Efficient Frameworks for Generalized Low-Rank Matrix Bandit Problems
NeurIPS(2024)
摘要
In the stochastic contextual low-rank matrix bandit problem, the expected
reward of an action is given by the inner product between the action's feature
matrix and some fixed, but initially unknown d_1 by d_2 matrix Θ^*
with rank r ≪{d_1, d_2}, and an agent sequentially takes actions based
on past experience to maximize the cumulative reward. In this paper, we study
the generalized low-rank matrix bandit problem, which has been recently
proposed in under the Generalized Linear Model (GLM)
framework. To overcome the computational infeasibility and theoretical restrain
of existing algorithms on this problem, we first propose the G-ESTT framework
that modifies the idea from by using Stein's method on
the subspace estimation and then leverage the estimated subspaces via a
regularization idea. Furthermore, we remarkably improve the efficiency of
G-ESTT by using a novel exclusion idea on the estimated subspace instead, and
propose the G-ESTS framework. We also show that G-ESTT can achieve the
Õ(√((d_1+d_2)MrT)) bound of regret while G-ESTS can achineve the
Õ(√((d_1+d_2)^3/2Mr^3/2T)) bound of regret under mild
assumption up to logarithm terms, where M is some problem dependent value.
Under a reasonable assumption that M = O((d_1+d_2)^2) in our problem setting,
the regret of G-ESTT is consistent with the current best regret of
Õ((d_1+d_2)^3/2√(rT)/D_rr) (D_rr will
be defined later). For completeness, we conduct experiments to illustrate that
our proposed algorithms, especially G-ESTS, are also computationally tractable
and consistently outperform other state-of-the-art (generalized) linear matrix
bandit methods based on a suite of simulations.
更多查看译文
AI 理解论文
溯源树
样例
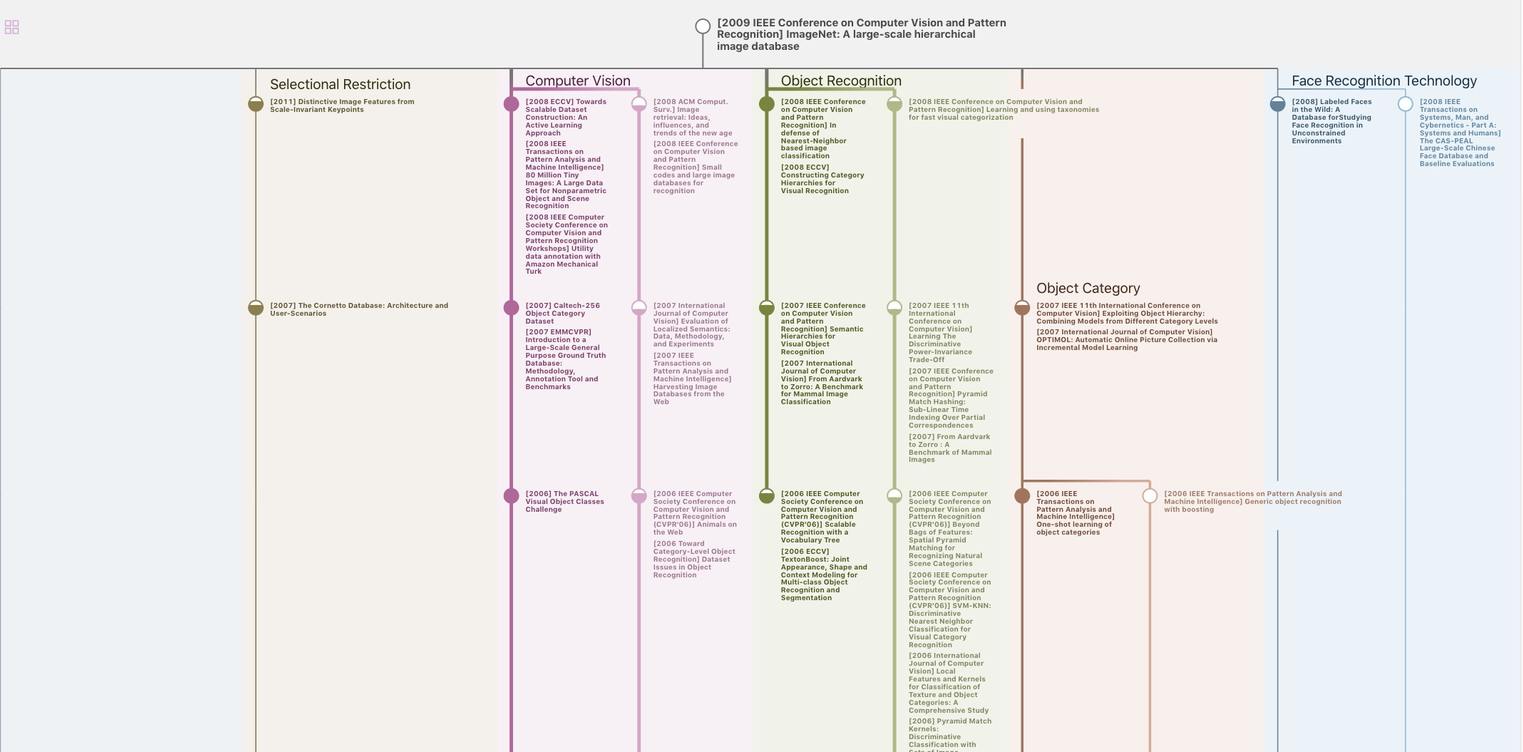
生成溯源树,研究论文发展脉络
Chat Paper
正在生成论文摘要