Imitation Is Not Enough: Robustifying Imitation with Reinforcement Learning for Challenging Driving Scenarios
2023 IEEE/RSJ INTERNATIONAL CONFERENCE ON INTELLIGENT ROBOTS AND SYSTEMS (IROS)(2023)
Abstract
Imitation learning (IL) is a simple and powerful way to use high-quality human driving data, which can be collected at scale, to produce human-like behavior. However, policies based on imitation learning alone often fail to sufficiently account for safety and reliability concerns. In this paper, we show how imitation learning combined with reinforcement learning using simple rewards can substantially improve the safety and reliability of driving policies over those learned from imitation alone. In particular, we train a policy on over 100k miles of urban driving data, and measure its effectiveness in test scenarios grouped by different levels of collision likelihood. Our analysis shows that while imitation can perform well in low-difficulty scenarios that are well-covered by the demonstration data, our proposed approach significantly improves robustness on the most challenging scenarios (over 38% reduction in failures). To our knowledge, this is the first application of a combined imitation and reinforcement learning approach in autonomous driving that utilizes large amounts of real-world human driving data.
MoreTranslated text
Key words
Urban Driving,Driver Behavior,Lane Detection,Crash Prediction Models,Simulation Models
AI Read Science
Must-Reading Tree
Example
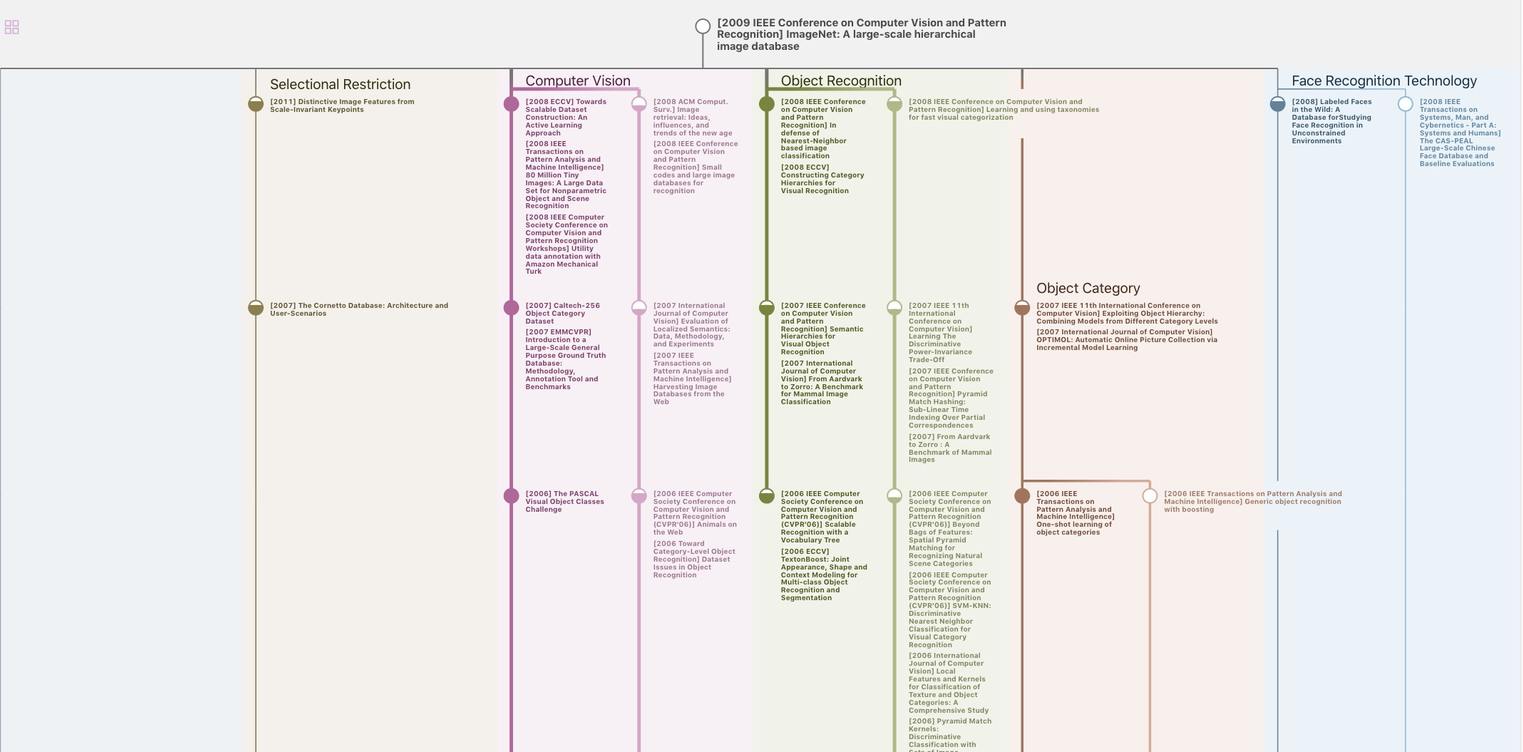
Generate MRT to find the research sequence of this paper
Chat Paper
Summary is being generated by the instructions you defined