Effective ASR Error Correction Leveraging Phonetic, Semantic Information and N-best hypotheses
2022 Asia-Pacific Signal and Information Processing Association Annual Summit and Conference (APSIPA ASC)(2022)
摘要
Automatic speech recognition (ASR) has recently achieved remarkable success and reached human parity, thanks to the synergistic breakthroughs in neural model architectures and training algorithms. However, the performance of ASR in many real-world use cases is still far from perfect. There has been a surge of research interest in designing and developing feasible post-processing modules to improve recognition performance by refining ASR output sentences, which fall roughly into two categories. The first category of methods is ASR N-best hypothesis reranking. ASR N-best hypothesis reranking aims to find the oracle hypothesis with the lowest word error rate from a given N-best hypothesis list. The other category of methods take inspiration from, for example, Chinese spelling correction (CSC) or English spelling correction (ESC), seeking to detect and correct text-level errors of ASR output sentences. In this paper, we attempt to integrate the above two methods into the ASR error correction (AEC) module and explore the impact of different kinds of features on AEC. Empirical experiments on the widely-used AISHELL-l dataset show that our proposed method can significantly reduce the word error rate (WER) of the baseline ASR transcripts in relation to some top-of-line AEC methods, thereby demonstrating its effectiveness and practical feasibility.
更多查看译文
关键词
AISHELL-l dataset,ASR error correction,ASR N-best hypothesis reranking,automatic speech recognition error correction,neural model architectures,oracle hypothesis,phonetic,semantic information,text-level error correction,text-level error detection,training algorithms,word error rate
AI 理解论文
溯源树
样例
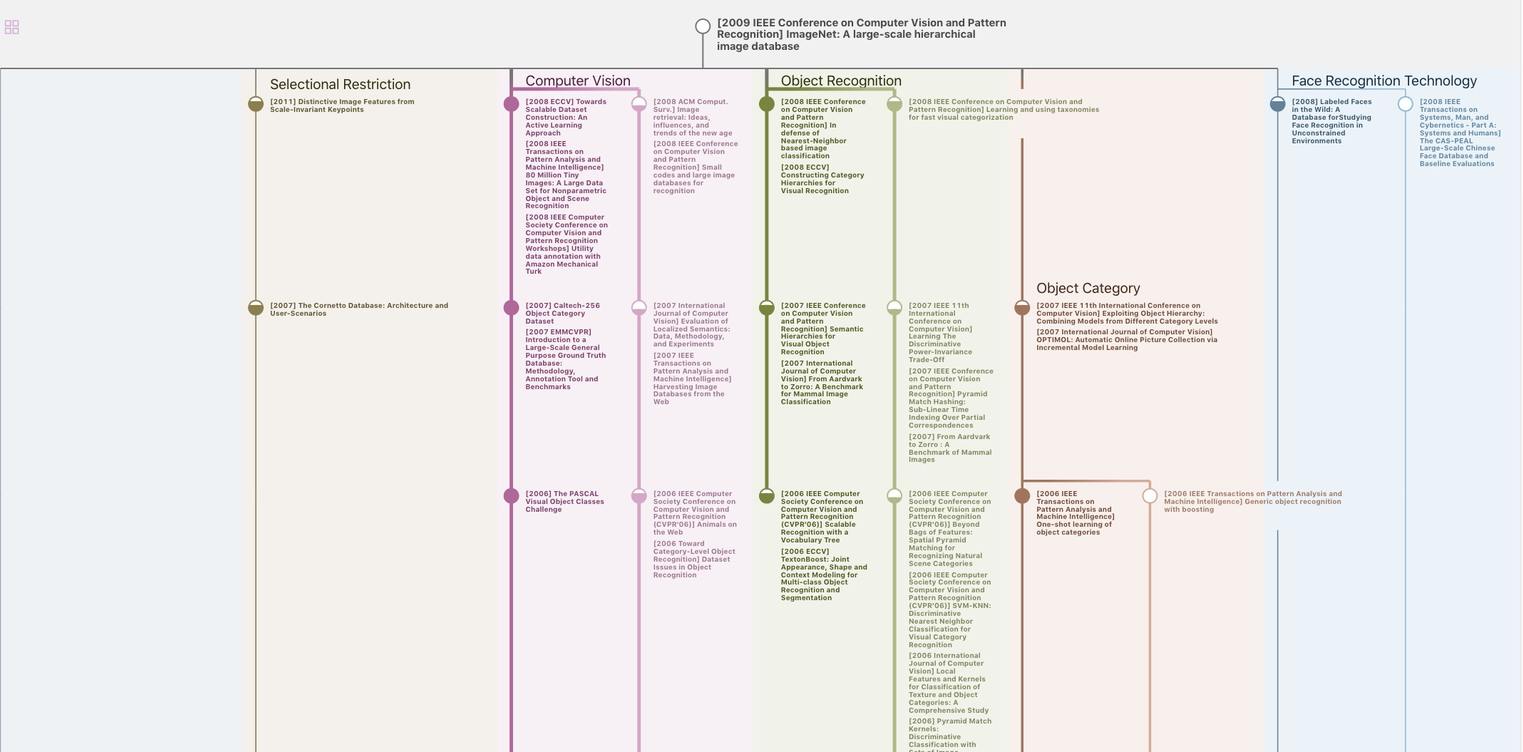
生成溯源树,研究论文发展脉络
Chat Paper
正在生成论文摘要