Contribution of Timbre and Shimmer Features to Deepfake Speech Detection
2022 Asia-Pacific Signal and Information Processing Association Annual Summit and Conference (APSIPA ASC)(2022)
Key words
acoustic features,ADD 2022,Audio Deep Synthesis Detection Challenge,auditory perception,deep learning,deepfake speech detection,deepfake speech signals,feature classification,feature extractions,multilayer perceptron neural networks,natural voice generation,shimmer feature,synthetic voice generation,timbre feature
AI Read Science
Must-Reading Tree
Example
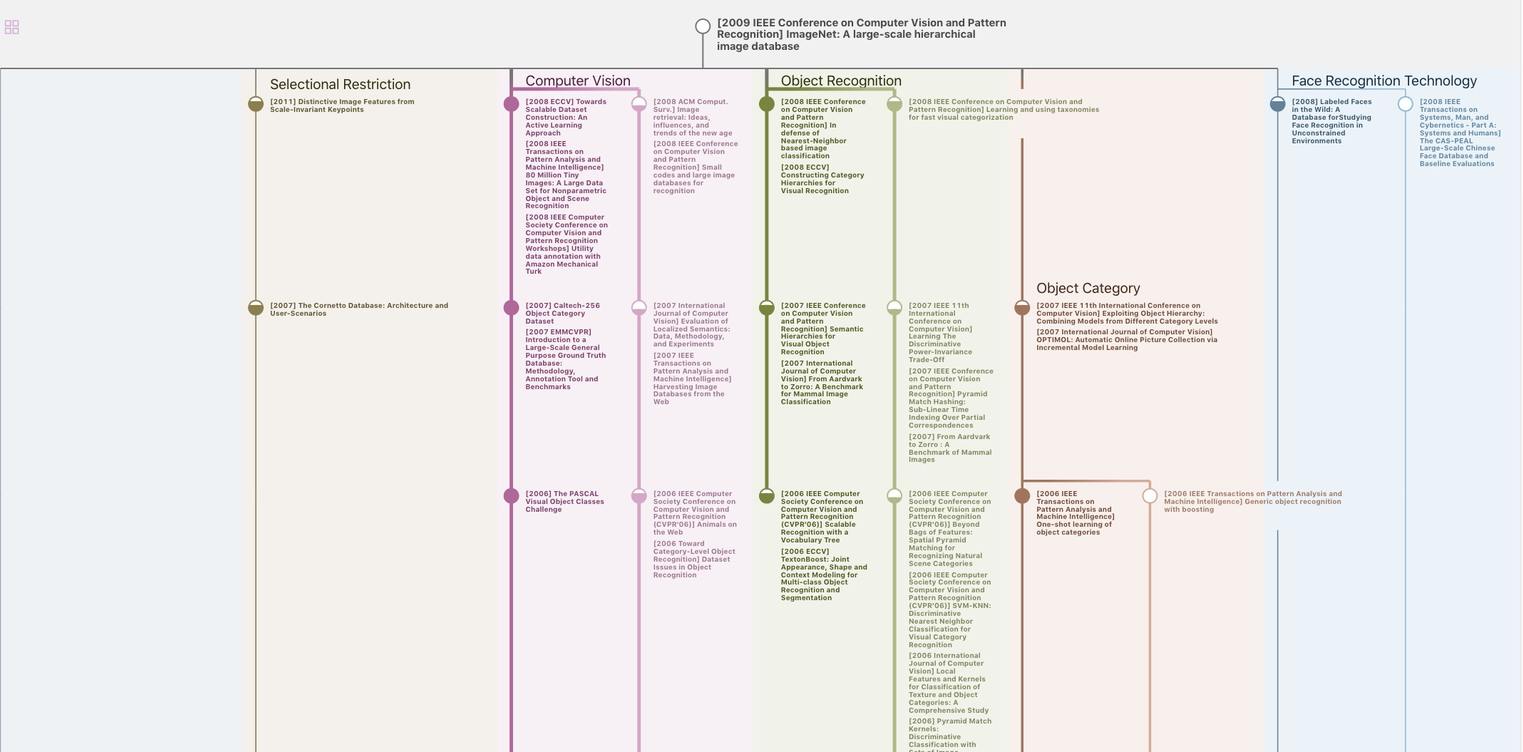
Generate MRT to find the research sequence of this paper
Chat Paper
Summary is being generated by the instructions you defined